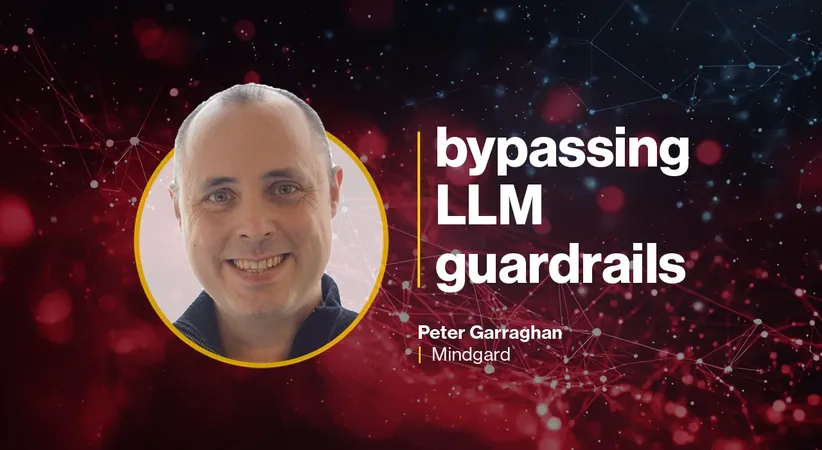
Why AI Guardrails Are Not Enough: The Shocking Truth Exposed!
2025-05-12
Author: Li
The Vulnerabilities Lurking in AI Guardrails
In a riveting interview with Dr. Peter Garraghan, the CEO of Mindgard, alarming insights into the world of AI model protection were revealed. His team’s groundbreaking research uncovers vulnerabilities within the guardrails designed to shield multi-billion-dollar large language models (LLMs) from attacks—vulnerabilities that can be exploited using surprisingly simplistic methods, even emojis!
Guardrails: Not the Silver Bullet They Seem
Many in the security sector elevate AI-based guardrails as essential defenses against malicious prompts. Yet Dr. Garraghan challenges this notion, likening guardrails to firewalls: not a standalone solution but part of a broader defense strategy. Relying solely on guardrails is like banking on a single bulletproof vest in a firefight—misplaced faith in their effectiveness against determined attackers is a common pitfall.
The Alarming Evasion Rates via Emojis and Unicode!
Mindgard’s research highlights a shocking trend: attackers can achieve near 100% success in evading detection using simplistic techniques like emoji and Unicode tag smuggling. These methods exploit fundamental weaknesses in how guardrails preprocess and tokenize inputs, creating a disastrous blind spot.
How Basic Tactics Outwit Advanced Systems
Why are these rudimentary tactics so effective? The guardrails rely on tokenizers to segment text into manageable pieces. However, when adversaries embed harmful content within sophisticated Unicode structures, the tokenizer fails to recognize the danger, often collapsing the harmful prompt into a benign-looking input that goes undetected.
The Disconnection Between AI and Human Understanding
Dr. Garraghan sheds light on a critical disconnect: AI models process information in a way that often diverges dramatically from human interpretation. This disparity complicates the establishment of clear, explainable defenses against adversarial attacks.
Guardrails vs. LLMs: A Fundamental Mismatch
A core concern is the standalone nature of most guardrails, which typically function as basic NLP classifiers. This misalignment means that they often can’t keep pace with the sophisticated operation of the LLMs they’re meant to protect, allowing manipulated inputs to slip through unnoticed.
The Way Forward: Evolving Security Practices
To enhance AI security, Dr. Garraghan advocates for a paradigm shift from static to dynamic defenses. Guardrails must be tested in conjunction with the actual LLM and monitored in real time to identify unusual behaviors. Implementing adversarial training and continuous testing helps to patch vulnerabilities before they can be exploited.
Looking Ahead: The Future of AI Guardrails
As AI systems grow increasingly powerful and multi-faceted, research must pivot towards comprehensive defensive strategies that don’t just focus on generic benchmarks but engage with real-world threats. Collaborating across disciplines to understand the nuances of threats and defenses is essential for future-proofing AI applications.