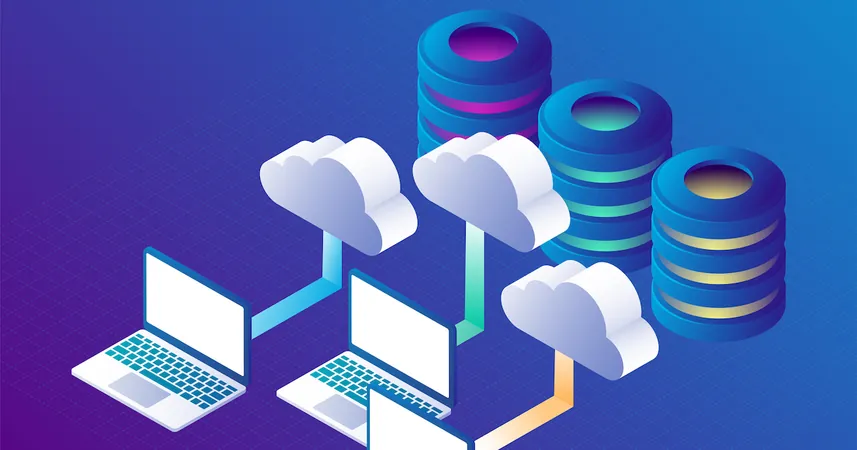
Meta's Revolutionary MobileLLM: A Game Changer for On-Device AI
2024-11-05
Author: Rajesh
Meta is making waves in the world of artificial intelligence with its ambitious MobileLLM project, which challenges the common narrative that larger models equate to better performance. In a bold move, Meta researchers are demonstrating that the true key to quality in smaller language models lies not in their size, but in the innovative design of their architecture.
The team at Meta has developed four groundbreaking models with parameter sizes of 125M, 350M, 600M, and 1B. These models have outperformed previous leading models by integrating unique features such as deep yet compact architectures, embedding sharing, and grouped-query attention mechanisms. This approach debunks the previously held belief, popularized by Kaplan, which suggested that performance gains in transformer models directly correlate with an increase in the number of parameters.
The researchers observed an important insight: for smaller models, deepening the network architecture plays a more significant role in enhancing performance compared to merely widening it. This revelation comes as the AI community wrestles with an explosion of data and a pressing need for more efficient models, especially for on-device applications where resource constraints are a reality.
A standout technique utilized in MobileLLM is embedding sharing, which was previously employed in the Meta TinyLlama project. This strategy allows the model to share weights across both input and output embedding layers, effectively reducing the overall parameter count without severely compromising accuracy. In fact, for the 125M-parameter model, the embedding layers contribute over 20% to the model’s total parameters, making this technique especially potent.
On a test basis, the 30-layer model at 125M parameters demonstrated that by implementing saving strategies through embedding sharing, the model could reduce parameters by 16M (around 11.8%), with only a minimal drop in accuracy. Remarkably, this minor accuracy loss could be compensated by reallocating resources to introduce additional layers, maximizing performance efficiently.
Moreover, Meta is also harnessing another innovative technique known as immediate block-wise weight sharing. This method allows for weight duplication between adjacent blocks, leading to reduced latency without a significant increase in model size. This strategy addresses the critical challenge of memory movement, a primary contributor to model latency, thus enhancing efficiency in on-device performance.
The impact of MobileLLM has been substantial, as researchers conducted extensive evaluations against previous state-of-the-art models in tasks such as zero-shot reasoning, question answering, and reading comprehension. The results were astonishing—MobileLLM-LS-125M not only matched but sometimes surpassed the performance of many conventional 350M models. Additionally, in the 350M category, MobileLLM exhibited a remarkable improvement, exceeding former performance benchmarks by more than four points.
As the demand for large language models skyrockets, particularly for mobile device applications, Meta underscores the urgency of this transition. The reliance on cloud computing leads to rising costs, latency issues, and concerning increases in energy consumption and carbon emissions. By emphasizing on-device model deployment, Meta aims to pave the way for a cleaner, more efficient future in AI while also improving responsiveness and overall user experience.
In conclusion, Meta's MobileLLM stands at the forefront of redefining what smaller models can accomplish, setting a strong, eco-friendly foundation for the future of large language models in mobile technology. The implications of this research are poised to resonate across various fields, enhancing the capabilities of AI right in our pockets. Stay tuned, as this may just be the beginning of a major shift in how we interact with AI.