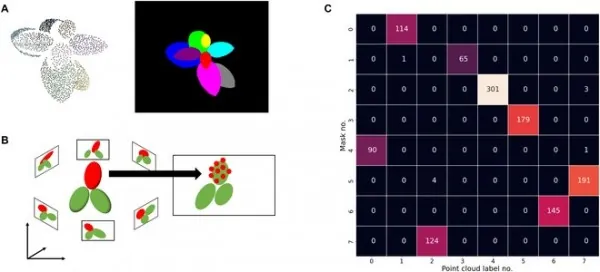
Groundbreaking Technique Transforms 3D Leaf Edge Reconstruction, Paving the Way for Enhanced Agricultural Practices
2024-11-11
Author: Ming
In a remarkable advancement for agricultural science, a new study has unveiled a revolutionary method for reconstructing the 3D edges of leaves, significantly enhancing the analysis of leaf morphology. This innovative approach, detailed in a recent publication in the journal *Plant Phenomics*, promises to unlock deeper insights into plant growth, ultimately optimizing agricultural productivity.
Leaves play a crucial role in photosynthesis and other vital physiological functions. Their diverse and complex shapes enable plants to adapt effectively to varying environmental conditions. However, traditional methods of measuring leaf morphology have primarily depended on 2D imaging techniques. These methods struggle to adequately represent the intricate 3D structures of leaves that are essential for a comprehensive understanding of their functionality and overall plant health.
The study, published on May 9, 2024 (DOI: 10.34133/plantphenomics.0181), proposes an advanced method for non-destructive analysis of leaf structures in three dimensions. Researchers employed a hybrid technique that merges deep-learning-based 2D instance segmentation with curve-guided 3D reconstruction methods. Initial tests were conducted on simulated leaf models under controlled conditions, revealing that performance varies with the support threshold (τt). Lower thresholds led to inaccuracies, while higher thresholds occasionally resulted in incomplete reconstructions.
A key challenge addressed in this research was leaf correspondence identification in dense plant scenes. Accurate reconstruction remains crucial as overlapping foliage can obscure individual leaves. Findings indicated that larger leaves were reconstructed more easily compared to smaller ones, particularly those with complex curvatures. Moreover, the method proved sensitive to camera noise, though it was less affected by the number of images taken or the levels of occlusion present.
Using Mask R-CNN on actual plant data, researchers successfully generated detailed masks for individual leaves. While the technique excelled with larger leaves, smaller ones faced hurdles, evident from lower average precision (AP) scores. Researchers applied this method to various soybean plants at different growth stages, showcasing its promise. However, complexities arose when analyzing highly occluded leaves or those that were particularly small, sometimes resulting in artifacts or distortions.
The research team, led by Dr. Koji Noshita, emphasizes that this methodological breakthrough will significantly enhance leaf morphology assessment. "By integrating deep learning with curve-based reconstruction techniques, we can achieve unprecedented detail in leaf analysis, possessing vital implications for agricultural research," said Dr. Noshita.
This pioneering 3D leaf edge reconstruction technique not only advances the field of plant phenotyping but also lays the groundwork for innovation in agriculture and ecological studies. Researchers believe that ongoing refinement of this approach will lead to practices that enhance agricultural efficiency, addressing global food security challenges head-on.
As the challenges of global food security continue to loom, advancements like this hold the potential to revolutionize agricultural techniques, allowing for better crop management and sustainable practices that can feed a growing population with finite resources. The future of agriculture may indeed be looking up, thanks to this cutting-edge research that bridges technology with nature’s complexity.