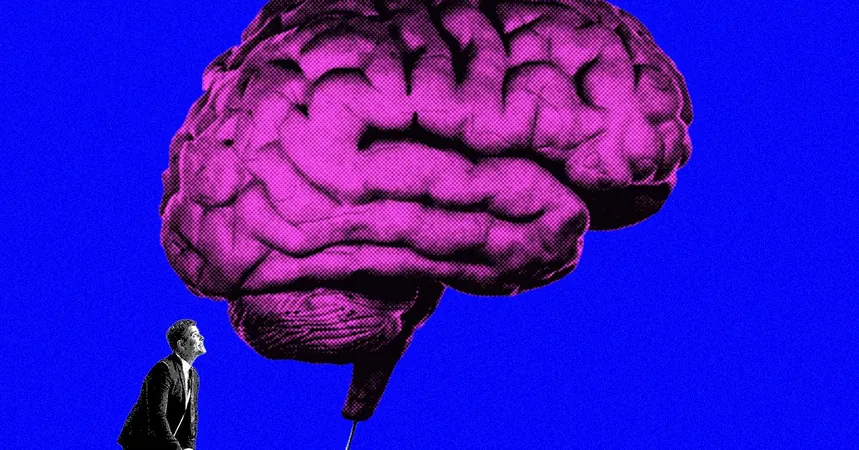
Transforming AI: How Databricks' Innovative Trick is Revolutionizing Model Performance Without Clean Data
2025-03-25
Author: Li
Introduction
Databricks, a pioneering leader in AI solutions for large enterprises, has devised a groundbreaking machine-learning technique designed to elevate the performance of artificial intelligence models without the traditional dependence on clean, labeled data.
The Dirty Data Dilemma
Jonathan Frankle, the Chief AI Scientist at Databricks, has meticulously engaged with numerous clients over the past year to uncover the prevalent obstacles impeding the reliable application of AI technologies. A major concern cited by Frankle is "dirty data," which hinders organizations from effectively training models for specific applications. As he aptly states, "Everyone has some data and an idea of what they wish to achieve, but the absence of clean data complicates the fine-tuning process to meet specific needs."
Empowering Businesses with Innovative Approaches
Databricks' innovative approach empowers businesses to develop their AI agents—software designed to carry out complex tasks—without allowing data quality issues to become a significant barrier. This technique unveils a unique perspective on the cutting-edge strategies engineers are implementing to enhance the capabilities of advanced AI systems, particularly in environments where quality data is scarce.
Reinforcement Learning and Synthetic Data
The method is built upon the principles of reinforcement learning—a technique that enables AI models to enhance their performance through iterative practice—and the creation of "synthetic," or AI-generated, training data. Notably, major players in the industry, including OpenAI, Google, and DeepMind, heavily incorporate these methodologies into their latest models. Not only does this highlight a growing trend, but it also aligns with emerging developments, such as Nvidia's reported acquisition of Gretel, a firm specializing in synthetic data generation.
The Best-of-N Method and Databricks Reward Model
The novel technique from Databricks is known as the "best-of-N" method. Essentially, it demonstrates that even subpar models can achieve commendable performances through repeated attempts. Databricks trained its model to predict human preferences among various outputs, creating a reward model named DBRM (Databricks Reward Model). This model subsequently enhances the performance of other models without requiring further labeled data.
Test-time Adaptive Optimization (TAO)
Using DBRM, Databricks identifies superior outputs, generating synthetic training data that is pivotal for further refining models to ensure improved outcomes from the onset. This advanced strategy is termed Test-time Adaptive Optimization (TAO). Frankle elaborates, "This method leverages relatively lightweight reinforcement learning to essentially integrate the benefits of best-of-N into the model itself." Encouragingly, research indicates that the TAO technique exhibits enhanced effectiveness when utilized on larger, more sophisticated models.
Commitment to Transparency and Custom Models
What sets Databricks apart is its commitment to transparency, showcasing its capabilities to develop potent custom models for its clients. The company has previously demonstrated its prowess with the development of DBX, a state-of-the-art open-source large language model (LLM) created from the ground up.
Real-world Applications and Challenges
In an age where companies are increasingly looking to adopt LLMs for automating tasks—from analyzing financial statements to processing health records—the lack of meticulously curated data remains a significant hurdle. For instance, in the finance sector, a well-functioning AI agent could analyze essential performance indicators and automatically compile reports for different analysts, while a healthcare AI might guide users to pertinent drug information or health conditions.
Performance Evaluation and Industry Impact
In a recent evaluation against FinanceBench—a benchmark assessing the proficiency of language models in answering financial queries—Databricks’ TAO method propelled the performance of the Llama 3.1B model from a mere 68.4% to an impressive 82.8%, eclipsing established models from OpenAI. This leap created a ripple effect of excitement regarding the potential of leveraging the TAO technique across various applications.
Expert Opinions on the Approach
Christopher Amato, a computer scientist at Northeastern University specializing in reinforcement learning, commended the approach, noting its promising implications while pointing out the persistent challenge of insufficient training data. Although he acknowledges the unpredictability sometimes associated with reinforcement learning, he believes that the TAO method could facilitate more scalable data labeling and foster continuous performance enhancement as models evolve.
Conclusion
Frankle reassures that Databricks is actively employing the TAO method to enhance its clients’ AI models and is paving the path for them to construct their own functional agents. One notable case involved a health-tracking app, which previously faced reliability issues in its AI model, but has now successfully integrated the TAO approach, thereby ensuring that the app delivers medically accurate information.
As Databricks continues to push the boundaries of AI model development, it beckons a new era where organizations can realize the full potential of artificial intelligence—transforming industries one algorithm at a time!