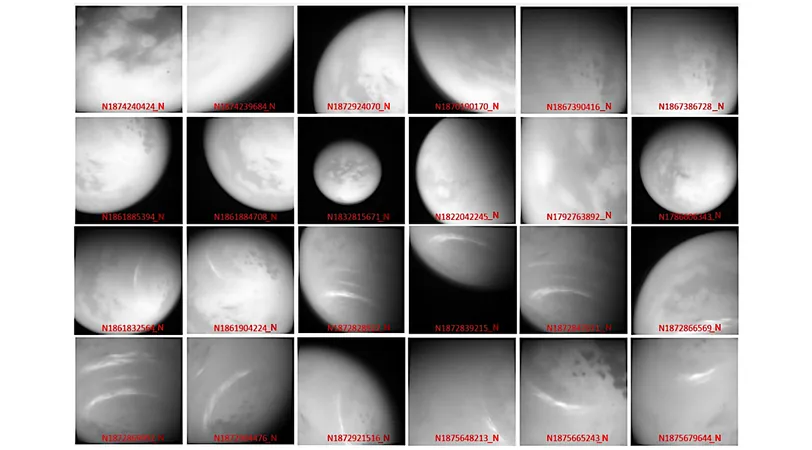
Revolutionizing Cloud Mapping on Titan: A Breakthrough with Artificial Intelligence
2025-01-09
Author: Li
Introduction
Recently, the field of planetary science has witnessed a groundbreaking advancement that merges deep learning with the analysis of extraterrestrial phenomena. While deep learning models have become commonplace in various computer vision tasks, their application in planetary science has been relatively limited. This is particularly the case when studying Titan, Saturn's largest moon, which is renowned for its thick atmosphere and complex weather systems.
Importance of Understanding Titan's Cloud Patterns
Understanding Titan's cloud patterns is crucial, as it offers insights into one of the most intricate climates in our Solar System. Unfortunately, much of the available imagery from missions like Cassini is still analyzed using traditional methods, which can be excessively labor-intensive.
Innovative Research Utilizing Mask R-CNN
In a recent innovative study, researchers have implemented a Mask R-CNN model—an advanced deep learning framework trained through transfer learning—to accomplish instance segmentation of Titan's clouds. This cutting-edge approach represents a significant leap in addressing big data challenges in planetary science.
Results of the Automated Cloud Mapping Process
The researchers demonstrated that automating the cloud mapping process provides quantitative metrics such as area and centroid calculations, which would be far too time-consuming for human analysts to produce. Remarkably, despite facing Titan's unique challenges, their automated method achieved accuracy levels that are on par with contemporary cloud identification studies conducted on Earth and other celestial bodies.
Comparison Between Human and Algorithm-Driven Approaches
The study also contrasts the efficiency of human labor with algorithm-driven techniques, highlighting the impressive speed offered by transfer learning. This advancement may well unlock new opportunities for data exploration on Titan, facilitating a more robust understanding of its meteorological phenomena.
Broader Implications for Planetary Science
Furthermore, the implications of this technology are vast, suggesting that similar methodologies could significantly enhance the analysis of image data in other areas of planetary science, where machine learning techniques have not yet reached their full potential.
Future Perspectives
Looking ahead, upcoming missions targeting not just Titan but the entire Solar System, as well as new remote sensing initiatives aimed at Earth, are set to generate an overwhelming amount of image data. Leveraging machine learning for analysis will be essential to manage and interpret this deluge of information effectively, ensuring that we continue to uncover the mysteries of our neighboring worlds.
Conclusion
This remarkable achievement not only paves the way for future discoveries about Titan but also sets a precedent for integrating advanced technologies into planetary research, ultimately transforming our approach to understanding the cosmos.