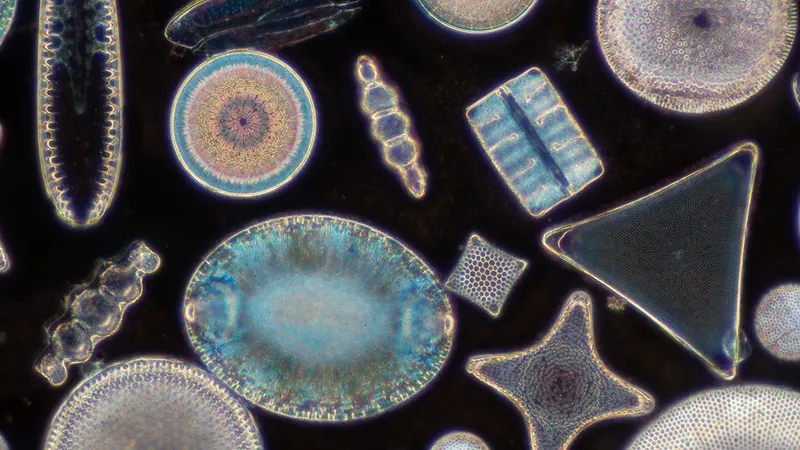
Transforming Biogeosciences: How Machine Learning Revolutionizes Image Analysis of Microorganisms
2024-11-06
Author: Daniel
Introduction
In an exciting advancement for the field of biogeosciences, researchers are leveraging machine learning to enhance the identification and cataloging of microorganisms found in aquatic sediments and the fossil record. A recent study published in the Journal of Geophysical Research: Biogeosciences highlights these innovative methods, which are crucial for understanding how these communities respond to the drastic environmental changes associated with climate change.
The Role of Diatoms
Diatoms, an extraordinary group of microscopic, photosynthetic organisms, play a pivotal role in global ecosystems. They are responsible for a significant share of the Earth's net primary productivity—essentially, they help sequester carbon and release oxygen into our atmosphere. However, when these organisms die, their fragile silica glass shells fracture into tiny pieces, complicating the identification process. Distinguishing these delicate frustules from the surrounding sediment has traditionally relied on labor-intensive manual techniques, leaving room for error and uncertainty.
Machine Learning as a Game-Changer
This is where machine learning steps in as a game-changer. By utilizing Convolutional Neural Networks (CNNs), researchers can significantly enhance their ability to analyze microscopic images. CNNs, a sophisticated form of deep learning, operate by organizing and optimizing the connections among numerous nodes, vastly improving the pattern recognition process. Unlike conventional feed-forward networks that confine learning to specific image locations, CNNs can generalize knowledge across different regions of an image, making them adept at identifying and categorizing diatoms amidst background clutter.
Innovative Methodology
In a groundbreaking paper, Godbillot and colleagues have pioneered a deep learning methodology that enhances the detection and classification of diatom species. This innovative approach not only streamlines the identification process but also allows scientists to track changes in species abundance and distribution, providing critical insights into the ecological impacts of climate change. As a result, this research signals the dawn of a new era for biogeoscientific tools, which can also be adapted for other challenges involving large, complex datasets.
Future Prospects
Expect to see a significant rise in the application of convolutional neural networks in biogeosciences and related fields in the upcoming years. The integration of such advanced AI techniques will likely open new frontiers in our understanding of microbial life and its response to global environmental shifts, making this a thrilling time for scientists dedicated to unraveling the complexities of our planet's ecosystems.
Conclusion
With the relentless pace of climate change impacting biodiversity, tools like these are more than just technological advancements—they are essential for informing conservation efforts and safeguarding Earth's fragile ecosystems.