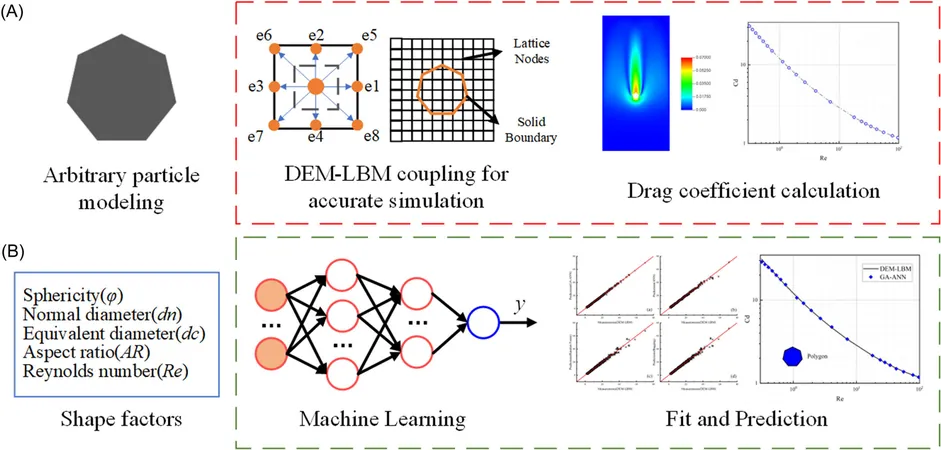
Revolutionizing Fluid Dynamics: AI Unveils New Strategies for Particle Movement Prediction!
2024-11-05
Author: Sophie
Introduction
Accurately modeling how particles move through fluids isn't just a technical challenge—it's a key factor influencing innovations in diverse fields like chemical engineering and aerospace. At the heart of this is the drag coefficient, a vital measure determining how particles settle and shift within fluid environments. While scientists have long understood the dynamics of spherical particles, predicting the drag coefficients of irregularly shaped particles has remained perplexing, representing a significant hurdle in fluid dynamics research.
Groundbreaking Study
In a groundbreaking study, a collaborative team from Nanjing University of Science and Technology and Westlake University has tackled this intricate issue head-on. Their research was published in the esteemed International Journal of Mechanical System Dynamics, shining a light on an innovative fusion of machine learning and advanced numerical methods. By applying the discrete element method (DEM) combined with the lattice Boltzmann method (LBM), they crafted a high-precision dataset tuned to unveil the complex interactions of non-spherical particles in fluids.
Machine Learning Models
The researchers developed four machine learning models to predict the drag coefficient of polygonal particles. Notably, one of these models achieved an impressive prediction error of less than 5%, marking a monumental leap forward in predicting particle-fluid interactions. This scientific breakthrough is set to reshape our understanding of fluid dynamics and enhance analytical capabilities.
Limitations of Traditional Methods
Typically, relying on single shape factors has proven inadequate for capturing the multiple elements affecting particle behavior. However, by leveraging DEM and LBM simulations, the research team generated a comprehensive dataset that empowered them to create several machine learning models. The standout performer was the genetic algorithm-artificial neural network (GA-ANN) model, which succeeded in minimizing prediction error dramatically. This highlights the profound impact machine learning can have in refining the accuracy of models related to particle-fluid dynamics.
Transformative Potential
Professor Cheng Cheng, a lead researcher on the project, emphasized the transformative potential of this study, stating, "This research demonstrates how machine learning can revolutionize our approach to complex problems in fluid dynamics. By harnessing the power of numerical simulations combined with AI, we've achieved a new level of predictive accuracy concerning the drag coefficients of polygonal particles, opening doors to advancements in both academic and industrial realms."
Implications of Findings
The implications of these findings are vast. Industries such as chemical processing, environmental engineering, and aerospace technology stand to benefit immensely from improved drag prediction models. Enhanced models can significantly optimize processes like sedimentation, filtration, and propulsion, outperforming existing systems by increasing their efficiency.
Conclusion
This research heralds an exciting new chapter in fluid dynamics, paving the way for future innovations aimed at harnessing the complexities of particle movement in various industrial applications. Stay tuned! The advancements stemming from this study might just lead to major breakthroughs that redefine the standards of efficiency across many sectors.