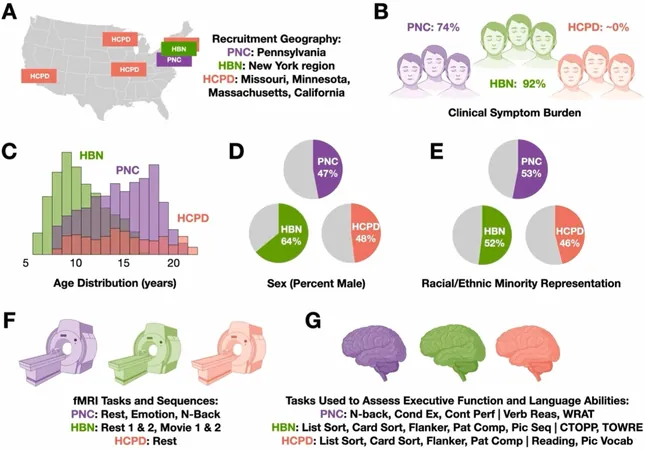
Unlocking the Future of Mental Health: How Diverse Datasets Are Revolutionizing Machine Learning in Neuroimaging
2024-11-04
Author: Wei Ling
In the ever-evolving field of neuroimaging, one of the most ambitious goals remains: connecting brain activity to behavior. This groundbreaking research may not only illuminate the workings of the human brain but also pave the way for personalized treatments for mental health disorders and neurological conditions.
Traditionally, scientists have leveraged brain images along with behavioral data to train machine learning models capable of predicting individual symptoms or illnesses based on brain function. However, these models have a critical flaw—they must exhibit versatility across various settings and populations to be truly impactful.
A recent study from Yale University showcases a promising breakthrough. The researchers assert that their predictive models can successfully operate on datasets that differ significantly from the training sets. They believe this adaptability is crucial for the creation of clinically relevant predictive models.
"Typically, predictive models show strong performance when tested on datasets similar to the ones on which they were trained," states Brendan Adkinson, the lead author of the study published in the journal *Developmental Cognitive Neuroscience*. "However, when applied to varied datasets, their performance often diminishes, rendering them ineffective for real-world scenarios."
This performance discrepancy often stems from differences among datasets, which include diverse factors such as age, sex, race and ethnicity, geographical location, and the presentation of clinical symptoms. Adkinson suggests that instead of viewing these variations as obstacles, researchers should embrace them as essential components of model development.
For a practical demonstration, the Yale team evaluated their models' efficacy by predicting two key cognitive traits: language abilities and executive function. They trained separate models on three substantially different datasets, and then tested each model on the other datasets. The results? Remarkably, the performance remained impressive, suggesting that generalizable models are indeed within reach.
The implications of this research extend beyond just the models themselves. Adkinson expresses a keen interest in exploring generalizability concerning specific populations. He notes that current large-scale data collection efforts are often concentrated in metropolitan centers, where researchers can access larger groups of people. This could lead to a gap in model applicability, particularly for individuals in rural settings.
As the field of neuroimaging continues to grow, the commitment to utilizing diverse datasets may signal a new era in personalized mental health treatment. With ongoing advancements and a focus on inclusivity, the dream of effective, individualized care could soon become a reality.