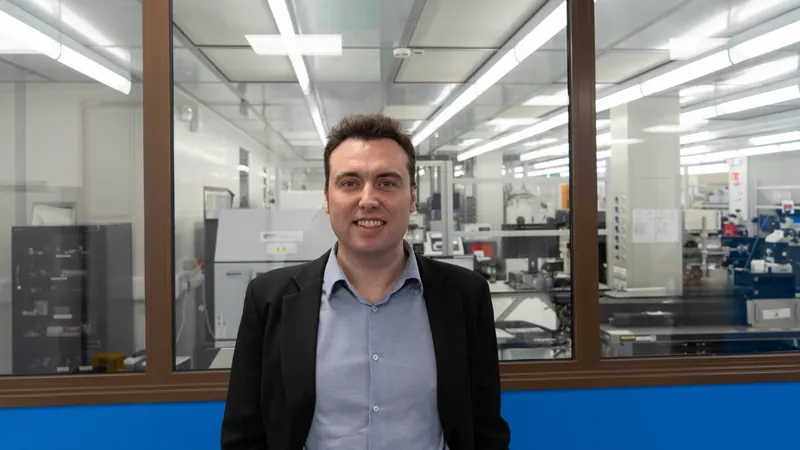
Revolutionizing AI with Neuromorphic Computing: Silicon Transistors Mimic the Brain!
2025-03-28
Author: Sarah
In a groundbreaking study, researchers from the National University of Singapore (NUS) have unveiled an astonishing discovery: a standard silicon transistor, the core component of microchips found in everything from computers to smartphones, can operate similarly to a biological neuron and synapse under certain conditions. This pioneering work, spearheaded by Associate Professor Mario Lanza from the Department of Materials Science and Engineering at NUS, represents a significant leap towards more efficient and scalable hardware for artificial neural networks (ANNs). Their findings were published in the prestigious journal *Nature* on March 26, 2025.
Bridging the Gap Between Silicon and Biological Intelligence
The human brain, composed of nearly 90 billion neurons interconnected by approximately 100 trillion synapses, enjoys remarkable energy efficiency—outpacing even the most advanced electronic processors. Studies highlight how these connections enable learning and memory through a phenomenon known as synaptic plasticity. For decades, engineers and scientists have sought to bring this efficiency to electronic processing through artificial neural networks. However, while these software-based ANNs have sparked a revolution in artificial intelligence, notably in applications like chatbots and image recognition, they typically require substantial computational power and energy, making them less practical for widespread applications.
Neuromorphic computing aims to harness the brain's efficiency by redesigning computing architectures to facilitate memory and computation in tandem—an approach known as in-memory computing (IMC). To truly mimic brain-like processing using silicon technology, researchers have faced hurdles due to the requirement for complex multi-transistor circuits or unverified new materials for manufacturing.
NS-RAM: A New Era in Electronics
According to Professor Lanza, “For true neuromorphic computing, we need hardware that combines scalability and energy efficiency.” The NUS team achieved a major milestone by demonstrating that just a single silicon transistor could replicate both neural firing and changes in synaptic weight—the essential actions of biological systems. This remarkable feat was accomplished by meticulously adjusting the transistor's bulk terminal resistance, leading to two critical phenomena: punch-through impact ionization and charge trapping. They also created a novel two-transistor cell that can operate in either neuron or synaptic modes, branded as "Neuro-Synaptic Random Access Memory" (NS-RAM).
Lanza highlighted the advantage of their approach, stating, “Unlike other solutions that depend on intricate transistor arrays or unproven materials, our method utilizes commercial CMOS (complementary metal-oxide-semiconductor) technology.” This platform, which is foundational to modern computer processors and memory chips, ensures that the technology is not only reliable but also scalable and compatible with existing semiconductor fabrication processes.
A Game Changer for AI Hardware
The NS-RAM cell has demonstrated impressive attributes during experimental tests, including low power consumption, consistent performance over numerous operational cycles, and predictable behavior across various devices. These characteristics are essential for constructing reliable ANN hardware suitable for real-world applications. This breakthrough is a significant stride toward developing compact, power-efficient AI processors capable of enabling quicker and more responsive computing—moving us closer to a future where technology emulates the remarkable capabilities of the human brain.
As we stand on the precipice of this neural revolution, the implications for artificial intelligence are vast, with potential applications ranging from more responsive consumer electronics to sophisticated machine learning systems that can learn and adapt like humans. The future of computing may soon be smarter and more efficient than we ever imagined!