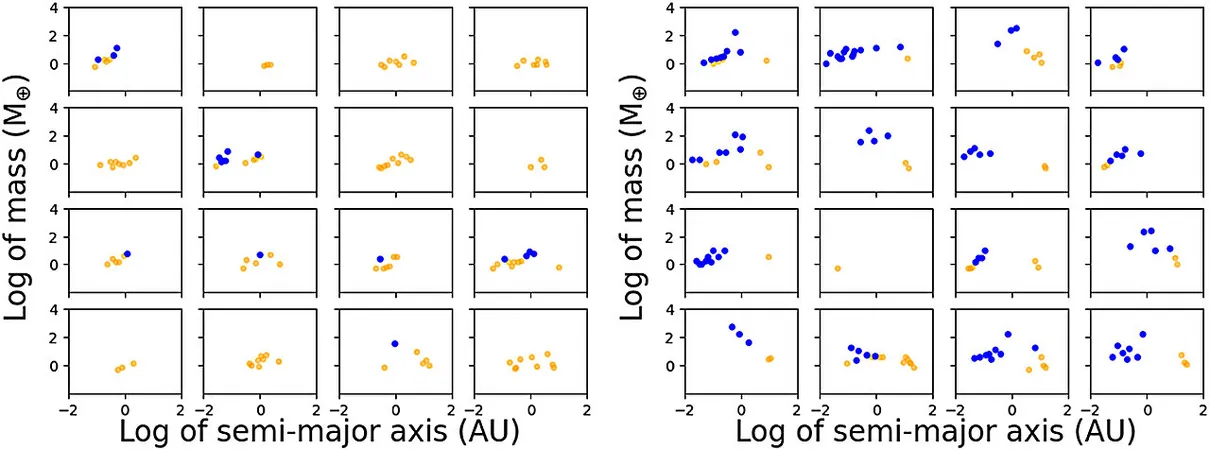
Revolutionizing the Hunt for Earth 2.0: How Machine Learning is Unleashing the Search for Habitable Planets
2025-04-09
Author: John Tan
A Game Changer in Planetary Research
A groundbreaking team from the University of Bern and the National Center of Competence in Research (NCCR) PlanetS is poised to reshape our understanding of the cosmos with a new machine-learning model designed to predict planetary systems that may host Earth-like planets. This innovation could accelerate the search for habitable worlds throughout the universe.
Why Exoplanets Matter—The Key to Finding Extraterrestrial Life
The quest for Earth-sized exoplanets—those orbiting stars beyond our sun—is a hot topic in modern planetary science. These planets are thought to be the most promising candidates for extraterrestrial life. Researchers at the University of Bern have unveiled a cutting-edge machine learning algorithm that identifies potential systems capable of nurturing Earth-like planets.
Meet the Brains Behind the Breakthrough
Leading the charge is Dr. Jeanne Davoult, a postdoctoral researcher at DLR in Berlin, who crafted the model during her doctoral research at the Physics Institute of the University of Bern. Alongside her are distinguished co-authors, including Prof. Dr. Yann Alibert, co-director of the Center for Space and Habitability, and Romain Eltschinger, a fellow Ph.D. student, both instrumental in bringing this research to life.
Inside the Algorithm: Training for Precision
The machine-learning model operates as a sophisticated statistical tool that learns to detect and categorize planetary systems potentially harboring Earth-like planets. "Our model relies on a custom algorithm I developed, trained to recognize the patterns that signify the presence of Earth-like planets within planetary systems," explains Dr. Davoult.
Harnessing the Power of the Bern Model
The algorithm utilizes data from the renowned Bern Model of Planet Formation and Evolution, a comprehensive framework established in 2003 that maps out the intricate processes behind planet formation. Dr. Alibert elaborates, "The Bern Model is unique in its capacity to interlink various physical processes, facilitating studies like this one.
Stunning Accuracy: 99% in Predicting Earth's Twin
The results are nothing short of astonishing. The machine-learning model boasts an accuracy rate of 99%, meaning that nearly all identified systems likely contain at least one Earth-like planet. This level of precision empowers astronomers to target specific systems more effectively.
A New Frontier in Discovering Habitable Planets
The model has already been tested against actual observed systems, leading to the identification of 44 candidates that could potentially harbor hidden Earth-like planets. An additional follow-up study has affirmed that these systems theoretically possess the right conditions for such planets.
Implications for Future Space Missions
Eltschinger, who contributed significantly to the model's refinement during his master’s thesis, notes that these findings are crucial for upcoming space missions like PLATO and LIFE, which aim to uncover and analyze small, cold planets. The model’s capabilities could dramatically reduce the duration of searches and increase discovery rates.
The Next Step in Our Cosmic Exploration
This revolutionary machine-learning approach marks a crucial advancement in the ongoing search for life-sustaining conditions beyond Earth. "We are taking significant strides towards uncovering planets that could support life and, by extension, exploring the possibility of life in the universe," concludes Dr. Alibert.