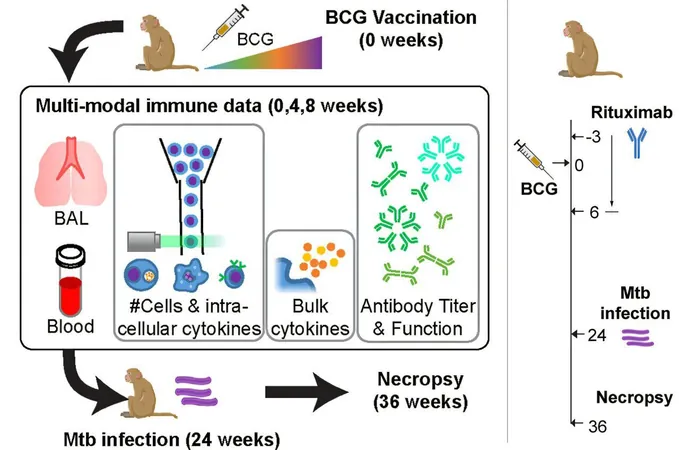
Revolutionizing Vaccine Development: New Computational Models Uncover Immune System Mysteries
2024-11-07
Author: Yu
Introduction
In an era where biological data is surging, recent advancements in technology have enabled scientists to gather an overwhelming volume of biological information. Through expansive studies in genomics, transcriptomics, proteomics, and cell profiling, researchers have amassed prodigious datasets from both cellular and multicellular systems. Yet, the challenge lies in deciphering this complex information, particularly when it comes to intricate biological interactions, such as those governing immune responses to pathogens.
Innovative Computational Methodology
Researchers at MIT have risen to this challenge with an innovative computational method that extracts valuable insights from biological datasets. Their cutting-edge technique has successfully elucidated the interactions that define the immune system's response to tuberculosis (TB) vaccines, revealing pathways that could significantly enhance vaccine efficacy.
Insights from MIT's Douglas Lauffenburger
Douglas Lauffenburger, the Ford Professor of Engineering at MIT, highlights the revolutionary potential of their computational modeling framework. "We’ve developed a model that not only predicts the outcomes of various interventions in complex biological systems but does so while embracing the multi-faceted nature of biological components," he explained.
Multifaceted Nature of Biological Data
The study, co-authored by Shu Wang and Amy Myers, has been recently published in *Cell Systems*. Their research focuses on the multifaceted nature of biological data — from genomic sequencing that identifies gene variants to messenger RNA analysis revealing gene expression, as well as proteomics that measure protein levels and cytometry which counts various cell types.
Limitations of Traditional Predictive Modeling
Traditional predictive modeling approaches, often reliant on AI and machine learning, have their limitations. They can forecast whether a vaccine will produce a strong immune response but fall short in elucidating the biological mechanisms at play. Lauffenburger notes, "While AI can serve clinical needs, it lacks the granularity required for comprehensive biological understanding."
Probabilistic Graphical Networks
To bridge this gap, the researchers employed probabilistic graphical networks—a modeling technique more frequently used in fields such as speech recognition and computer vision, but underutilized in biological research. Their innovative adaptation involves filtering out noise to identify direct relationships between biological components. Lauffenburger remarks, “This allows us to pinpoint the essential interactions underlying complex systems.”
Focus on BCG Vaccine
In their investigation, the researchers focused on the BCG vaccine, an attenuated form of Mycobacterium bovis used to combat tuberculosis, though its efficacy has varied over time. Previous studies explored alternative administration routes for BCG, such as intravenous and inhalational delivery, seeking improved immune responses. The MIT team aimed to clarify the mechanisms driving stronger outcomes associated with intravenous administration.
Data Analysis and Findings
Analyzing approximately 200 variables in their data from animal studies, including cytokine levels and diverse immune cell profiles, the researchers tracked both pre- and post-vaccination responses. Their modeling efforts revealed critical pathways whereby the vaccine mobilizes specific T cells, which in turn activate B cells that generate antibodies against the pathogen.
Implications for Vaccine Development
“This modeling acts like a roadmap, allowing us to identify the key interaction routes that matter most,” said Lauffenburger. Their predictions also proved accurate; when the model suggested that eliminating B cells would minimally affect the overall response, experimental results validated this conclusion.
Future Prospects
The implications of this computational approach extend far beyond tuberculosis. Vaccine developers can leverage these insights to refine their formulations, enhancing their potential before clinical testing. Lauffenburger’s team is already applying this modeling strategy to explore malaria vaccines administered in various African nations and is also investigating how tumors interact with immune cells, aiming to predict treatment responses in cancer therapies.
Conclusion
The potential of this research is staggering. As scientists continue to unlock the mysteries of the immune system, we move closer to vaccines that not only combat existing diseases but adapt more dynamically to emerging health threats. The future of vaccine development has truly arrived, filled with promise and precision.
Stay Informed!
Stay tuned as MIT News continues to cover groundbreaking advancements in research and innovation!