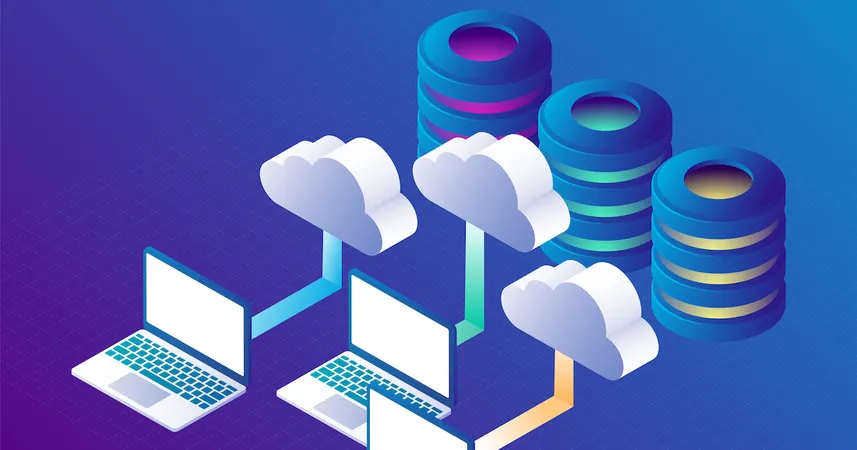
Meta's MobileLLM Revolutionizes On-Device AI: Smaller Is Better!
2024-11-05
Author: Noah
Meta's MobileLLM Revolutionizes On-Device AI: Smaller Is Better!
Meta's pioneering research team has set their sights on redefining the landscape of large language models (LLMs) with their groundbreaking MobileLLM initiative. Their ambitious goal is to demonstrate that the quality of smaller AI models is not merely a function of parameter count, but rather the result of meticulous architectural design. Through innovative techniques, Meta has developed four models with parameter sizes ranging from 125 million to a staggering 1 billion, each outshining previous benchmarks in accuracy.
One of the key breakthroughs of MobileLLM is its departure from the long-held "scaling law" theory—originally posited by researchers including Kaplan—that suggests model performance improves solely with an increase in parameters. Meta’s findings are turning the tables. They reveal that for smaller models, a deeper architecture dramatically enhances performance compared to mere width adjustments. The researchers' experiments indicate that optimizing architectural depth is critical for achieving superior results.
Previously implemented in the Meta TinyLlama model, embedding sharing has been a cornerstone of their new architecture. This technique involves reusing weights in the input and output embedding layers, thereby significantly reducing the overall number of parameters and streamlining the model's architecture. For lesser parameter counts, such as the 125M model, this approach can make a substantial impact, with embedding layers representing more than 20% of total parameters. Specifically, for a 30-layer model of 125 million parameters, employing weight sharing can lead to an 11.8% reduction in total parameters with only a minor drop in accuracy. Remarkably, this slight dip can be countered by redistributing saved parameters to incorporate additional layers, effectively enhancing model performance.
In addition to embedding sharing, another innovative technique browsers are thrilled about is immediate block-wise weight sharing. By allowing weights to be replicated between adjacent blocks, this method can curtail latency without considerable growth in model size. This is particularly advantageous in scenarios where memory movements predominantly dictate model latency.
Equipped with these cutting-edge strategies, MobileLLM strives to establish a robust framework for efficient design of smaller models. Meta researchers conducted extensive comparisons between MobileLLM and existing state-of-the-art sub-billion parameter models across various challenging tasks, including zero-shot common sense reasoning, question answering, and reading comprehension. For instance, the MobileLLM-LS-125M model matched or even surpassed the performance of numerous earlier 350M models in zero-shot reasoning tasks. Likewise, the 350M iteration of MobileLLM outperformed its predecessors by over 4 points while maintaining a similar or reduced model size.
The implications of these advancements are vast. Meta articulates a pressing demand for LLMs optimized for mobile devices—aiming to curtail cloud service costs and latency. Furthermore, they raise an alarm regarding the rising energy consumption and carbon emissions linked to mainstream larger models. By transitioning to on-device AI approaches, Meta proposes a solution that not only addresses environmental concerns but also markedly enhances model performance by reducing latency.
With the advent of MobileLLM, the future of AI on mobile platforms looks promising. As we navigate through an era increasingly focused on sustainability and efficiency, Meta's advancements may very well be a game changer in the quest for greener, faster, and more effective AI solutions. Stay tuned, as this could redefine how we interact with technology on our devices!