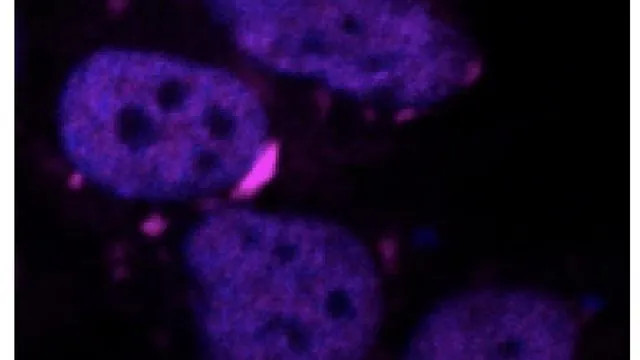
Revolutionary Machine Learning Tool Predicts Protein Behavior Linked to Neurodegenerative Diseases
2025-04-02
Author: Arjun
A groundbreaking research group, led by Gian Gaetano Tartaglia at the Italian Institute of Technology (IIT), has developed a cutting-edge machine-learning algorithm that aims to revolutionize our understanding of how proteins contribute to neurodegenerative diseases like Amyotrophic Lateral Sclerosis (ALS), Parkinson's, and Alzheimer's. The algorithm, named catGRANULE 2.0 ROBOT, offers a unique approach to identifying molecular targets vital for future research and therapies, as detailed in a recent article published in the journal Genome Biology.
Neurodegenerative diseases represent a pressing health issue globally, with Italy alone seeing approximately 1 million affected individuals. Each patient incurs an average healthcare cost of about seventy thousand euros, underscoring the urgent need for effective intervention strategies. The research conducted by Tartaglia's team delves into the complex chemical-physical behavior of specific proteins associated with these debilitating conditions.
In healthy cells, certain proteins can form biomolecular condensates—dense clusters that can support cellular functions and stress responses. However, when the condensation process is disrupted, these proteins can aggregate into harmful structures that eventually lead to cell death. Notable examples include Lewy bodies found in Parkinson's disease, filamentous accumulations in motor neurons related to ALS, and amyloid plaques indicative of Alzheimer's.
The transition from health to disease often hinges on alterations in protein structure, which can convert functional biomolecular condensates into toxic aggregates. With the insights of post-docs Michele Monti and Jonathan Fiorentino, the catGRANULE 2.0 ROBOT algorithm was designed to explore the relationship between protein mutations and the behavior of these condensates. Its pioneering machine-learning technology offers researchers a way to trace harmful proteins, thereby laying the groundwork for targeted research and therapeutic development.
As Tartaglia explains, 'Identifying biochemical signals associated with neurodegenerative diseases is essential for timely interventions that could mitigate cognitive decline.' The system they have trained detects the formation of condensates—a precursor to toxic aggregate formation—while acknowledging the significant role of protein-RNA interactions in this process.
The mechanism behind the formation of these biomolecular condensates hinges on liquid-liquid phase separation, a phenomenon where certain proteins possess a three-dimensional structure conducive to clumping. RNA molecules, in turn, play a regulatory role in either promoting or inhibiting this clumping behavior through their interactions with proteins.
The research team is focused on understanding RNA-protein interactions, training catGRANULE 2.0 ROBOT to utilize this data to predict scenarios where biomolecular condensates might form. By analyzing protein structures and their amino acid sequences along with RNA affinities, researchers can assess the likelihood of generating toxic aggregates as a result of phase separation. Notably, any mutations altering protein-RNA interactions could have serious pathological consequences by affecting condensate formation.
This innovative study is part of the IVBM-4PAP project, spearheaded by IIT, aimed at creating the In-Vivo Brillouin Microscope (IVBM). This groundbreaking tool will allow researchers to observe proteins and condensates in living cells without external interference, providing critical insights that could lead to new therapeutic targets for neurodegenerative diseases. The catGRANULE 2.0 ROBOT serves as a theoretical foundation for this initiative, generating actionable predictions about which proteins and mutations are of particular relevance.
By marrying the computational prowess of this algorithm with hands-on experiments using the upcoming IVBM, researchers now possess a potent asset for identifying early pathological signals and devising novel therapeutic approaches to curb the progression of neurodegenerative diseases. This integrated strategy holds promise for significantly reducing the long-term impacts of these conditions on individuals and society at large.
As advancements in methodologies continue, the hope for more effective treatments and proactive responses to neurodegenerative diseases grows ever closer. Stay tuned for further developments that could one day change lives.