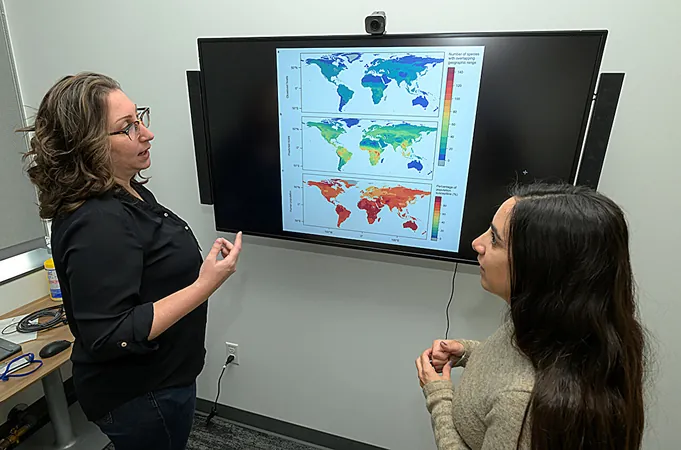
Revolutionizing Pandemic Prevention: AI Model Pinpoints Virus Reservoirs Hidden in Animal Kingdom!
2025-03-31
Author: Rajesh
Introduction
In a groundbreaking development, researchers at Washington State University have introduced a powerful machine learning model designed to predict which animal species are likely to harbor and spread viruses capable of infecting humans. This innovative tool could be a game-changer in our efforts to limit or even prevent future pandemics.
About the Model
The model, which specifically targets orthopoxviruses—including notorious threats like smallpox and mpox—analyzes both the genetic characteristics of viruses and the traits of potential host animals. By identifying species that could act as reservoirs and predicting geographical hotspots for potential outbreaks, this AI-driven approach offers a proactive strategy for managing zoonotic threats.
Key Findings
The study detailing this remarkable work has been published in the journal Communications Biology, revealing crucial insights that could reshape our understanding of virus emergence. "Nearly three-quarters of emerging viruses that infect humans come from animals," emphasized Stephanie Seifert, a leading viral emergence expert at WSU. "If we can better predict which species pose the greatest risk, we can undertake preventive measures before outbreaks escalate."
The analysis indicated Southeast Asia, equatorial Africa, and the Amazon region as high-risk zones for orthopoxvirus outbreaks. These areas not only harbor numerous potential hosts but also have concerningly low vaccination rates against smallpox—a crucial protective measure that ceased with the virus's eradication in 1980.
Animal Families of Concern
Furthermore, the study identified a variety of animal families likely to be involved in mpox transmission, such as rodents, felines, canids (dogs and relatives), and even raccoons. Notably, the model accurately ruled out rat populations, which have shown resistance to mpox in lab studies, showcasing its precision.
Improved Predictive Accuracy
Katie Tseng, a graduate student in veterinary medicine and the study's lead author, highlighted that the model’s predictive accuracy surpasses previous models. "While we initially targeted orthopoxviruses, the versatility of this tool means we could adapt it to forecast hosts for various other viral threats," she explained.
Pilar Fernandez, a disease ecologist involved in the project, pointed out a key innovation: traditional models relied heavily on ecological traits like habitat and diet. However, this new approach incorporates the genetic makeup of the viruses, leading to improved predictions. "Our model not only enhances the accuracy of host predictions but also paints a clearer picture of how viruses may spread across species," Fernandez stated.
Importance of Identifying Reservoirs
While orthopoxviruses typically lead to localized outbreaks, alarming occurrences such as the global spread of mpox in 2022 have raised concerns over the establishment of new endemic areas through novel animal reservoirs. Identifying these reservoirs is crucial for anticipating spillover events, but traditional methods of field sampling are often impractical and resource-heavy. The new model streamlines this process and can help target wildlife surveillance efforts effectively.
"When searching for the mpox virus reservoir in biodiversity hotspots like Central Africa, the task can feel overwhelming," Seifert acknowledged. "Leveraging machine learning models to prioritize our sampling efforts could significantly enhance our ability to trace these viruses back to their origins and assess the risks they pose to public health."
Conclusion
As this promising technology continues to evolve, the implications for global health are vast, heralding a new era of prevention strategies in the fight against pandemics. Stay tuned as we uncover more about the potential of AI in safeguarding our future!