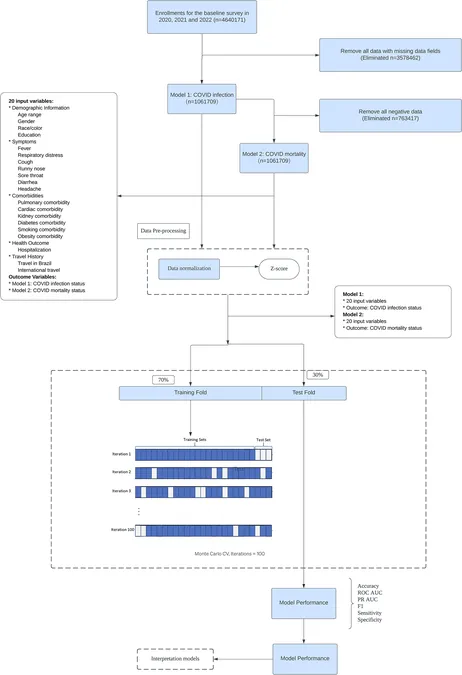
The Surprising Insights on COVID-19 Predictions: How Timing Affects Outcomes
2025-04-24
Author: Siti
The End of an Era: Reflecting on COVID-19
The World Health Organization (WHO) has officially declared an end to the COVID-19 public health emergency, but the aftermath leaves us with deep, lasting scars. To date, over 773 million cases have been reported, and millions have tragically lost their lives.
Variants: The Ever-Changing Face of COVID-19
Since the outbreak began, several variants of SARS-CoV-2 have emerged, each exhibiting unique characteristics that drastically influenced infection rates and mortality. The Alpha variant originated in the UK, Delta swept in from India, and Omicron made waves after being identified in South Africa, causing widespread concern due to its rapid transmission and mutation.
Revolutionizing Predictions: The Role of Data
In this digital age, COVID-19 has radically transformed public health practices through the use of advanced analytics. Predictive models leveraging machine learning have emerged, but their robustness and accuracy have been called into question. Models are often trained on datasets that may not capture the nuances of each variant's effects, potentially skewing results.
The Play of Time: How Temporality Complicates Accuracy
A growing body of research indicates that machine learning models struggle with accuracy when predictions are made across different time periods. This inconsistency suggests a need for deeper examination of how various factors—especially the dominant variant during a specific timeframe—impact predictive performance for infection and mortality.
Investigating Feature Contributions: Shapley Values to the Rescue
To get to the bottom of these discrepancies, a fresh approach using Shapley values will be used to assess the importance of individual features in predictive models. This methodology provides clarity on how different factors contribute to infection and mortality predictions, helping identify the specific traits that lead to variation in model performance over time.
A Trove of Data: The Brazilian Perspective
Leveraging a comprehensive database from Brazil, encompassing records from 2019 to 2022, this study investigates over a million individuals. This extensive dataset allows for a detailed analysis of the impact of temporal factors on predictive model performance.
Key Findings: A Tale of Two Predictions
The exploration highlights a stark contrast between predicting infection and mortality rates. While COVID-19 infection predictions faced substantial accuracy challenges depending on the year of data used, mortality predictions proved to be remarkably stable, showing less variability regardless of temporal factors.
The Dominant Features: Unraveling the Core Predictors
Importantly, the study reveals that certain consistent features—like age and hospitalization status—dominate mortality predictions across all three years. This stability underlines the importance of these variables in understanding COVID-19 outcomes.
Conclusion: A Call for Robust Models
The findings emphasize the critical need for machine learning practitioners to consider temporal factors in their models. The study advocates for regular updates and assessments using Shapley values to ensure predictive models remain relevant and accurate as the pandemic evolves.
Implications for Future Public Health Strategies
As we navigate the complexities of public health in a post-pandemic world, these insights could help shape stronger and more reliable predictive models, ultimately guiding effective interventions in future health crises.