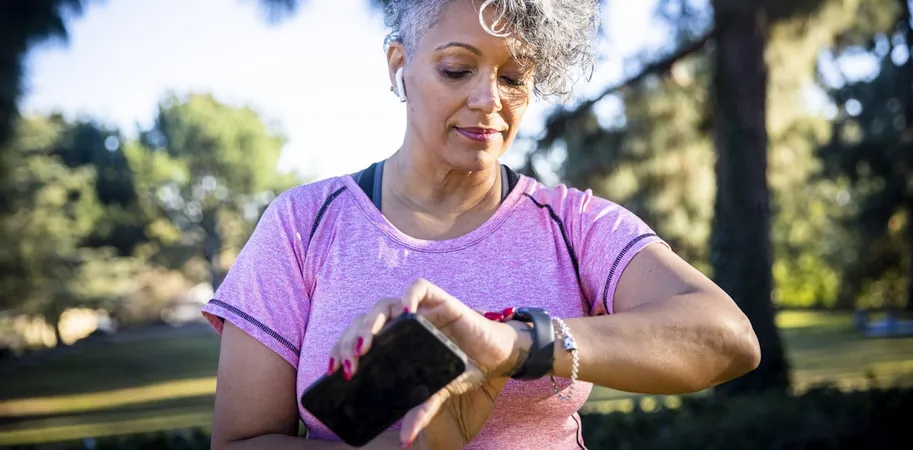
Revolutionizing Health: How AI Learns from Your Wearables
2025-04-10
Author: Michael
Unlocking Insights from Wearable Technology
Every day, our bodies create a wealth of bio-signals—everything from heart rates to sleep patterns—that can be captured by wearable devices like smartwatches. These signals not only reveal our immediate well-being but can also provide crucial insights into mood swings and a variety of health conditions.
The Challenge of Data Interpretation
Collecting bio-signal data may be relatively straightforward and cost-effective, thanks to studies that ask participants to wear devices for short periods. However, the real challenge lies in teaching machine learning algorithms to understand the connections between these bio-signals and specific health issues. That’s where tech-savvy researchers come into play.
Spotting Atrial Fibrillation
Many modern smartwatches, from brands like Apple and Samsung, are now equipped to detect atrial fibrillation—an irregular heart rhythm that, if neglected, can lead to severe complications like strokes. Machine learning algorithms are being trained to recognize the patterns of this condition within bio-signal data.
The Costly Labeling Process
To make this work, vast datasets of labeled bio-signals are required. Experts such as cardiologists manually sift through millions of entries to pinpoint instances of atrial fibrillation, which can be both time-consuming and costly. This issue isn’t unique to heart health; it spans many disorders.
Innovative Pretraining Solutions
To tackle these challenges, researchers are finding innovative ways to train algorithms with fewer labels by employing a technique known as pretraining. By using large, unlabeled datasets, algorithms can learn to identify valuable relationships even with minimal labeled examples.
Navigating Bio-Signal Obstacles
Unraveling the connections between bio-signals and health disorders is complicated by noise and personal differences. Bio-signals can be disrupted by physical movement, and individual uniqueness in physiological signals adds another layer of complexity. The relationship between any single bio-signal and a disorder is often obscured, making machine learning essential.
Pretraining: A Smart Warm-Up
Pretraining serves as a warm-up for machine learning algorithms, easing them into recognizing crucial relationships between bio-signals and disorders. Researchers employ techniques such as "filling in the blanks"—simulating gaps in bio-signals to teach algorithms to predict missing data.
Proven Success with Predictions
Once algorithms master predicting ordinary heartbeats, they can more easily adapt to identify irregular patterns characteristic of atrial fibrillation. The concept applies to various bio-signals, allowing pretraining on easy-to-collect data to streamline the learning process for more difficult disorders.
Speeding Up Diagnosis and Detection
Enhancing pretraining methods can significantly bolster the efficiency and accuracy of disease detection through machine learning. This not only reduces the need for expert labeling but also enables quicker development of proactive health monitoring features, like Google’s Loss of Pulse smartwatch function.
A Future of Early Detection
Armed with a rich array of bio-signals and advanced data analysis techniques, researchers are poised to uncover vital health insights that could revolutionize early diagnosis. Catching diseases in their infancy often makes treatments more effective, paving the path toward a healthier future.