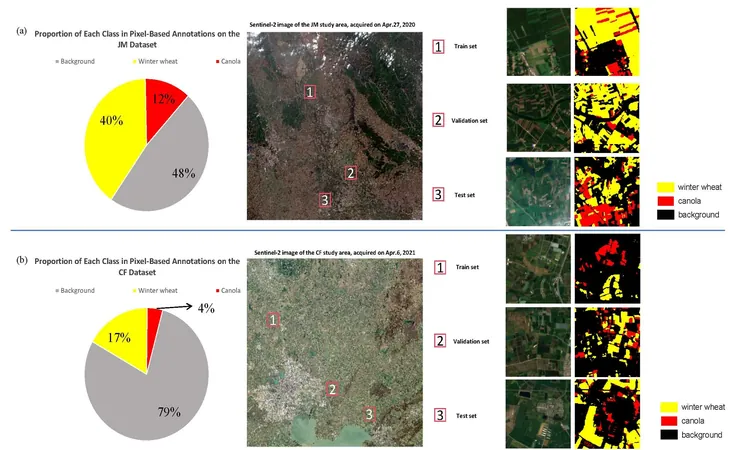
Revolutionary Dual-Branch Model Transforms Crop-Type Mapping in Fragmented Asian Farmlands!
2024-12-10
Author: Sophie
Introduction
In the vast agricultural landscapes of Asia, particularly in China, farmers often grapple with small, scattered fields that lack distinct boundaries. This chaotic arrangement poses significant hurdles for effective crop distribution and agricultural analysis, particularly when leveraging advanced remote sensing technology. However, a breakthrough is on the horizon.
The Dual-Branch Model
A dedicated research team from the Hefei Institutes of Physical Science under the Chinese Academy of Sciences has unveiled an innovative solution: the dual-branch deep learning model (DBL). This cutting-edge technology revolutionizes crop-type mapping in irregular agricultural settings across Asia.
Significance of the Research
According to Associate Professor Xu Taosheng, who spearheaded the research, 'This model addresses the intricate challenges associated with crop-type mapping in the diverse and often convoluted planting plots found throughout Asia.' The remarkable findings of their study were detailed in the prestigious journal, Remote Sensing of Environment.
Technical Overview
The researchers developed the dual-branch model utilizing state-of-the-art deep learning techniques paired with time-series datasets. The model features two distinct branches: one dedicated to capturing large-scale landscape patterns and another that hones in on minute details, such as slight variations in crop growth over time. This strategic combination empowers the DBL to accurately identify different crop types, even in complex and disorderly fields where traditional methods struggle.
Advantages of the Dual-Branch Model
One of the most significant advantages of this model is its ability to analyze both temporal and spatial data. 'Crops evolve and undergo changes over time, and our model meticulously tracks these transitions,' Xu explained. To enhance its precision in fragmented agricultural environments, the team developed two new datasets—CF and JM—that encapsulate the characteristics of scattered farmlands, including varying plot sizes and shapes.
Results and Accuracy
The results speak volumes: the dual-branch model achieved an impressive overall accuracy rate of 97.7%, with a commendable 90.7% accuracy in identifying specific crop types within small fields. This level of adaptability and precision showcases the model's potential for real-world applications, especially in regions where fragmented land management is the norm.
Conclusion and Future Implications
With such promising results, the dual-branch model has the potential to transform how farmers and agricultural analysts approach crop monitoring and management in Asia. This innovation not only enhances agricultural productivity but also positions remote sensing technology as a cornerstone for sustainable farming practices in the future.
Final Thoughts
Is this new model the game-changer the agricultural world has been waiting for? Only time will tell as it continues to be applied in real-world farming scenarios!