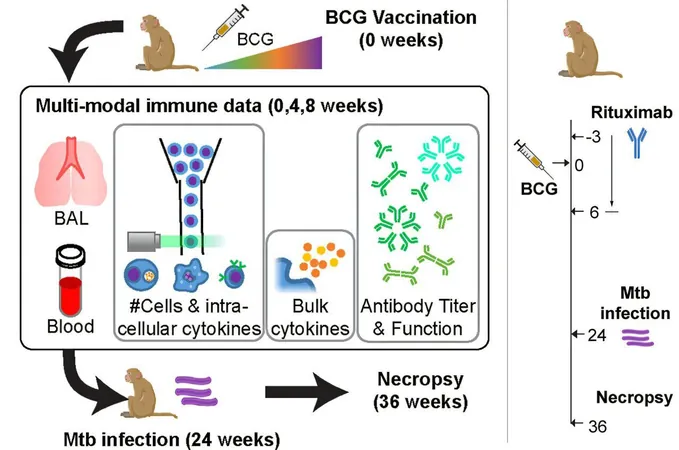
Revolutionary Computational Method Could Transform Our Understanding of Immunological Responses and Vaccines
2024-11-07
Author: Benjamin
Revolutionary Computational Method Could Transform Our Understanding of Immunological Responses and Vaccines
In the last two decades, the explosion of biotechnologies has enabled researchers to amass extensive biological datasets through large-scale experiments in genomics, transcriptomics, proteomics, and cytometry. While this data represents a treasure trove of biological insights, deciphering it, especially when studying complex biological systems like the human immune response, poses significant challenges.
A team of biological engineers at MIT has introduced an innovative computational model designed to extract valuable information from these massive datasets. Their groundbreaking approach successfully unraveled the intricate interactions driving the immune response to tuberculosis vaccination and subsequent infections, potentially revolutionizing vaccine development and our understanding of complex biological networks.
Douglas Lauffenburger, the Ford Professor of Engineering at MIT and the study's senior author, stated, “We've established a computational modeling framework that can predict repercussions of adjustments in elaborate systems involving multiple scales and diverse components.” The study highlights not only their findings but calls upon researchers across various fields to implement this versatile modeling technique.
Insights into Complex Biological Systems
By utilizing a combination of genomic sequencing, RNA transcript analysis, and proteomic investigations, scientists acquire a wealth of data regarding cellular gene variants, gene expression, protein presence, and cellular diversity. Traditional computational methods, often reliant on machine learning, struggled to explain the biological mechanisms behind the input-output relationships predicted by these models.
Lauffenburger pointed out the limitations of conventional AI methodologies in biological research, emphasizing the need to understand the processes occurring between inputs and outputs—an aspect he considered crucial for biological inquiry.
To tackle this issue, the research team adopted probabilistic graphical networks, a modeling approach commonly utilized in fields like speech recognition and computer vision but underexplored in biology. This enables a more detailed analysis of how various biological variables are interconnected, providing clearer insights into complex systems.
By employing a technique known as graphical lasso, the MIT researchers enhanced the capacity of their model to filter out extraneous correlations, thus revealing only the most critical interactions that define the system.
Dissecting the Immune Response to Vaccination
To validate their methodology, the research team studied a widely used tuberculosis vaccine called BCG (Bacillus Calmette-Guérin). Despite its prevalence in areas heavily affected by tuberculoses, its effectiveness can wane over time, leading to ongoing research into alternative delivery methods. Early animal studies had suggested that intravenous or inhalation delivery improved immune responses compared to traditional injection.
The MIT team analyzed comprehensive data from these studies, which included around 200 distinct variables, encompassing levels of cytokines, antibodies, and various immune cell types from approximately 30 animal subjects. By applying their probabilistic model, the researchers delineated the immune response pathways activated by the BCG vaccine, revealing that it primarily stimulates a specific subset of T cells. These T cells engage in producing cytokines that, in turn, activate B cells responsible for generating targeted antibodies against tuberculosis.
Lauffenburger likened their findings to a subway map, illustrating essential pathways leading to a strong immune response, even amid numerous other changes happening in the immune system. By predicting the effects of certain immune cell suppressions on vaccine efficacy, the model proved accurate, suggesting a bright future for its applications in vaccine development.
Future Implications and Applications
The implications of this research are far-reaching. It provides vaccine developers with a powerful tool for predicting the performance of potential vaccines, enabling informed modifications prior to human trials. Lauffenburger's lab is now extending its modeling efforts to investigate a malaria vaccine recently administered to children in Kenya, Ghana, and Malawi, as well as studying tumor microenvironments to anticipate cancer responses to various treatments.
With promising advancements in computational biology on the horizon, the ability to model and understand the complex interactions within biological systems continues to pave the way for revolutionary breakthroughs in medicine and vaccine development.
**Stay tuned, because the future of immunological research is bright, and we may soon unlock even greater secrets about our immune system and how to best harness it for health advancements!**