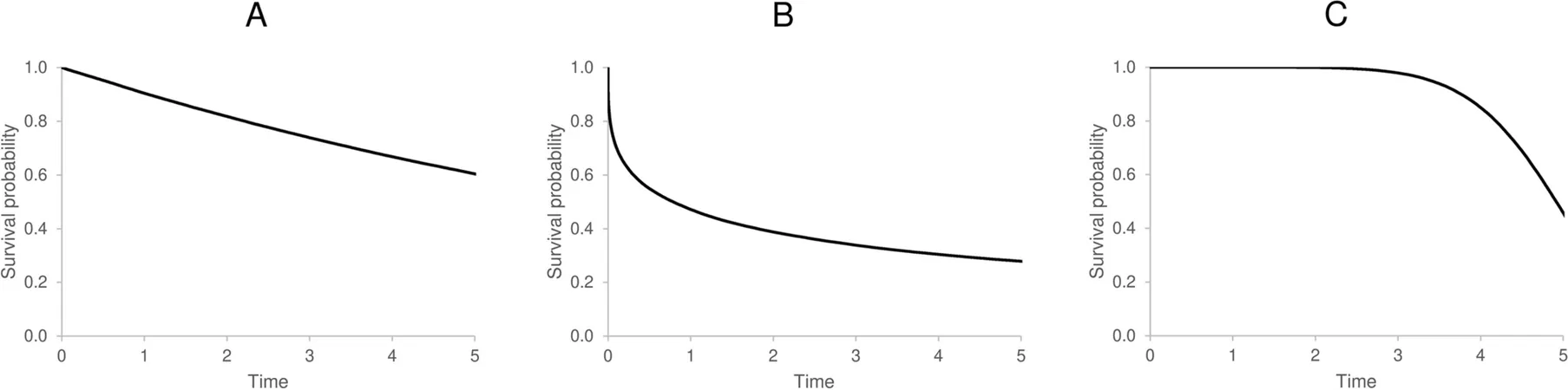
The Hidden Dangers of the Poisson Model: How Immortal Time Bias Distorts Health Outcomes!
2024-11-09
Author: Li
Background
In the world of epidemiology, one of the most significant pitfalls in observational studies involves the infamous immortal time bias. This bias can dramatically skew the perceived relationship between exposure and outcomes, particularly when dealing with health data where exposure can change during a patient's follow-up period.
To tackle this issue, researchers often turn to time-dependent analyses such as the Cox and Poisson regression models. While these models are valuable tools, the Poisson model comes with a critical caveat: it assumes that the event rate remains constant over time. When this assumption is violated, especially in situations with time-varying risks, the results can be misleading.
Methods
Our investigation utilized a simulation study designed to explore the effects of the Poisson model under different scenarios of hazard dynamics. We operated under the Weibull distribution, known for its flexibility in modeling time-to-event data, and we established three scenarios: a constant hazard, a decreasing hazard, and an increasing hazard over time.
The simulation also considered time-varying exposure, allowing patients to switch from unexposed to exposed once during the study. Through fitting the Poisson model to these simulated data sets, we tracked how the exposure-outcome relationship was impacted under the various hazard conditions.
Results
The findings were astounding! Even minor fluctuations in risk over time led to significant biases in estimating the exposure-outcome relationship. Specifically, when the risk of an event decreased or increased, the Poisson model consistently underestimated or overestimated the true exposure effect when compared to the actual outcomes. The degree of bias heightened with the prevalence of exposure and the timing of when exposure occurred during the study.
Conclusions
Our analysis revealed startling revelations about the Poisson model: using it without adjusting for time-varying risks can lead to seriously biased estimates. When evaluating situations where outcome risks fluctuate, researchers must take care to adjust for these variations, or alternatively, consider using models that do not assume constant risks, like the Cox model.
Given the urgency of accurately assessing health risks, especially during pregnancy, our study broadened its scope to real-world data. We examined the connection between antibiotic use during pregnancy and short-term neonatal outcomes using comprehensive healthcare databases from Lombardy, Italy.
The implications of this research are profound, as our findings suggest a potentially inflated risk for adverse neonatal outcomes among women who used antibiotics during the third trimester when analyzed through the Poisson model—a stark contrast to what was observed with the more flexible Cox model.
Real-World Implications
The Poisson model’s limitations are crucial to address in various healthcare contexts. For instance, risks for adverse outcomes stemming from cardiovascular events or pregnancy complications can vary significantly over time. Misinterpretation of these risks due to over-reliance on the Poisson model can lead to misguided health policies and treatment protocols.
Stay informed and aware of the model you choose in your research projects, as it can mean the difference between tracing accurate health trends and propagating erroneous medical conclusions.
In conclusion, while the Poisson model provides invaluable insights when used correctly, it also holds hidden dangers that could compromise our understanding of epidemiology. Researchers must take heed to adjust for the trend in outcome risk or else explore other modeling avenues to ensure their findings genuinely reflect causal relationships. The stakes for patient health are far too high to ignore!