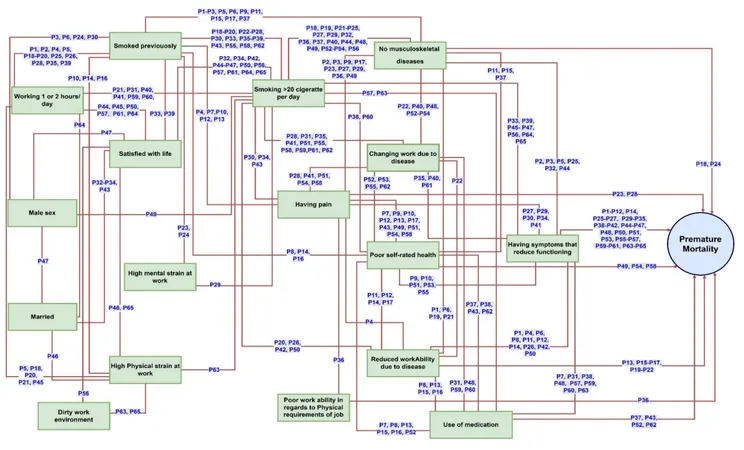
Unraveling the Hidden Causes of Premature Death: Insights from Finland's Longitudinal Study
2025-01-27
Author: Sarah
Data Collection Methodology
The study began by gathering comprehensive data from a robust sample of municipal workers through structured questionnaires initiated in 1981. Out of the 7,344 questionnaires dispatched, 6,257 responses were collected from individuals who had a minimum of five years' tenure within the municipal sector, achieving a remarkable response rate of 85.2%. This establishes a solid foundation for exploring the factors influencing premature death.
Crucially, mortality data were meticulously sourced from the Finnish national population register, allowing researchers to link questionnaire responses to actual death occurrences until March 31, 2010. This linkage formed the bedrock for determining premature mortality rates based on life expectancy data from the baseline year of 1981.
What Constitutes Premature Mortality?
To quantify premature death, researchers established a metric that integrates an individual’s age, sex, and life expectancy at the study's commencement. Participants who passed away before reaching their actuarial life expectancy were identified as having died prematurely, with a strict temporal buffer to minimize overclassification errors.
Identifying Predictive Factors
The research examined an extensive range of 80 variables categorized into sociodemographic factors, health and functioning metrics, lifestyle behaviors, and work-related conditions. Key sociodemographic variables included age, marital status, and educational background. Health metrics encompassed a self-assessment of health status, body mass index (BMI), and reported chronic illnesses.
Moreover, lifestyle factors such as smoking habits, alcohol consumption, and levels of physical activity were incorporated, while subjective experiences related to emotional well-being and work satisfaction complemented the analysis.
The GUHA Data Mining Method: A New Frontier in Epidemiological Research
The GUHA (General Unary Hypothesis Automaton) method, distinct from traditional data mining techniques, operates on a logical formalism that allows for systematic hypothesis generation and testing. By employing this framework, the researchers were able to uncover significant associations within the dataset that might otherwise remain hidden in conventional analyses.
Utilizing LISp-Miner software for data analysis, researchers looked for variable combinations correlated with premature death. The analysis required sophisticated contingency table evaluations to test the strength and validity of detected associations.
Remarkable Findings on Premature Mortality Pathways
The study yielded 67 unique paths of multi-predicate associations related to premature mortality, spotlighting critical health behaviors like smoking—specifically, smoking more than 20 cigarettes daily emerged as a substantial predictor. Indeed, among the study population, 70.4% of the recorded 2,196 deaths were deemed premature, further underscoring the vitality of understanding these associations.
In terms of gender disparities, distinctive patterns surfaced. Men exhibited a higher prevalence of significant predictors linked to premature mortality, predominantly revolving around smoking and physical health complaints, while women's paths included more health and emotional factors.
Sensitivity Analysis Confirms Findings
Subsequent sensitivity analyses affirmed the robustness of these findings across varying buffer periods for defining premature mortality. This multi-faceted analytical approach provided enhanced validation of the discovered associations, ensuring they were not mere artifacts of data manipulation or chance.
Implications for Public Health
The insights garnered from this extensive Finnish study not only underscore the multifactorial nature of premature mortality but also highlight the pressing need for tailored public health interventions. Targeting behavioral health—particularly smoking cessation and improvement of life satisfaction—could drastically uplift the longevity and well-being of municipal workers and wider populations.
As researchers continue to unravel the web of predictors linked to premature mortality, the GUHA method stands as a powerful tool, unlocking previously hidden correlations that may drive future public health policies and research initiatives aimed at reducing mortality rates.
Conclusion
This expansive examination of the factors contributing to premature death in Finnish municipal employees illuminates critical areas for further research and action, offering hope for older generations navigating health challenges in modern society.