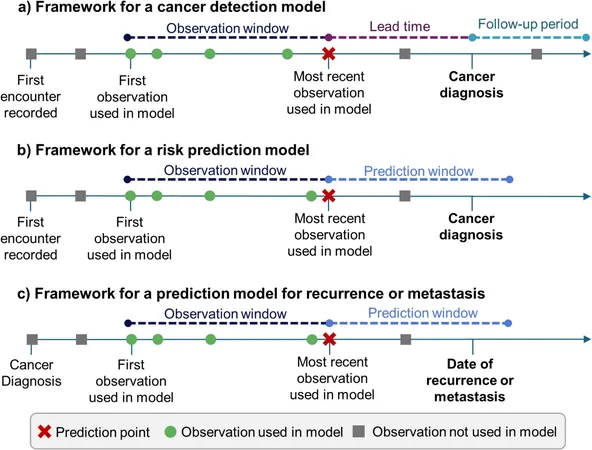
How AI is Revolutionizing Cancer Prediction: The Potential of Longitudinal Data from EHRs
2025-01-28
Author: Yu
Cancer remains one of the most formidable health crises worldwide, responsible for nearly 10 million deaths in 2022 alone, with projections indicating that the number of cases could soar to 35 million by 2050. The urgency for effective prediction models is critical, as early diagnosis can significantly enhance treatment efficacy and survival rates. With technological advancements, artificial intelligence (AI) is evolving into a powerful ally in this battle against cancer.
AI technologies, particularly machine learning (ML), hold the promise of transforming traditional healthcare paradigms. While statistical methods have long been employed in risk prediction models, AI offers a more nuanced approach, capable of sifting through extensive electronic health records (EHRs) to identify patterns that may elude human analysts. By examining retrospective data captured during patient care, such as lab results and diagnoses, AI can more accurately identify high-risk patients and facilitate timely medical interventions.
Typically, cancer prediction models have relied on static, cross-sectional analyses of EHR data. However, the application of longitudinal data is gaining momentum. This approach considers the temporal context of patient data, acknowledging that changes over time—like the transition from long-standing diabetes to new-onset diabetes—can be more indicative of cancer risk than isolated measurements. The dynamic nature of health data allows for a richer analysis, potentially leading to improved predictive models that consider a patient's evolving circumstances.
Longitudinal data harnesses the power of AI to address myriad healthcare challenges, including those encountered in predicting mortality and sepsis. However, its examination in the context of cancer prediction remains sparse. Current systematic reviews have not explicitly focused on utilizing longitudinal EHR data for cancer prediction, leaving a notable gap in research.
This scoping review intends to fill that void, summarizing AI methodologies employed to analyze longitudinal data for cancer predictions. It aims to: 1. Identify and describe various AI-based methods for predicting cancer from longitudinal EHR data. 2. Examine the time windows employed in these models and evaluate their efficacy. 3. Highlight potential biases in cancer prediction research, guiding future studies in designing robust models. 4. Offer recommendations for optimizing the design and reporting processes of longitudinal prediction models for enhanced clinical applicability.
To accomplish these objectives, an exhaustive search strategy was devised, evaluating various databases, including MEDLINE and PubMed, resulting in some 1214 studies. After rigorous screening, 35 studies were selected for detailed review.
The findings reveal a fascinating landscape of cancer prediction efforts spanning diverse populations and employing various methodologies. Notably, pancreatic and colorectal cancers have been the focus of many studies, likely due to their significant global health impact. Predominantly U.S.-based, the research also observed contributions from countries like the Netherlands, Taiwan, and South Korea—emphasizing the international commitment to leveraging AI for healthcare innovations.
Another interesting aspect to note is the necessity of implementing rigorous measures in the development and validation of prediction models. The scoping review found that a staggering 90% of the studies evaluated exhibited a high risk of bias. This calls for greater attention to data collection processes, particularly the consistency of health records across different clinical settings.
Amidst these findings, several recommendations have emerged. Researchers are urged to standardize terminology related to cancer prediction to enhance clarity. Exploring various prediction windows is essential to ascertain their optimality. Moreover, reproducibility should become a priority, with calls to share research code and highlight data sources—an essential step to ensure scientific integrity.
In conclusion, the application of AI in cancer prediction through longitudinal data from EHRs represents a groundbreaking frontier in medical research. As methodologies continue to evolve, maximizing the use of rich patient data has the potential not only to improve predictive accuracy but also to save lives by enabling earlier cancer detection and intervention. The journey ahead is filled with promise, and with diligent research and informed practices, we can harness AI's full potential to revolutionize cancer care. Stay tuned for what comes next in this exciting intersection of technology and healthcare!