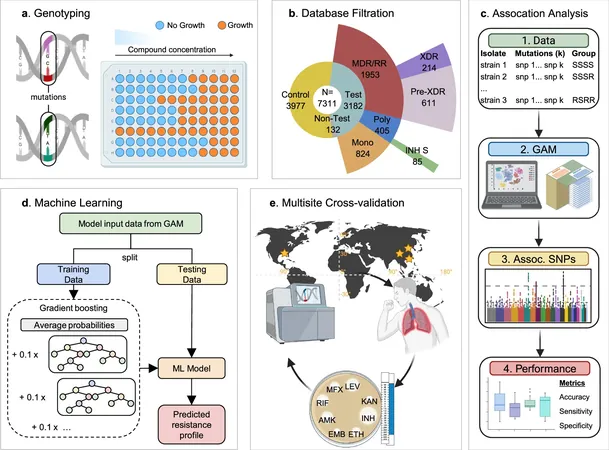
Revolutionary AI Method Set to Change the Game in Antibiotic Resistance Prediction!
2025-04-07
Author: Li
In an alarming twist, drug-resistant infections are becoming a formidable challenge to global health. Pathogens such as tuberculosis and staphylococcus are at the forefront, making treatment options complex and costly. According to the World Health Organization, in 2021, over 450,000 individuals fell victim to multidrug-resistant tuberculosis, and the grim success rate for treatments plummeted to just 57%. This crisis demands innovative solutions, and researchers at Tulane University have stepped up with groundbreaking advancements.
Introducing a Game-Changing Model
Introducing an advanced artificial intelligence-based method that surpasses traditional standards, Tulane scientists have unveiled a new Group Association Model (GAM), designed to detect genetic markers of antibiotic resistance in Mycobacterium tuberculosis and Staphylococcus aureus with remarkable precision. Unlike conventional approaches, which often draw erroneous links between unrelated mutations and drug resistance, GAM operates without prior assumptions about resistance mechanisms. This flexibility allows the model to reveal novel genetic alterations that could escape standard detection.
Addressing Current Limitations
Current testing protocols employed by organizations like the WHO can be cumbersome and occasionally miss subtle mutations. Traditional culture-based testing takes valuable time, while some DNA-based tests lack sensitivity for rare variants. Tulane's innovative model addresses these issues head-on by leveraging whole genome sequencing to meticulously compare groups of bacterial strains exhibiting distinct resistance profiles. This helps in pinpointing genetic changes that are reliable indicators of antibiotic resistance.
Expert Insights
Dr. Tony Hu, a leading researcher and director of the Tulane Center for Cellular & Molecular Diagnostics, emphasized, “Imagine using a comprehensive genetic fingerprint of bacteria to identify what shields it from specific antibiotics. We are teaching computers to discern patterns of resistance without human intervention.
Research Findings
In their study, the researchers analyzed over 7,000 strains of Mycobacterium tuberculosis and nearly 4,000 strains of Staphylococcus aureus, successfully identifying critical mutations associated with antibiotic resistance. The findings revealed that GAM not only equaled but often surpassed the accuracy of the WHO’s resistance database, significantly decreasing the likelihood of false positives that could mislead treatment decisions.
Enhancing Patient Outcomes
Julian Saliba, a graduate student involved in the research, added, "Existing genetic tests can mistakenly categorize bacteria as resistant, leading to potentially harmful treatment decisions. Our method clarifies which mutations genuinely lead to resistance, thus enhancing patient outcomes and reducing misdiagnoses."
Improved Predictions with Machine Learning
With the integration of machine learning, the ability to predict resistance even when data is limited or incomplete has remarkably improved. In validation studies using clinical samples from China, Tulane’s GAM model outperformed existing WHO-based methodologies in forecasting resistance to essential front-line antibiotics—a substantial development in the fight against antimicrobial resistance.
The Broader Impact
Early detection of resistance is crucial, as it empowers healthcare providers to customize treatment plans effectively before infections escalate. Moreover, the potential applications of this breakthrough model extend beyond human health; it may also play a pivotal role in agriculture, tackling antibiotic resistance challenges in crops where such issues are becoming increasingly prevalent.
Conclusion
As the world grapples with an escalating antibiotic crisis, the emergence of Tulane University’s innovative AI method heralds hope for timely, effective treatment strategies against deadly bacterial infections. Will this technological leap steer us toward a future where antibiotic resistance can be managed more effectively? Only time will tell!