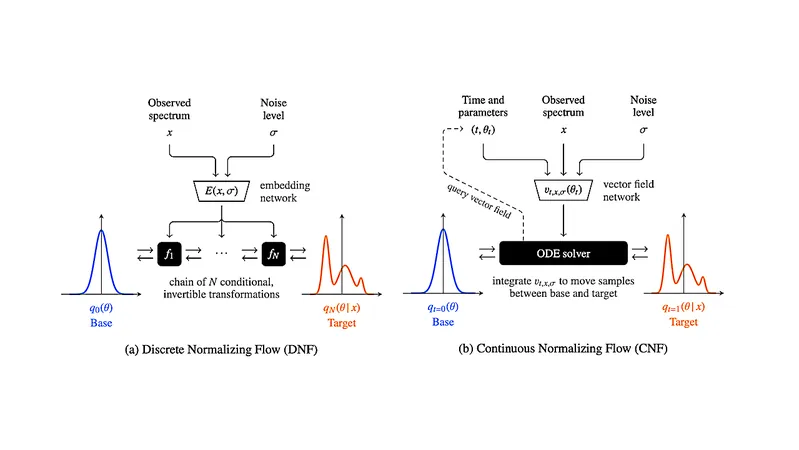
Revolutionizing Exoplanet Atmosphere Analysis: The Power of Flow Matching Technology!
2024-10-30
Author: Li
Introduction
Understanding the atmospheric properties of exoplanets is crucial for unraveling the mysteries of their formation, evolution, and potential for habitability. However, traditional Bayesian methods for atmospheric retrieval, such as nested sampling, often come with a hefty computational cost. In response to this challenge, a new wave of machine learning (ML) methodologies—including neural posterior estimation (NPE)—has gained traction.
Introducing Flow Matching Posterior Estimation (FMPE)
In our latest research, we introduce flow matching posterior estimation (FMPE), a cutting-edge ML approach aimed at enhancing the reliability and accuracy of atmospheric retrieval. Unlike its predecessors, FMPE offers significant architectural flexibility and scalability while retaining many advantages seen in NPE.
Importance Sampling: A Key Feature
A notable feature of our methodology involves employing importance sampling (IS). This technique not only verifies and rectifies ML outcomes but also computes the Bayesian evidence estimate, ensuring a higher level of confidence in the results. Moreover, by conditioning our ML models on specified noise levels of the spectral data, we enable adaptability to various noise models, accommodating a range of scenarios encountered during atmospheric analysis.
Performance Evaluation
When evaluated against simulated data, our noise level-conditional FMPE has shown to perform exceptionally well, matching the efficiency of traditional nested sampling across different noise levels. Remarkably, FMPE outpaces NPE by training approximately three times faster and achieving higher efficacy in IS application.
Practical Implications
The practical implications of importance sampling are profound. It aids in identifying inaccuracies in ML results and highlights model failures through low efficiency indicators. This, in turn, fosters accurate Bayesian evidence assessments, paving the way for reliable ML-driven approaches in exoplanetary science.
Future Directions
Furthermore, our advances in noise level conditioning serve an essential purpose for the future; they prepare the groundwork for designing next-generation instrumentation, ultimately expanding the range of signal-to-noise ratios that can be explored.
Conclusion
With our findings set for publication in "Astronomy & Astrophysics," this research is not just a leap forward in astrophysics but a significant stride towards truly understanding the atmospheres of distant worlds. Could this be the breakthrough that leads to discovering alien life? Stay tuned for the findings that could change everything we know about our universe!