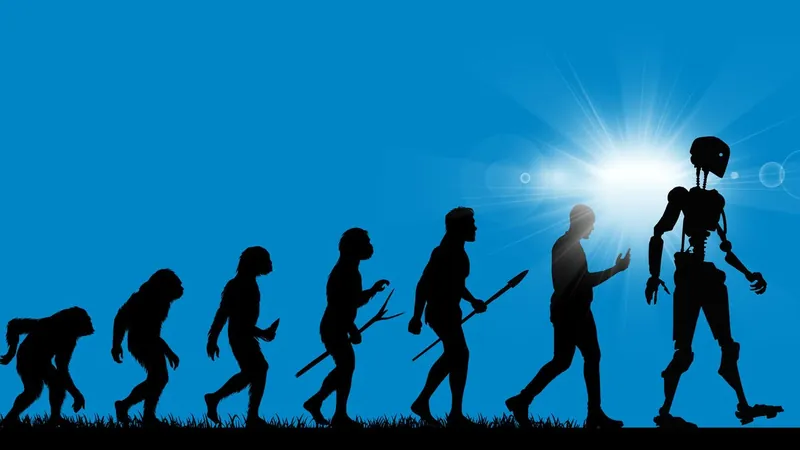
Revolutionizing AI: Google DeepMind's 'Mind Evolution' Technique Could Redefine Intelligent Problem Solving!
2025-01-24
Author: John Tan
Introduction
In a groundbreaking revelation, Google DeepMind is shifting the paradigm of artificial intelligence with an innovative technique termed "mind evolution." This approach aims to enhance the capabilities of AI systems, particularly in complex, practical tasks that traditional methods struggle to address.
The Rise of Inference in AI
One of the most significant trends throughout the past year in AI has been the refinement of inference—essentially, how AIs make predictions. Recent improvements, particularly with large language models (LLMs), have highlighted the effectiveness of techniques like "chain-of-thought," where the model communicates its reasoning step-by-step. This has previously fueled notable enhancements in benchmark tests, propelling models like OpenAI's GPT-3 to impressive scores on challenging assessments such as the ARC-AGI test.
Challenges Faced by Leading AI Models
However, the reality is that even the leading AI models, including Google DeepMind's Gemini and OpenAI's GPT-1, often falter in practical scenarios. A case in point: when tasked with creating a travel itinerary on the TravelPlanner benchmark, these advanced models have remarkably low success rates—just 5.6% for one and 11.7% for the other!
The Mind Evolution Technique
In response to these lackluster results, Kuang-Huei Lee and his fellow researchers at Google DeepMind have proposed a transformative method, "mind evolution," which goes beyond the conventional chain-of-thought approach. This new technique mimics natural selection, utilizing a genetically inspired algorithm that encourages LLMs like Gemini 1.5 Flash to generate a range of potential solutions. These solutions are subsequently evaluated based on their fitness to address the posed query.
How Mind Evolution Works
Natural evolution iteratively weaves progress through trial and error—more fit organisms thrive while less effective ones fall by the wayside. In parallel, mind evolution conditions the AI to critically assess and improve its responses through a parallel dialogue emulating a conversation between an "author" character generating ideas and a "critic" character refining those ideas based on feedback.
Breakthrough Results
The breakthrough results are staggering. When employing this mind evolution technique, the Gemini model achieved an astounding 95.2% success rate on TravelPlanner, soaring above the typical rates observed previously. Even more astonishing, the more powerful Gemini Pro model reached an exceptional 99.9% accuracy!
Computational Intensity of Mind Evolution
However, there is a caveat: mind evolution is computationally intense, requiring significantly more resources than the standard Gemini approach. The Flash variant utilizes 167 API calls for evaluation compared to just one for the standard functions, and it consumes millions of tokens due to the intricate prompts involved. Despite this, mind evolution proves to be more efficient than many other search strategies, steadily improving its effectiveness with each output evaluation.
StegPoet Benchmark and Performance
In a fascinating twist, Lee's team introduced an original benchmark called StegPoet, challenging Gemini's competency in steganography—the art of concealing messages within text. Results show that even with mind evolution, performance on this task was only 43.3% for Gemini Flash and 79% for Gemini Pro, though these numbers are significantly better than random chance.
Conclusion and Future Prospects
Ultimately, the findings from Google DeepMind's exploration into mind evolution expose an expansive frontier within AI inference, showcasing new methodologies that transcend simple prompt crafting. As the AI community watches with bated breath, the challenge remains: how to finetune these resource-intensive strategies without compromising the quality of output.
Stay tuned as advancements in AI technology continue to unveil staggering possibilities for human-machine collaboration!