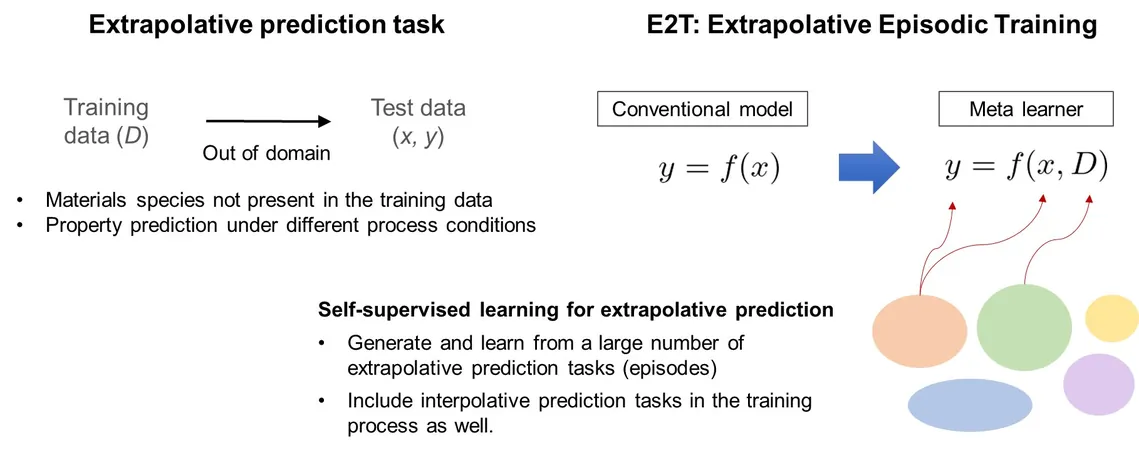
Revolutionizing Materials Science: AI Breakthrough in Predictive Learning
2025-04-16
Author: Daniel
A Game-Changer in Materials Research
A groundbreaking advancement in machine learning is set to revolutionize materials science, boasting the ability to predict material properties beyond traditional data limits. Spearheaded by a research group including Kohei Noda from JSR Corporation and Professor Ryo Yoshida at the Institute of Statistical Mathematics, this innovative approach is transforming how materials are discovered.
The Challenge: Limited Data and Predictive Power
At the heart of materials science lies the quest to uncover novel materials within unknown territories—places where no data currently exists. Unfortunately, conventional machine learning techniques typically excel at interpolating predictions, restricting them to regions close to the existing training data. This limitation is exacerbated in materials research due to the prohibitive costs of data collection.
Introducing E2T: The Extrapolative Solution
In response to these challenges, the research team introduced an innovative algorithm called E2T (Extrapolative Episodic Training). This approach trains a 'meta-learner' model on a wealth of artificially crafted extrapolative tasks derived from available data. The result? A model that autonomously learns to make extrapolative predictions.
Astounding Results in Material Property Predictions!
E2T has showcased remarkable effectiveness in predicting material properties, including those not present in the training set. The meta-learner's training allows it to quickly adapt to new domains with minimal additional data, drastically improving prediction accuracy.
Why This Matters: The Future of Material Discovery
Recent strides in machine learning have unleashed tremendous potential for discovering and developing new materials. The technology can explore vast candidate spaces to find materials with desirable properties. Nevertheless, the persistent issue of insufficient data limits the scope of machine learning applications. The quest for groundbreaking materials continues, necessitating a leap into the unknown.
Meticulous Methodologies Behind Extrapolation
The study examines various techniques within machine learning designed to enable extrapolation, such as domain generalization and data augmentation. Among these, the meta-learning framework introduced by E2T stands out for its capability to directly foster broadly applicable extrapolative learning methods.
Real-World Testing and Performance
In testing over 40 property prediction tasks encompassing both polymeric and inorganic materials, E2T consistently outperformed traditional machine learning models—especially in extrapolative accuracy. Despite showing impressive results, the performance could still be optimized further to achieve the so-called 'oracle' level, which signifies a model trained on comprehensive data.
The Road Ahead: Bridging the Unknowns
As materials science aims to explore uncharted territory, E2T’s findings raise an enthralling question: can models leverage their existing learning to adapt to entirely new environments? Early evidence suggests that E2T might hold universal applicability, opening doors not only in material science but also across various AI domains.
A Future Fueled by AI: Innovating Foundation Models
One of the most exciting implications of this research is the potential for E2T to enhance foundation models. These models, which are trained on extensive datasets, could gain significant adaptability and reduced data requirements, ultimately leading to high levels of predictive accuracy. The innovative extrapolative capabilities brought forth by E2T may lead to groundbreaking advancements across numerous scientific fields.