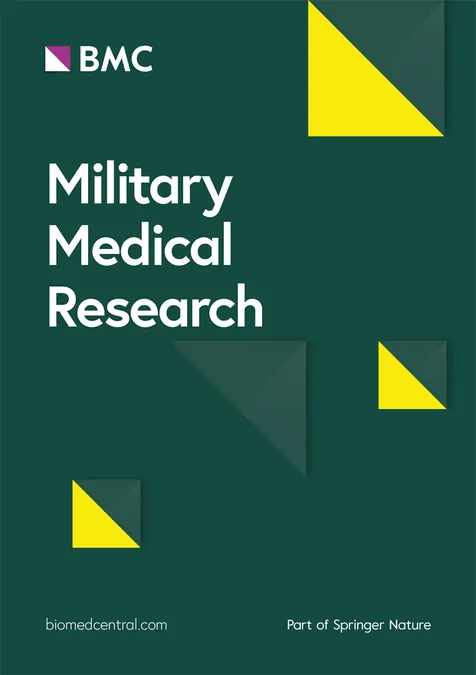
Are AI Virtual Cells the Future of Cancer Research? Unanswered Questions Abound!
2025-04-30
Author: John Tan
Unlocking the Potential of AI in Oncology
In a groundbreaking perspective piece, experts Carlos M. Ardila and Pradeep K. Yadalam explore the revolutionary possibilities of Artificial Intelligence Virtual Cells (AIVCs) in cancer research. Published recently in *Military Medical Research*, the article confirms that AIVCs could reshape the landscape of oncology by enabling sophisticated simulations and accelerating drug development. Yet, despite this potential, several critical questions remain unanswered.
The Challenge of Cellular Dynamics
AIVCs are designed to mimic the complex and ever-changing behavior of cancer cells. However, the authors point out a troubling gap: How will these virtual models handle the inherent randomness of cellular processes? Fluctuations in gene expression and molecular interactions are natural, but the current framework lacks clarity on how to differentiate between significant biological variations and technical noise. To enhance accuracy, could integrating stochastic differential equations with advanced machine learning techniques like Bayesian neural networks prove transformative?
Integrating Multi-Omics Data: A Double-Edged Sword
Another point of concern is how AIVCs will incorporate various omics data—each with unique scales and noise characteristics. While the ability to interpolate data across different time points is a game-changer, what measures will ensure the accuracy and reliability of these integrations? The authors suggest that using hybrid architectures could help normalize data from disparate sources. As omics technologies evolve, will AIVCs remain agile enough to incorporate groundbreaking data like single-cell multi-omics?
Synthetic Data: A Double-Edged Sword?
The concept of generating synthetic data to offset the scarcity of biological samples holds promise, especially in rare cancer types. Yet, is the reliability of generative AI models truly dependable? The commentary outlines three potential safeguards to enhance the validity of synthetic outputs, including adversarial validation and physics-based constraints. Will these strategies effectively mitigate risks of bias within generated data?
Personalized Medicine: Can We Scale?
The prospect of using AIVCs as personalized, patient-specific models opens exciting avenues for virtual drug trials. However, the scalability of these models raises questions. How can AIVCs adapt to individual variances like genetic makeup or environmental factors without losing relevance? A hierarchical modeling approach coupled with cloud-based solutions could potentially address computational challenges.
Bridging the Gap: Interdisciplinary Collaboration Needed
Despite discussing collaboration across disciplines, the article does not fully explore how to integrate expertise from diverse fields such as clinical practices, experimental science, and artificial intelligence. Establishing 'Translational AI Units' could facilitate this integration, ensuring that AIVCs are both scientifically sound and user-friendly. But what protocols will be necessary to protect patient data while enabling effective data sharing?
Conclusion: The Road Ahead for AIVCs in Cancer Research
While the perspective on AIVCs highlights transformative potential, addressing these unanswered questions is crucial for advancing cancer research. The proposed frameworks—ranging from stochastic modeling to collaborative research initiatives—could pave the way for effective and tailored cancer treatments. The scientific community eagerly anticipates tackling these challenges to harness the complete power of AIVCs in the battle against cancer.