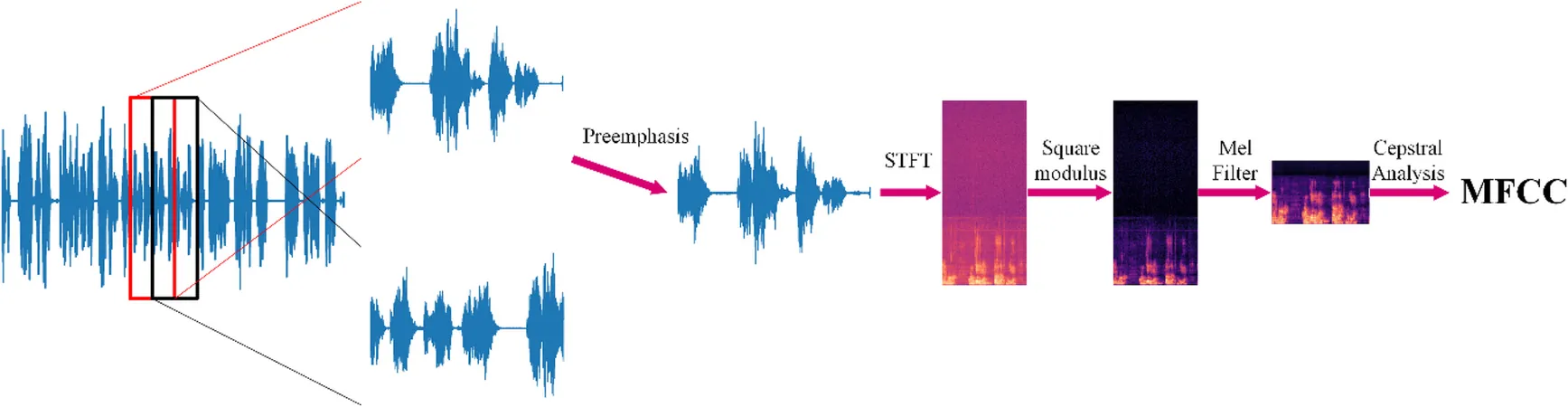
Unlocking the Voice of Schizophrenia: Can Speech Patterns Predict Mental Illness?
2025-05-08
Author: Sarah
The Silent Epidemic: Understanding Schizophrenia
Schizophrenia affects roughly 1% of the global population, casting a shadow of distress over patients and their families. Alarmingly, recent data from China indicates a steep increase in its prevalence over recent decades, revealing a worrying trend that healthcare systems must address head-on.
Decoding the Complexity of Diagnosis
Diagnosing schizophrenia is no easy feat. Symptoms vary wildly among patients, leading to challenges even for seasoned psychiatrists. Traditional diagnostic methods often fall short, resulting in misdiagnoses. This highlights the pressing need for innovative diagnostic models that can simplify this process.
Speech: The Hidden Clue in Diagnosing Schizophrenia
Recent advancements in speech analysis have shed light on the significant differences between the speech patterns of those with schizophrenia and healthy individuals. These discrepancies can reveal crucial insights into a patient's condition, particularly through the lens of their emotional state.
Harnessing Deep Learning to Analyze Speech Patterns
The integration of deep learning techniques has started to revolutionize how we analyze these speech patterns. By studying acoustic signals, researchers have achieved remarkable accuracy in predicting emotional symptoms related to schizophrenia. For instance, one study reported an astounding 97.76% accuracy using innovative speech detection models.
The Role of Emotional Expression in Speech
What makes speech analysis particularly challenging is the nuance of emotional expression. Patients often exhibit impaired emotional recognition, leading to subtle yet significant differences in how they convey thoughts. A comprehensive speech analysis model must account for these variations, taking into consideration demographic information as well.
Building a Robust Detection Model
To create an effective detection model that truly reflects individual differences, controlled studies using standardized texts with varying emotional cues have been employed. This approach aims to isolate semantic interference and enhance diagnostic accuracy by fusing various layers of audio analysis.
Research Framework: A Look at the Participants
In an extensive study involving 230 participants—156 diagnosed with schizophrenia—the selection criteria were rigorous. This included a thorough screening to ensure the integrity of the data collected, essential for extracting meaningful insights.
The Power of Deep Neural Networks
With the use of cutting-edge convolutional neural networks (CNNs), researchers are learning to detect schizophrenia through nuances in vocal patterns. The result? Unprecedented accuracy levels in identifying mental health conditions based on speech alone.
Insights from the Study: Speech as a Diagnostic Tool
Utilizing the advantages of speech patterns, researchers have gleaned insights that traditional diagnostic methods could not provide. By focusing on the subtle differences in speech, especially under neutral emotional stimuli, diagnostic accuracy has soared.
Future Directions: What Lies Ahead?
This groundbreaking research opens the door to the potential for real-time speech analysis in clinical settings. However, to maximize its efficacy, further validation across diverse groups and conditions is necessary, alongside a deeper understanding of the cross-over with other mental illnesses.
Conclusion: Voices of Change
Ultimately, decoding the speech of patients offers hope for better diagnostics, treatment, and understanding of schizophrenia. As we refine these techniques, we move closer to alleviating the stigma and burden of mental illness, one voice at a time.