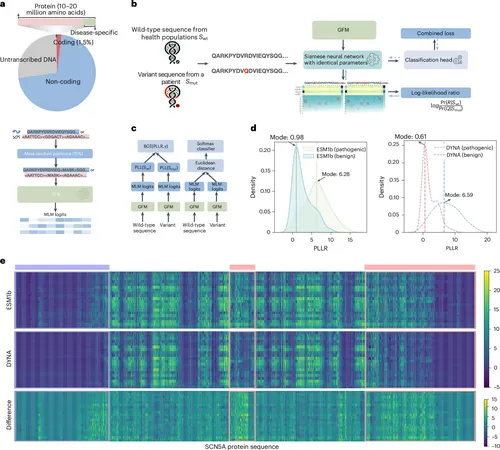
Groundbreaking AI Model Revolutionizes Prediction of Gene Variants Linked to Diseases
2025-03-25
Author: Nur
In a significant stride for personalized medicine, researchers at Cedars-Sinai have unveiled an innovative artificial intelligence model dubbed DYNA. This cutting-edge tool has shown the remarkable ability to distinguish harmful gene variations from benign ones, potentially revolutionizing how physicians diagnose and treat diseases.
The findings, published in *Nature Machine Intelligence*, highlight DYNA's superiority over current AI models when it comes to predicting the linkage between specific DNA mutations and various cardiovascular diseases and other disorders. This advancement could open new avenues for targeted therapies tailored to individual patients, enhancing the overall effectiveness of medical interventions.
"AI has dramatically increased our capacity to analyze vast amounts of genetic data across diverse populations," explained Huixin Zhan, Ph.D., a key contributor from the Department of Computational Biomedicine at Cedars-Sinai. "However, a substantial portion of these genetic variations—up to 50%—remains of uncertain significance, leaving us puzzled about their possible impact on health. DYNA addresses many of these uncertainties, making it a game changer in genetic research and clinical applications."
Current AI systems are adept at identifying gene variants likely to disrupt the structure or function of proteins, potentially leading to diseases. Nonetheless, they often fall short in correlating these variants to specific illnesses, thereby limiting their practical application in real-world medical scenarios. DYNA fills this critical gap by accurately linking gene variants to their corresponding diseases.
The development of DYNA involved the application of a sophisticated AI methodology known as a Siamese neural network to refine two existing models. By leveraging these enhanced models, researchers successfully predicted the likelihood that certain gene variants are associated with conditions such as cardiomyopathy (the enlargement and dysfunction of the heart muscle) and arrhythmias (irregular heartbeats).
In a rigorous validation process, the DYNA model's predictions were compared against data sourced from ClinVar, a reputable public database that catalogues genetic variations linked to specific diseases. The results indicated that DYNA effectively matched gene variants with the associated diseases.
Looking ahead, Jason Moore, Ph.D., another co-author and chair of the Department of Computational Biomedicine, expressed optimism about DYNA's potential impact. "This model creates a versatile platform for studying a range of genetic disorders. Future iterations of DYNA could be instrumental in equipping healthcare professionals with advanced tools designed to customize diagnoses and treatment plans based on each patient's unique genetic makeup."
This breakthrough represents a monumental shift in understanding genetic diseases, potentially enabling faster and more accurate diagnoses and paving the way for revolutionary advances in personalized medicine. The future of healthcare may very well lie in tailoring treatments not just to symptoms, but to the very genetics of the individual patient.