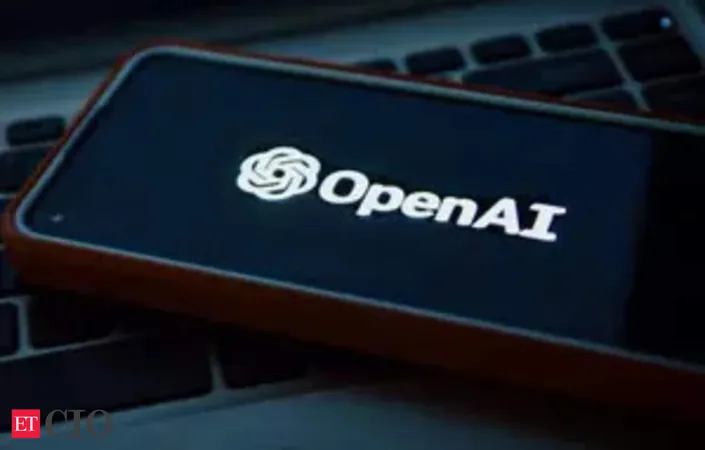
The New AI Frontier: OpenAI and Rivals Shift from Scalability to Smarter Thinking Techniques
2024-11-11
Author: Wei
The AI Landscape Evolution
In a pivotal shift within the artificial intelligence sector, leading companies like OpenAI are re-evaluating the direction of their developments as traditional methods of scaling large language models encounter critical limitations. As of November 11, 2024, AI researchers, investors, and industry insiders are revealing that a new focus on human-like reasoning techniques may redefine the landscape of AI technology.
Concerns Over Scaling
Despite the prior mantra that “bigger is better” for model improvement, many experts, including AI co-founder Ilya Sutskever, are voicing concerns about the efficacy of simply adding more data and computational power. Following the significant success of ChatGPT two years ago, the technology industry has increasingly relied on “scaling up” as a strategy for enhanced performance. However, Sutskever, who left OpenAI this year to start his own lab, Safe Superintelligence (SSI), suggests that this approach has reached a pivotal plateau.
A New Era of Discovery
“The 2010s were the age of scaling,” Sutskever stated. “Now, we are returning to an era of wonder and discovery, where the focus needs to be on the right kind of scaling.” His team is currently investigating alternative methods to improve pre-training, the foundational phase in AI model development.
Challenges in Advancing AI Models
As researchers race to create a new breed of large language models to surpass OpenAI's nearly two-year-old GPT-4, they have encountered significant hurdles. Training these intricate models can be a costly endeavor—often amounting to tens of millions of dollars. Compounding this is the harsh reality that the existing models consume vast quantities of data, leading to shortages as easily accessible datasets become depleted.
Innovations in Decision-Making
In response to these challenges, new methodologies like “test-time compute” are taking center stage. This innovative technique allows AI models to evaluate multiple answers during the “inference” phase, thereby enhancing their decision-making capabilities in real-time. Notably, OpenAI's latest model, o1, leverages this approach, bringing it closer to a more human-like capacity for complex problems.
Competition and Market Dynamics
This advancement has spurred competition among major players, including Anthropic, xAI, and Google DeepMind, all racing to refine their versions of these new techniques. As the AI landscape evolves, venture capitalists are closely monitoring shifts in demand for AI hardware, thus altering business dynamics previously dominated by companies like Nvidia, whose chips have become the gold standard.
The Future of AI Development
Sonya Huang from Sequoia Capital emphasized the potential transformation in the marketplace: “We are moving toward a scenario where distributed, cloud-based servers for inference become more critical than massive pre-training clusters.”
Implications for AI Hardware
The implications of this shift extend beyond development strategies and into the competitive realm of AI hardware. Nvidia, currently the world's most valuable company following a significant surge driven by AI chip demand, may face heightened competition in the inference market. This transition could ultimately lead to a broader spectrum of chips catering to diverse aspects of AI processing.
Conclusion
As the AI revolution marches forward, the lessons learned from initial limitations may pave the way for smarter, more efficient intelligence systems that better emulate human reasoning and creativity. The future of AI is not just about scaling; it's about redefining how these technologies think.