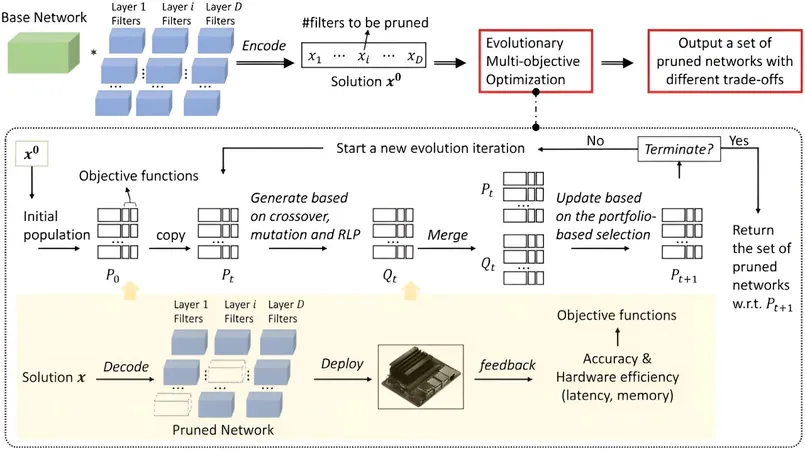
Revolutionizing Neural Networks: The Ultimate Pruning Technique for Tailored Hardware Optimization!
2024-09-23
Introduction
In the ever-evolving world of artificial intelligence (AI), deploying deep neural networks (DNNs) on devices with limited resources, like smartphones and IoT gadgets, is a pressing challenge. But a groundbreaking solution is set to change the game: hardware-aware neural network pruning.
What is Neural Network Pruning?
Neural network pruning is a critical technique that streamlines DNNs to make them fit better within the constraints of various hardware setups. Every platform—whether it's a powerful server or a compact mobile device—presents unique challenges in terms of hardware capabilities and resource availability. This diversity necessitates the development of pruned models that are finely tuned to individual hardware configurations.
The Innovative Approach from Shenzhen
According to a cutting-edge study released in the journal *Fundamental Research*, researchers from Shenzhen, China, have introduced an innovative approach to hardware-aware neural network pruning, utilizing multi-objective evolutionary optimization to optimize DNNs specifically for the hardware they will run on.
Insights from Researchers
Dr. Ke Tang, a senior researcher and the lead author of the study, elaborates: “We propose to employ Multi-Objective Evolutionary Algorithms (MOEAs) to effectively manage the neural network pruning challenge.” MOEAs stand out from traditional optimization methods by requiring no assumptions of differentiability or continuity, making them especially adept at black-box optimization—a crucial advantage when dealing with complex networks. Additionally, their ability to identify multiple Pareto-optimal solutions simultaneously allows users to customize their preferences effortlessly.
The HAMP Variant
However, the researchers discovered that while MOEAs unlock incredible potential, they often grapple with low search efficiency. In response, they developed a new variant called Hardware-Aware Multi-Objective Evolutionary Network Pruning (HAMP). “HAMP is a leading-edge memetic MOEA that integrates a sophisticated portfolio-based selection method with a surrogate-assisted local search operator. It stands out as the only method adept at managing direct hardware feedback while simultaneously ensuring accuracy,” says Wenjing Hong, the study's first author.
Validation through Experiments
The practical implications of HAMP were validated through rigorous experiments conducted on the mobile NVIDIA Jetson Nano. The findings indicated that HAMP not only surpasses existing state-of-the-art network pruning techniques but also effectively meets multiple objectives.
Conclusion and Future Implications
The results make it clear: HAMP delivers exceptional solution sets that encompass various trade-offs between latency, memory capacity, and overall accuracy, paving the way for fast deployment of neural networks in real-world applications.
As the world leans more heavily into AI, innovations like these are crucial. The potential implications span industries—from enhancing mobile apps to improving smart devices—by ensuring superior performance without sacrificing efficiency. This development signifies a monumental leap towards integrating robust AI capabilities into accessible technology!
Stay Tuned!
Stay tuned as we continue to follow innovations that are transforming the AI landscape and making powerful technology available to everyone!