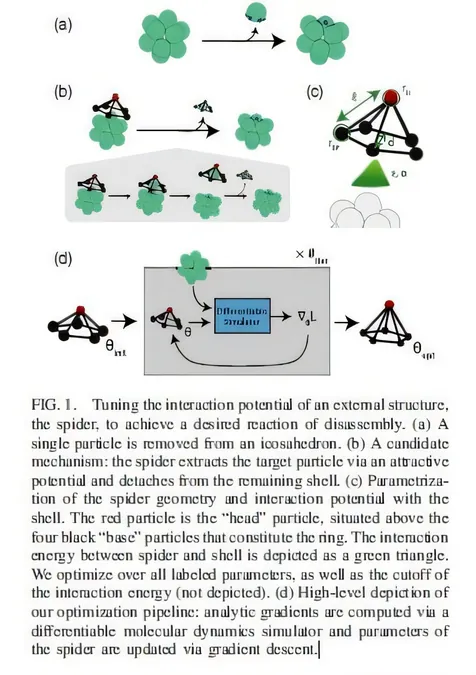
Revolutionizing Medicine: Machine Learning Creates a Molecular Can Opener
2024-12-27
Author: Li
In an age where medical treatments are progressively shifting towards more targeted, personalized, and effective approaches, researchers are enthusiastic about harnessing molecular technology for specific therapeutic applications. These advancements hold promise for areas such as gene therapy and efficient drug delivery systems, which are essential for widespread clinical use.
A groundbreaking study published in Physical Review Letters highlights the efforts of a team led by Harvard's Ryan K. Krueger, who utilized machine learning to design a sophisticated "opener" for molecules trapped within a molecular cage. By employing differentiable molecular dynamics, the researchers were able to devise complex reactions that direct system outcomes with precision.
The research focused particularly on the controlled disassembly of colloidal structures—microscopic mixtures where tiny insoluble particles are dispersed throughout another medium, such as milk or smoke. The innovative design created by the team could selectively detach a particle enveloped by a tightly bound shell of colloidal particles, akin to the protective capsids that encapsulate viruses.
The "opener" molecule, aptly termed the "spider," was developed with an intricate shape that reflects its intended function. The researchers noted that disassembly is crucial to dynamic processes within living systems, including defect repair and self-replication. The team specifically targeted the dismantling of icosahedral shells, composed of 12 particles characterized by their geometrically unique 30 edges, closely resembling viral capsids.
A key aspect of their research was the understanding of "patchy" particles, which interact with other shell particles and the encapsulated molecule through tailored interaction parameters. Introduced two decades ago, the concept of patchiness allows for versatile tuning of particle interactions—essential for achieving desired behaviors based on recent advances in simulation technology.
The disassembly challenge presented a balancing act of exerting enough force to dismantle the shell while preserving the remaining substructure. The researchers modeled the interaction energies using a Morse potential, a well-established framework in physics that simplifies the complexity of interatomic forces.
Their innovative approach employed a pyramid-like structure, the "spider," designed to perch on the cluster of shell particles and interact dynamically. Following extensive simulations, the researchers meticulously adjusted how the spider interacted with the shell particles, considering varying levels of attraction and repulsion.
The use of molecular dynamics offered a more efficient means of examining how different configurations of the spider could extract the caged molecule. Instead of employing a brute force method, which would demand extraordinary computational resources, the team adopted machine learning to optimize their design by minimizing a loss function that balanced disassembly effort and structural integrity.
An exciting discovery was made when they allowed the spider's parameters to adapt flexibly. Upon optimization, the energy required to liberate the caged particle diminished significantly, showcasing the efficiency of incorporating asymmetrical flexibility into the spider's design over its initially symmetrical form.
The implications of this research reach far beyond basic science. The methodologies developed here could facilitate experimental realization of complex theoretical models that have previously struggled due to the limitations of precise energy interaction tuning. As Krueger and his colleagues suggested, their findings open up a realm of possibilities for innovative applications in materials science and drug delivery systems that could lay the groundwork for the next generation of medical therapies.
The fusion of machine learning with molecular dynamics not only enhances the capabilities of scientists but also symbolizes a pivotal shift in how we comprehend and manipulate complex biological systems—a leap forward that could redefine therapeutic strategies in the future.