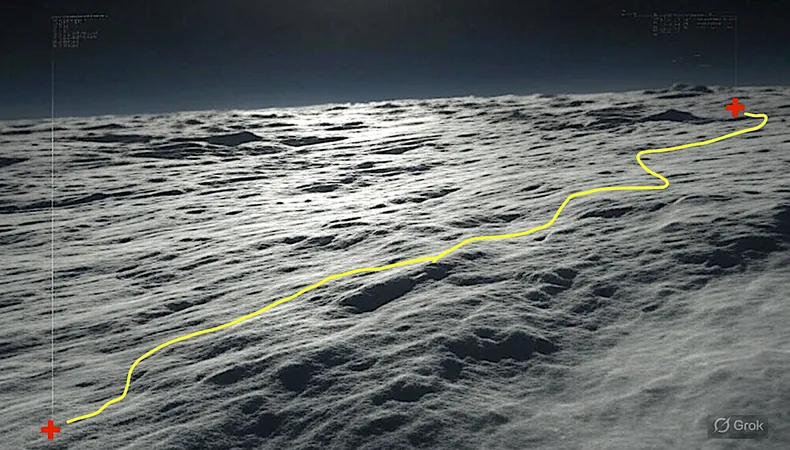
Revolutionizing Astrobiology: The Rise of Machine Learning and AI for Space Exploration
2025-04-03
Author: John Tan
In the ever-evolving field of space exploration, artificial intelligence (AI) and machine learning (ML) have emerged as game-changers, particularly in the field of astrobiology. These advanced technologies are enabling unprecedented levels of data processing, rapid analysis, and insightful pattern recognition that enhance our understanding of life beyond Earth.
Astrobiology, which investigates the potential for life in the universe, presents unique challenges. Models in this field must adeptly differentiate complex biotic signals from the intricate and often noisy abiotic backgrounds found on distant planetary bodies. The urgency for such capabilities is underscored by the rapid increase in data generated by advanced space instruments, making AI and ML indispensable tools for not just data analysis but also autonomous decision-making in space missions.
Why Science Autonomy Matters
The motivation behind enhancing scientific autonomy through AI and ML lies in the sheer volume of data produced by modern space missions. AI technologies facilitate the processing of vast datasets at a speed that surpasses human capabilities, allowing scientists to glean meaningful insights in real-time. This is especially vital in astrobiology, where finding evidence of life amidst complex datasets is a formidable task.
For instance, the innovative techniques used in astrophysics and Earth observation—such as crater identification—can be adapted to identify biological markers on other planets and moons. This adaptability is crucial as humanity sets its sights on increasingly distant celestial bodies, such as Titan and Europa, where missions like NASA’s Dragonfly and the Europa Clipper are poised to unfold.
Overcoming Communication Limitations
One of the significant hurdles in deep-space missions is the communication latency caused by the vast distances from Earth. This latency can hamper timely decision-making and scientific discovery. As spacecraft travel further from our planet, they encounter fundamental communication limits and stringent mission design challenges, such as low bandwidth and limited power and storage resources.
To remedy these issues, substantial investments in infrastructure are required. Upgrading facilities like NASA’s Deep Space Network will help improve data return rates, but these enhancements alone are not sufficient. Here, the integration of onboard AI and ML technologies plays a crucial role. These tools allow spacecraft to analyze data, prioritize scientific objectives, and make autonomous decisions based on real-time data without waiting for Earth’s directions.
A Vision for the Future
The long-term vision for space missions is to achieve comprehensive in situ analysis. This means equipping spacecraft with the ability to conduct real-time analyses, make autonomous decisions, and respond dynamically to new findings in the field. Communication between autonomous agents and Earth will be critical for ensuring accountability and learning from these decisions.
As missions increase in complexity, so too must our machine learning techniques evolve. Leveraging AI can significantly enhance mission efficiency not just by optimizing data transmission but also by enabling opportunistic science, anomaly detection, and resource optimization. These advancements will be pivotal for the success of future missions aimed at unlocking the mysteries of life beyond our planet.
In conclusion, the integration of AI and ML into astrobiology represents a frontier that promises to enhance not only how we process and analyze data from the cosmos but also our overall approaches to exploration. As we prepare for the next wave of missions, embracing these technologies will be key to unraveling the mysteries of life in the universe.
Stay tuned as we delve into how these autonomous systems can reshape our understanding of astrobiology and drive the next generation of space exploration!