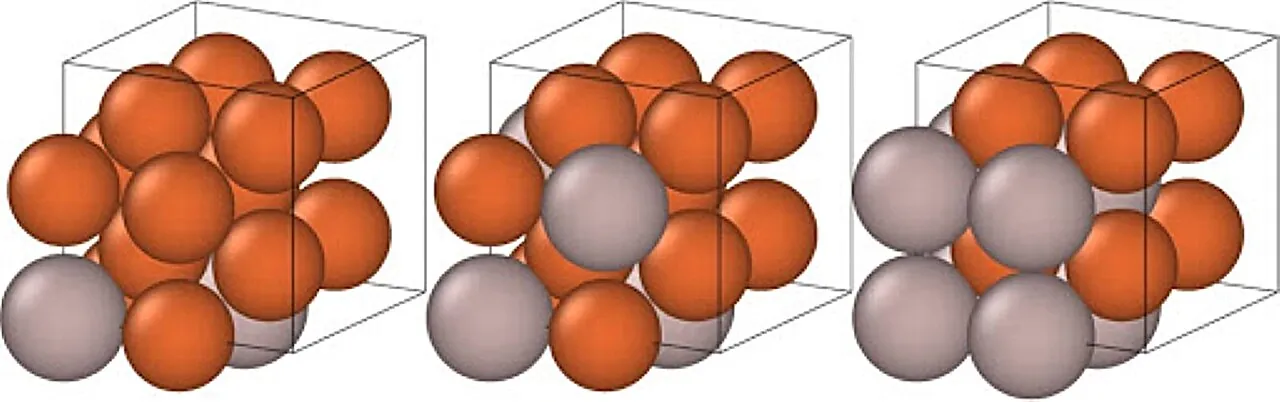
Revolutionizing Material Science: How Magnetic Force Training Enhances AI Simulations
2025-04-16
Author: Rajesh
Unlocking the Secrets of Magnetic Materials
Imagine a world where artificial intelligence can accurately simulate the behavior of magnetic materials crucial for next-gen electronics and advanced medical technologies. An international team of researchers has taken a groundbreaking step in this direction by developing a new method that leverages machine-learning interatomic potentials (MLIP) to predict magnetic material properties with unprecedented reliability.
The Game-Changing Approach
At the heart of this innovation is the training of AI models using magnetic forces—derivatives of energy concerning magnetic moments—to enhance the precision of simulations. Unlike traditional methods that depend heavily on costly and time-consuming experimental approaches, this AI-driven technique allows for faster research and prototyping.
Why Magnetic Materials Matter
From refrigerator magnets to cutting-edge medical imaging devices, magnetic materials are omnipresent. Mastering magnetism at an atomic level can unlock futuristic applications in fields like spintronics, which utilizes electron charge and spin, and targeted drug delivery using magnetic nanoparticles.
Overcoming Simulation Hurdles
Typically, simulating materials requires massive computational power, especially when using methods like Density Functional Theory (DFT) that examine thousands of atoms. However, MLIPs can drastically speed up this process, enabling scientists to model larger systems and extended processes.
The Power of Magnetic Force Training
The researchers tackled the limitations of standard MLIPs by integrating magnetic moments into their models, ultimately enhancing the learning process. By training these models on data derived from around 2,600 atomic configurations of an iron-aluminum alloy, they achieved remarkable accuracy.
Stunning Results and Future Possibilities
The results were striking: a tenfold reduction in prediction error for magnetic forces while keeping conventional force predictions stable. The new method also proved 100% reliable when optimizing atomic structures, making it a cornerstone for practical applications.
The Road Ahead
This research doesn't just open doors; it blazes trails for virtual screening and optimizing the compositions of new magnetic alloys and materials. Future applications could include understanding effects such as defects and grain boundaries in larger systems, which are vital for improving electric motors, transformers, and MRI technologies.
A Collaborative Effort
Experts from diverse institutions, including Skoltech and several international partners, contributed to this innovative study. As more researchers adopt this method, the landscape of materials science is set to evolve, making complex simulations more accessible and consistent.
Conclusion: A New Era in Material Simulations
The integration of magnetic force training into AI models not only enhances the reliability and accuracy of simulations but also paves the way for groundbreaking advancements across multiple scientific disciplines. The future of material science has arrived, and it's powered by AI.