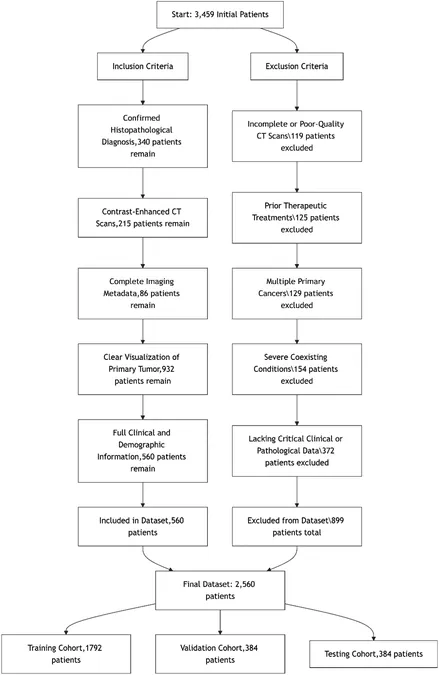
Revolutionizing Esophageal Cancer Diagnosis: A Breakthrough Framework Combines AI and Imaging Techniques
2025-05-10
Author: Siti
A Major Leap in Esophageal Cancer Grading
Esophageal cancer poses a significant hurdle in oncology, marked by high mortality rates and often diagnosed at advanced stages. Accurate grading of this cancer is crucial as it aids in formulating tailored treatment strategies and improving patient survival rates. This groundbreaking study unveils an innovative framework that harnesses machine learning—specifically radiomics and deep learning—to enhance the accuracy and consistency of esophageal cancer grading.
The Importance of Early Grading
Pinpointing cancer grades early on is vital for crafting effective treatment plans. High-grade tumors are typically more aggressive and pose a greater risk of metastasis, which correlates with poorer outcomes. By classifying tumors early, healthcare providers can tailor interventions—be it surgery, chemotherapy, or radiotherapy—tailored to each patient’s needs.
Diverse Tumor Considerations
Esophageal cancer is categorized based on the tumor's anatomical location: the upper, middle, and lower esophagus. This categorization is clinically significant, impacting treatment approaches. For instance, tumors in the upper esophagus might need extensive surgical actions, while those in the lower may require management at the gastroesophageal junction.
Harnessing the Power of Radiomics and Deep Learning
Radiomics is pivotal in extracting vital quantitative information from CT scans, reflecting crucial tumor features such as shape and texture directly linked to patient outcomes. Tackling the inconsistencies in ROI segmentation, this study employs a multi-segmentation strategy for improved reliability.
Deep Learning: The Game Changer
Utilizing advanced convolutional neural networks (CNNs), this framework automates feature extraction and cultivates end-to-end learning. Models like DenseNet121 and EfficientNet-B0 are leveraged to capture complex imaging data patterns, further enhanced by attention mechanisms that spotlight key diagnostic areas in tumors, refining both accuracy and interpretability.
A Dual Approach to Feature Extraction
This novel framework melds radiomic features with deep learning insights. Radiomic metrics offer reliable, interpretable parameters while deep features encapsulate intricate tumor characteristics. By integrating both, the framework establishes a more robust diagnostic tool.
Validation on a Large Scale: Ensuring Reliability
This study is anchored by an extensive dataset from 2,560 patients, rigorously collected over several years. Following strict inclusion and exclusion criteria, the results validate the framework’s efficacy across diverse patient demographics, further enhancing clinical applicability.
Innovation in Imaging: Segmentation and Feature Extraction
Adopting a multi-segmentation approach significantly reduces bias and enhances reproducibility in feature extraction. This ensures that the primary tumor regions delineated for analysis are consistent and reliable—essential for nuanced image evaluation.
Enhanced Diagnostic Performance
In testing, models embedding the best features culminated in outstanding diagnostic accuracy, with XGBoost achieving an AUC of 96.70% when combining radiomic and deep learning features. This underscores the framework's potential to substantially outperform traditional methods.
Conclusion: A New Era in Cancer Diagnostics
This pioneering integration of radiomics and deep learning models represents a significant advancement in esophageal cancer grading. By focusing on reproducibility, interpretability, and robust clinical validation, this framework not only enhances diagnostic precision but also lays the groundwork for future research into more effective cancer treatment pathways.
Looking Ahead: The Future of Cancer Grading
As the field progresses, further exploration into diverse imaging modalities and the implementation of real-time clinical decision support systems could further amplify the impact of this innovative approach, ultimately enhancing outcomes for patients battling esophageal cancer.