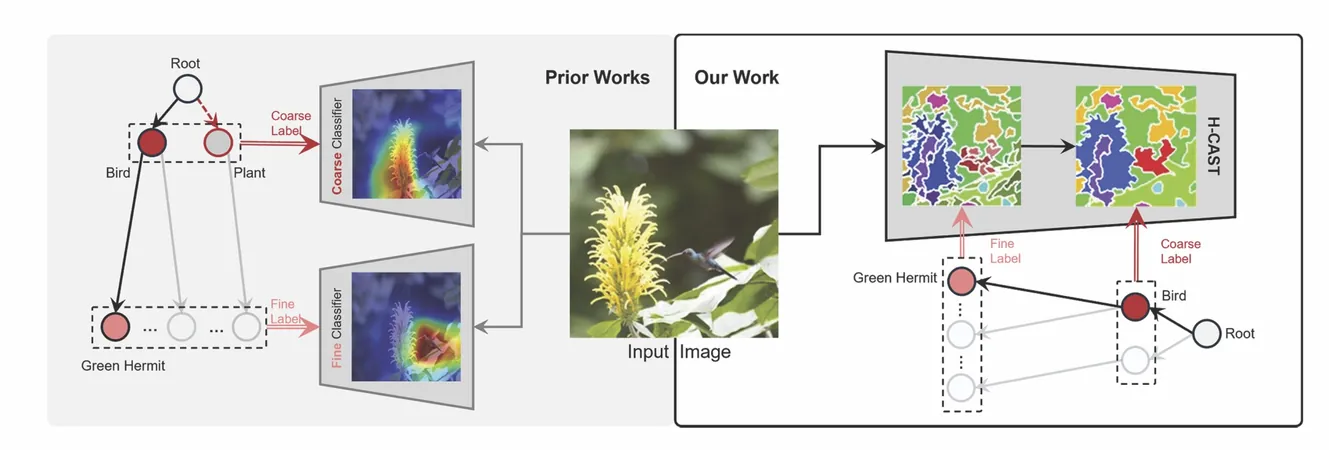
Revolutionizing Image Classification: Meet H-CAST, the Smart AI Model that Sees Hierarchically!
2025-05-14
Author: Siti
A groundbreaking AI model named H-CAST is transforming how we classify images by employing a hierarchical tree structure that ranges from broad to specific categories. Instead of getting lost in minute details, this model categorizes images progressively, such as classifying a bird into an eagle and further into a bald eagle.
Unveiled at the International Conference on Learning Representations in Singapore, H-CAST builds on the previous model CAST, advancing the field of visually grounded single-level classification. The research was also highlighted on the arXiv preprint server.
While many skeptics believe that deep learning can offer precise classifications, this method often falters when faced with imperfect images. Stella Yu, a computer science and engineering professor at the University of Michigan, explains, "Real-world applications encounter numerous flawed images. If a model zeroes in solely on fine details, it risks failing with images lacking enough clarity for that precision."
H-CAST's hierarchical approach offers a multi-tiered classification, tackling images at various levels of detail simultaneously. However, previous models struggled to maintain consistency when treating each level as a separate task. For example, while identifying a bird, a fine-level classifier may rely on specific traits like beak shape, while broader classifications depend on overall form. This often led to conflicting predictions: a model could suggest "green parakeet" while another might classify it as "plant."
What sets H-CAST apart is its ability to align fine to coarse predictions by employing intra-image segmentation, keeping all levels focused on the same object yet viewed through different lenses of detail.
Unlike past hierarchical models that trained from broad to specific semantic labels (like bird to hummingbird), H-CAST begins its recognition using fine visual details like beaks and wings that comprise coarser structures. This innovative strategy enhances clarity and accuracy in predictions.
Seulki Park, the lead author and postdoctoral researcher, emphasizes, "Most prior solutions have concentrated on semantics alone. Our findings show that consistent visual grounding across levels can vastly enhance performance. We aim to encourage a rethinking towards cohesive and interpretable recognition systems."
The research team employed unsupervised segmentation, a technique typically reserved for recognizing structures within larger images, to bolster hierarchical classification without needing pixel-level labels. This approach significantly improves segmentation quality.
To validate its performance, H-CAST was rigorously tested against four benchmark datasets, showcasing its superiority over existing hierarchical and baseline models. Stella Yu remarked, "Our model outperformed zero-shot CLIP and leading baseline models in hierarchical classification, yielding both higher accuracy and more reliable predictions." For example, in the BREEDS dataset, H-CAST achieved a remarkable 6% improvement in full-path accuracy compared to the previous top performer and an 11% advantage over baseline models.
Feature-level analysis further revealed H-CAST’s prowess in retrieving samples that maintain visual and semantic consistency across varying levels of the hierarchy—an area where earlier models frequently tripped over visually similar but semantically incorrect predictions.
The implications of H-CAST are vast, promising applications in scenarios requiring an understanding of images at multiple levels. It stands to greatly benefit wildlife monitoring for species identification and can assist autonomous vehicles in interpreting imperfect visuals, enabling safer and wiser decision-making even when details are obscured.
Park noted, "Humans naturally default to broader concepts; if I can't ascertain whether an image shows a Pembroke Corgi, I still know it’s a dog. However, models often stumble at that flexible reasoning. Our goal is to develop a system capable of adjusting its prediction level similar to human cognition."
H-CAST was developed and tested using ARC High Performance Computing resources at the University of Michigan, with contributions from UC Berkeley, MIT, and Scaled Foundations.