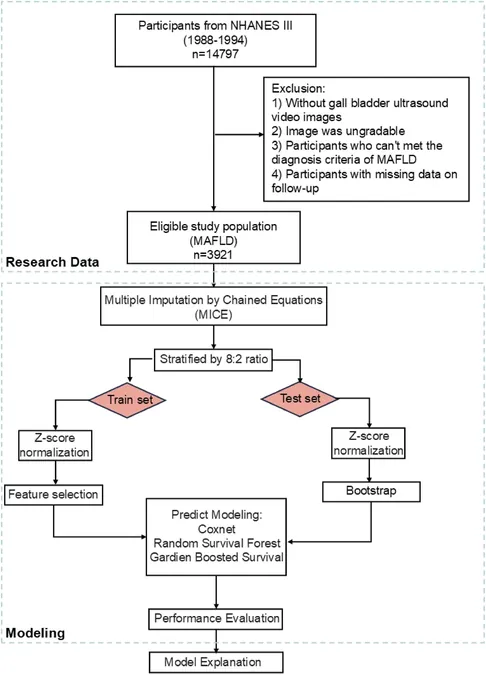
Revolutionizing MAFLD Mortality Predictions: The Power of Machine Learning
2025-05-15
Author: Jia
Rising Threat of Metabolic Dysfunction-Associated Fatty Liver Disease (MAFLD)
As the prevalence of Metabolic Dysfunction-Associated Fatty Liver Disease (MAFLD) soars, so does its mortality rate. This alarming trend underscores the urgent need for effective prediction models to identify high-risk individuals. While traditional methods have struggled, advanced machine learning algorithms are stepping into the limelight, promising enhanced predictive capabilities.
Harnessing Machine Learning for Accurate Predictions
A groundbreaking study tapped into data from the National Health and Nutrition Examination Survey (NHANES III), involving 3,921 MAFLD patients tracked over 26 years. Among these individuals, a striking 46.3% succumbed to various causes. Researchers deployed state-of-the-art machine learning models—including Coxnet, Random Survival Forest (RSF), and Gradient Boosted Survival (GBS)—to forecast all-cause mortality and sift through critical risk factors influencing these deaths.
Key Findings: Who's Most at Risk?
The standout Coxnet model excelled in both short and long-term forecasts, achieving a remarkable AUC of 0.82 at five years and an impressive 0.88 at 25 years. Critical risk factors identified included age, waist circumference, the FORNS score, alcohol consumption to liver ratio (AAR), and the Fatty Liver Index (FLI). Notably, smokers who enjoyed fewer than 100 cigarettes showed significantly improved survival rates compared to heavy smokers.
A Closer Look at MAFLD: Why Predict?
MAFLD, a leading cause of chronic liver disease, affects approximately 25% of the global population. This condition is often intertwined with metabolic issues like obesity and type 2 diabetes. Previous research revealed that MAFLD patients face a 17% higher risk of overall mortality compared to their non-MAFLD counterparts. With the mortality burden increasing, predictive models are crucial for timely interventions that can enhance patient care.
The Importance of Interpretable Machine Learning
Utilizing machine learning isn't just about predictions; it's also about understanding the 'why' behind these predictions. By leveraging the SHAP method, researchers illuminated the key features driving the model’s outputs, offering valuable insights that health practitioners can use to tailor interventions targeting modifiable risks.
Future Directions: A Path Towards Better Care
This study marks a pivotal step in utilizing machine learning to forecast mortality among MAFLD patients. However, while the Coxnet model shone in predictive power, the need for larger, diverse datasets is essential for broader application in real-world scenarios. The integration of specific mortality causes and innovative deep learning techniques could further refine these models, paving the way for even more accurate risk assessments in managing MAFLD.
Conclusion: A New Hope for MAFLD Management
As machine learning continues to advance, the potential to enhance MAFLD management grows exponentially. By identifying at-risk individuals and understanding the factors at play, healthcare professionals can initiate timely interventions, ultimately improving survival rates and quality of life for millions suffering from this increasingly prevalent disease.