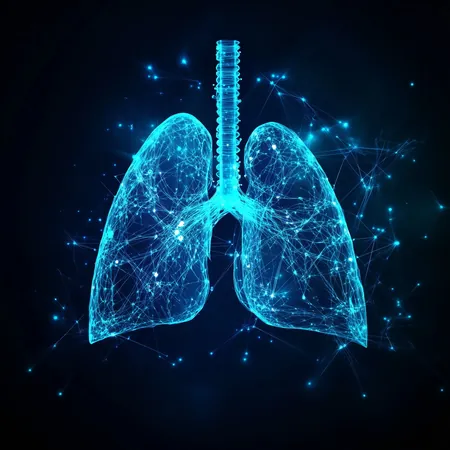
Revolutionizing Lung Cancer Detection: The Game-Changing Role of Quantum Machine Learning
2024-09-28
In a groundbreaking presentation at the 2024 Asia Pacific Conference on Innovation in Technology, researchers unveiled a study that leverages quantum machine learning (QML) to significantly enhance the accuracy of lung cancer detection. This innovative approach utilizes advanced QML models such as Pegasos QSVC and Variational Quantum Classifier (VQC), demonstrating unprecedented potential in an area where early detection is critical yet notoriously difficult.
The Urgent Need for Enhanced Detection Methods
Lung cancer, alarmingly one of the leading causes of cancer-related deaths globally, presents significant challenges regarding early diagnosis. Traditional diagnostic methods, including CT scans and biopsies, often fall short. They are not only costly and time-consuming but can also miss early-stage cancers, contributing to the disease's high mortality rates. With risk factors such as smoking and environmental pollutants compounding the issue, the medical community is in dire need of more efficient and scalable detection mechanisms.
Quantum Machine Learning: A New Frontier
The pioneering study focuses on using QML models to identify lung cancer risks more accurately and efficiently. Conventional machine learning struggles with high-dimensional data complexity, but QML offers the potential to tackle these challenges with superior computational efficiency. By combining Pegasos QSVC and VQC, the researchers aimed to push the boundaries of lung cancer prediction accuracy.
Among the findings, Pegasos QSVC eclipsed VQC with an impressive 85% classification accuracy. This model's success is attributed to its robust capacity for handling complex datasets and its intrinsic ability to avoid overfitting through regularization. Researchers meticulously transformed a diverse dataset from Kaggle, comprising 26 health attributes from over 1,000 patients, into a binary classification problem. They successfully categorized patients based on high-risk and not high-risk labels, optimizing the model’s predictive capabilities.
Peeking Under the Quantum Hood
Both models were scrupulously assessed through various metrics including accuracy, recall, precision, and F1 score. While VQC showcased potential, Pegasos QSVC thrived on the dataset's intricacy thanks to its stochastic gradient descent optimization, adaptable parameters, and resilience to noise. The improved regularization parameter further refined QSVC's performance, underscoring QML’s promise in the complex realm of healthcare and early cancer detection.
The Road Ahead: Challenges and Opportunities
Despite the excitement surrounding QML, the study notes significant hurdles ahead. Scalability remains a foremost concern; as datasets grow, current quantum computing hardware may struggle to keep up. The researchers advocate for further investigation into quantum error correction and enhancements in qubit stability to expand applications in healthcare.
As QML technology evolves, it holds the promise to transform predictive diagnostics, particularly for high-mortality diseases like lung cancer. The next crucial steps involve broadening the dataset and enhancing the quantum models to ensure generalized applicability in various healthcare contexts.
Conclusion: A Beacon of Hope for Lung Cancer Detection
The future of lung cancer detection could drastically change, thanks to the integration of quantum machine learning. If successfully implemented, these advancements may not only provide critical early warnings but also save countless lives in the process. As the healthcare landscape continues to shift towards innovation, the marriage of technology and medicine heralds exciting possibilities that were once thought to be the realm of science fiction. Stay tuned for what could be the next revolutionary breakthrough in healthcare technology!