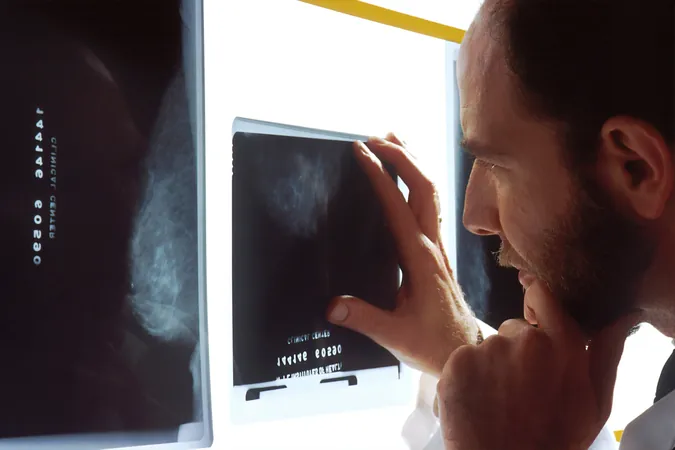
Revolutionary Method to Boost Radiologists’ Diagnostic Accuracy Unveiled!
2025-04-03
Author: Mei
Introduction
Medical imaging has always posed a challenge when it comes to precise interpretation, especially with common tools like X-rays. Radiologists frequently resort to terms such as "may" or "likely" to communicate the likelihood of conditions like pneumonia accurately. But here’s the shocking revelation: a recent study indicates that these descriptive phrases can often mislead — with radiologists sometimes being overconfident in their assessments.
Groundbreaking Research
Published in the arXiv preprint server, this groundbreaking research by a collaborative team of MIT researchers along with experts from hospitals affiliated with Harvard Medical School introduces a new framework designed to quantify and improve the reliability of radiologists' diagnostic reports. This method aims to ensure the words chosen by radiologists legitimately reflect the accuracy of their findings.
Issues with Confidence Levels
One of the key aspects the study highlighted is the tendency of radiologists to miscalculate their confidence levels. For instance, when a radiologist claims that a situation is "very likely," they often fall into the trap of overconfidence. On the flip side, using phrases like "possibly" can indicate unwarranted uncertainty. “The influence of the words radiologists use is paramount,” emphasizes Peiqi Wang, an MIT graduate student and lead author of the paper. “Enhancing the reliability of these reports ultimately benefits patient care.”
Innovative Methodology
The team utilized clinical data to develop an innovative methodology to assess how effectively radiologists convey certain levels of certainty through language. An example provided in the study illustrates this well: if a radiologist describes a chest X-ray as showing “possible pneumonia,” the referring physician might choose to conduct further imaging to confirm the diagnosis. However, if the report states “likely pneumonia,” immediate treatment may be initiated, potentially affecting patient outcomes.
Calibration and Probability Distributions
So how does this method work? Traditionally, calibration metrics rely on straightforward confidence scoring, similar to how weather apps predict rain likelihood. If a weather app forecasts an 83% chance of rain, it is considered well-calibrated if it rains 83% of the time under those circumstances. Wang points out that human language, however, escapes such rigid numerical representation.
The research team instead adapted their approach to treat ambiguous certainty phrases as probability distributions. This means a phrase like "consistent with" may indicate a high probability of pathology presence, while "may represent" reflects a broader, uncertain range of potential outcomes.
Findings and Recommendations
The researchers further sketched out probability distributions based on surveys conducted with radiologists, revealing the layered meanings behind each term. Phrases were meticulously ranked, and certainly, their framework provided specific suggestions to enhance diagnostic reports, helping radiologists modify their language for accuracy.
In practical terms, the study revealed that radiologists generally exhibited a tendency to be underconfident when diagnosing common issues like atelectasis but overconfident when confronted with more subjective conditions like infections. By applying their calibration framework, the researchers believe that even slight modifications to the language radiologists use could drastically improve diagnosis accuracy.
Future Directions
Looking ahead, Roy Wang and his team plan to push the boundaries of this research further, aiming to incorporate a broader array of clinical data. “By expanding this framework, we hope to refine and revolutionize diagnostic practices not only for X-rays but also for abdominal CT scans and beyond.”
Conclusion
As the medical field continues to evolve, tools that enhance decision-making accuracy could lead to remarkable improvements in patient care — a development that everyone in healthcare should eagerly anticipate!