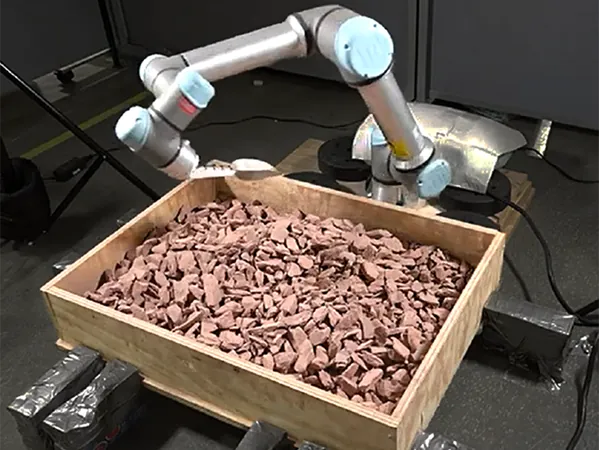
Revolutionizing Excavation: How AI-Powered Robots Are Set to Transform Complex Terrains
2024-10-28
Author: Arjun
Introduction
In an exciting breakthrough that could change the landscape of construction and excavation, a team of researchers from the University of Illinois Grainger College of Engineering, led by computer science professor Kris Hauser, is pioneering the use of artificial intelligence to enhance robotic excavation. Thanks to a substantial grant of $963,000 from the National Science Foundation (NSF), this initiative is focusing on how robots can effectively navigate and manipulate complex terrains, such as sand, soil, and rocks.
Harnessing AI and Machine Learning
The team is harnessing the power of machine learning techniques, particularly graph neural networks (GNNs), to create a new paradigm in how robots 'sense' their environments. This advanced technology may enable robots to combine tactile feedback with visual data, allowing for a more nuanced understanding of their surroundings.
Expanding Applications of GNNs
While GNNs have previously been used mostly for predicting the behavior of small objects in limited environments, Hauser and his team aim to broaden this application to address the challenges presented by larger, more unpredictable terrains. "We thought, why not try to predict behavior in large, deformable environments?" says Hauser. "We’re developing a learning-based simulator that models how the Earth behaves when a robot scoops, dumps, or smooths the ground."
Collaborative Research Effort
The research doesn't stop there; it’s being conducted in collaboration with Yunzhu Li from Columbia University. Together, they are exploring innovative approaches to understanding robots' interactions with these challenging surfaces. The focus is on enhancing robot efficiency in essential activities such as scooping and grading.
Key Goals and Adaptability
A key goal is to ensure that GNN models can adapt to a wide range of tasks, from massive excavation to detailed grading across various materials. Hauser elaborates, "If a robot encounters unexpected resistance while digging, it should be able to recognize the material—perhaps compacted soil mixed with rocks—and modify its strategy in real-time. This adaptability is crucial for work in environments where conditions can change rapidly."
Hardware Development
In terms of hardware, the team is developing a 3- to 4-foot robotic excavator equipped with a robust GPU to manage the hefty computational demands of these operations. Hauser expresses enthusiasm about the possibility of miniaturizing the technology, including plans for an experimental remote-controlled excavator that could deliver powerful computing capabilities without needing constant internet access.
Open-Source Initiative
One of the most progressive aspects of this research is the intention to release open-source software that can model and manipulate granular materials. This initiative aims to democratize access to groundbreaking research tools, enabling hobbyists and professionals alike to engage with the cutting-edge technology.
Implications for the Future
The implications of this research are significant. By embedding advanced computing capabilities directly into robotic systems, these machines are poised to undertake extensive construction and excavation tasks with minimal human intervention, particularly in remote areas where connectivity is problematic. As this project unfolds, it promises to redefine the future of excavation and construction, making it smarter, more efficient, and accessible to a broad audience.
Conclusion
Stay tuned, as the age of AI-driven excavation is just beginning!