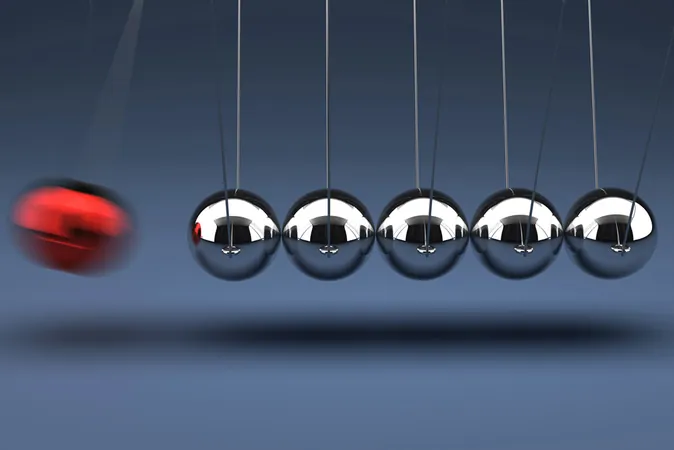
Unlocking the Secrets of Causality in Complex Systems: A Revolutionary MIT Algorithm
2024-11-01
Author: Nur
Introduction
Understanding causality is crucial for interpreting the intricate web of interactions that shape our world. Whether it’s the migration patterns of different animal species, fluctuations in stock market prices, regional voting behaviors, or evolving climatic conditions, grasping what causes one variable to shift can empower us to influence future outcomes effectively.
The Challenge of Complex Systems
However, pinpointing the root causes in complex systems is often daunting. Variables can intertwine, creating a convoluted tapestry that obscures clear causal relationships. In an exciting development, engineers at MIT have pioneered a groundbreaking algorithm designed to unravel these complexities and identify crucial influencing variables within multifaceted systems.
How the Algorithm Works
This innovative algorithm processes time-series data—such as the changes in marine species populations—assessing how different variables interact. For example, it can track how fluctuating sardine populations might predict anchovy numbers in the same marine ecosystem. By constructing a 'causality map,' the algorithm reveals likely cause-and-effect relationships, delineating whether variables interact synergistically (where one variable’s effect relies on another) or redundantly (where two variables exert similar effects independently).
Causal Leakage and Versatility
One standout feature of the algorithm is its ability to detect 'causal leakage.' This term describes the extent to which unexplained phenomena influence a system's behavior, suggesting that unseen variables may also be affecting outcomes.
Álvaro Martínez-Sánchez, a grad student in MIT's Department of Aeronautics and Astronautics, emphasizes that this method is versatile across disciplines. The implications extend beyond biology; it can enhance our understanding in fields ranging from neuroscience—tracking neuronal communication—to climatology, providing insights into weather patterns.
Applications in Aerospace Engineering
The MIT team plans to apply this algorithm in aerospace engineering to optimize aircraft designs and minimize fuel consumption. Adrián Lozano-Durán, an associate professor in the same department, notes that incorporating causality into models can significantly improve our grasp of the relationship between design aspects and overall aircraft efficiency.
Building on Previous Techniques
Additionally, the researchers are not operating in a vacuum. This work builds upon previous computational techniques that sought to establish causal links within complex systems. However, many of these past methods failed in specific circumstances, especially when distinguishing types of causality, such as unique, synergistic, or redundant influences.
Inspiration from Information Theory
In developing their approach, the engineers drew inspiration from information theory, which deals with how messages communicate through networks based on Claude Shannon's foundational work. This new algorithm regards the system as a network where variables transmit information, shedding light on their interdependencies.
A Broader Understanding of Causality
By simultaneously evaluating multiple variables, rather than examining pairs, this method provides a more nuanced understanding of causality. It establishes a system for measuring the transfer of information between variables, enabling the construction of causality maps that clarify interactions and predict how changes ripple through the system.
SURD: A Game-Changing Algorithm
The innovative algorithm, named SURD (Synergistic-Unique-Redundant Decomposition of causality), has already undergone rigorous testing through various benchmark scenarios—from predator-prey dynamics to environmental data tracking—and has consistently identified causal connections, outperforming existing methods.
Potential Applications and Impact
The potential applications of SURD extend across numerous fields, including climate science, economics, public health, social sciences, and fluid dynamics. By unveiling hidden causal networks, this research could ignite transformative advancements across scientific and engineering disciplines.
Conclusion
In summary, the MIT team’s development marks a significant leap in our ability to penetrate the complexities of causality. This research not only augments our understanding of systems but also equips us with the tools to predict and influence future outcomes in various sectors—highlighting the profound impact of recognizing and understanding the causal fabric of our interconnected world.