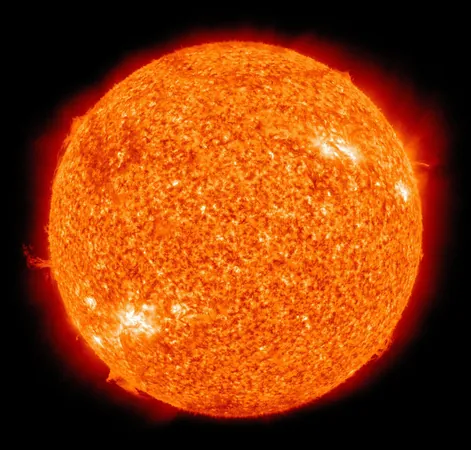
Revolutionary Neural Network Boosts Data Compression for Groundbreaking Solar Telescope!
2025-03-27
Author: Daniel
Introduction
In a remarkable development, a team of researchers from the Yunnan Observatories of the Chinese Academy of Sciences and Southwest Forestry University has unveiled an innovative neural network methodology designed to significantly improve the efficiency of spectral data compression from the New Vacuum Solar Telescope (NVST). This pioneering research, published in the esteemed journal Solar Physics, addresses the growing challenges experienced in the storage and transmission of high-resolution solar observation data.
Challenges in Data Management
The NVST, which plays a critical role in solar research, generates enormous volumes of spectral data, creating substantial burdens for data storage and transmission. Conventional compression methods, like principal component analysis (PCA), typically offer modest compression ratios of around 30, but they often introduce distortions that can compromise the integrity of reconstructed data. Such challenges have limited the applicability of these traditional techniques in high-stakes scientific environments.
Innovative Solution: Convolutional Variational Autoencoder (VAE)
To tackle these obstacles, the researchers have turned to advanced deep learning strategies, specifically utilizing a Convolutional Variational Autoencoder (VAE) for compressing Ca II (8542 Å) spectral data. This cutting-edge approach has achieved stunning compression ratios of up to 107 without sacrificing data quality. The decompressed data remains so faithful to the original observations that errors fall within the inherent noise levels, maintaining scientific reliability crucial for solar observations. Notably, errors in Doppler velocity measurements are kept below 5 km/s, a threshold that is vital for accurate analysis in solar physics.
Impact and Future Prospects
This breakthrough not only enhances the efficiency of data transmission and sharing from the NVST but also opens doors for greater collaboration among scientists, enabling them to share and analyze solar data more effectively. Moreover, the researchers believe this scalable solution can be adopted by other solar observatories that are grappling with similar data management issues, allowing for advancements in solar research on a global scale.
Conclusion
As excitement builds around this innovative approach, the potential implications for solar physics and related fields could be profound—ushering in a new era of solar observation that promises to deepen our understanding of solar phenomena and their impacts on space weather and beyond!