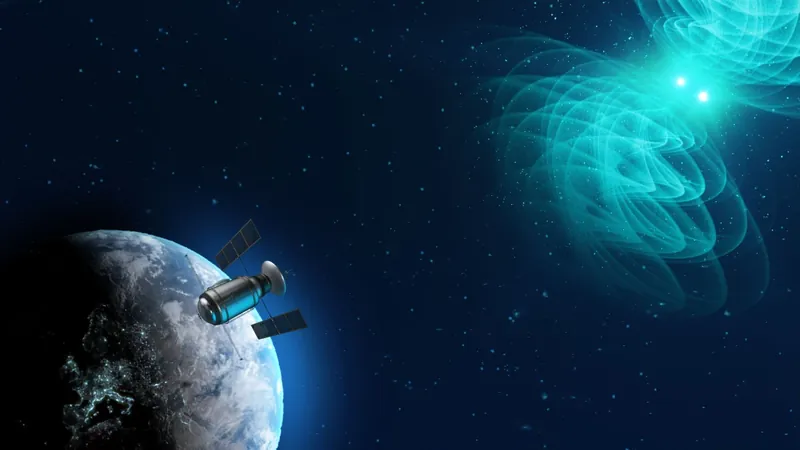
Revolutionizing Neutron Star Mergers Detection with AI: A Game Changer for Astronomers!
2025-03-31
Author: Sarah
Introduction
A groundbreaking artificial intelligence (AI) and machine learning (ML) approach is set to transform the way astronomers characterize binary neutron star (BNS) mergers, promising rapid and precise analysis based on the gravitational wave signatures produced during these cosmic events. Although this innovative method has yet to be tested on live merging events, it holds the potential to significantly speed up estimates regarding the mergers' locations and the masses of the neutron stars involved. This information could enable telescopes to quickly target and observe the accompanying electromagnetic signals from these significant astronomical phenomena.
Gravitational Waves and Observatories
Neutron stars and black holes generate ripples in spacetime, referred to as gravitational waves (GWs), during their mergers. Since the first detection of such waves in 2015, scientists have employed kilometer-scale interferometers, which measure the minuscule expansions and contractions of space-time caused by gravitational waves. These observatories are located in the U.S., Italy, and Japan, and are collectively known as the LVK observatories: the Laser Interferometer GW Observatory (LIGO), the Virgo GW Interferometer, and the Kamioka GW Detector (KAGRA).
The Importance of Early Detection
The merger of two neutron stars not only releases gravitational waves but also emits electromagnetic radiation. Even though both types of waves travel at the speed of light, the electromagnetic signals can be delayed due to complex processes occurring during the merger. As a result, the LVK observatories often detect the gravitational wave signal seconds or even minutes before the electromagnetic pulse arrives. Early and accurate detection of gravitational waves thus enhances the likelihood of capturing additional signals associated with these cosmic events.
Challenges in Signal Analysis
However, analyzing gravitational wave signals is challenging due to their intricate nature. The standard technique for interpreting these signals, Bayesian inference, is known to be sluggish. While there are faster alternatives available, many rely on algorithmic approximations that could compromise accuracy.
A Machine Learning Breakthrough
A team of physicists led by Maximilian Dax from the Max Planck Institute for Intelligent Systems in Tübingen, Germany, has made a significant breakthrough by developing a machine learning framework that can characterize and localize BNS mergers within just one second of a gravitational wave event, without relying on approximations. This was achieved by training a deep neural network model with millions of simulated gravitational wave events.
Implications of the Research
Once this neural network is fully trained, it can quickly process new gravitational wave data to predict crucial details of the merging neutron stars, including their masses, locations, and spins. Perhaps most importantly, this neural network provides a precise sky map, allowing astronomers to rapidly identify where the merger has occurred.
Focus on Electromagnetic Counterparts
Building on their previous work with binary black hole mergers, the research team emphasized the importance of quick inference for BNS mergers, particularly as these events produce electromagnetic counterparts, unlike black hole mergers. The results, published in *Nature*, aim to enhance the likelihood of observing these electromagnetic signals associated with BNS mergers and capture them closer to the actual merger time.
Future Directions
The implications of this cutting-edge approach are vast. Not only could it lead to more frequent observations of electromagnetic counterparts during neutron star mergers, but it could also unravel vital insights into the underlying processes at play during such cosmic collisions. Dax noted that this method might serve as a model for addressing the challenges posed by future gravitational wave detections, especially as the next generation of Gravitational Wave detectors, which are expected to produce longer signal durations, comes online.
Encouragingly, while the team has initially focused on data from existing detectors like LIGO and Virgo, they are poised to delve further into applying their machine learning strategies to next-generation gravitational wave detectors. This could open up new frontiers in our understanding of the universe's most violent events!
Conclusion
Stay tuned as astronomers prepare for a new era of discoveries, where AI paves the way for unprecedented insights into the cosmos!