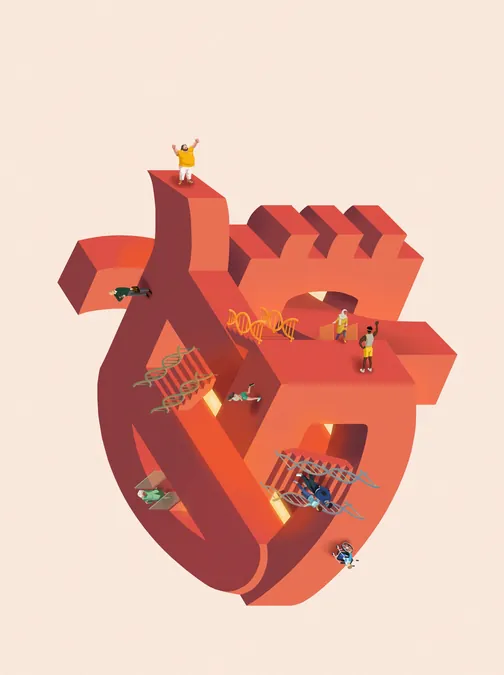
Revolutionary Machine Learning Model Transforms Risk Assessment for Heart Disease
2025-04-17
Author: Siti
A Game-Changer in Heart Disease Detection
Coronary artery disease (CAD) reigns as the leading cause of death in the United States. Alarmingly, effective preventive treatments are underutilized, often because individuals remain unaware of their CAD risks until it's too late.
Scripps Research Unveils Groundbreaking Predictive Model
Enter the scientists at the Scripps Research Translational Institute, who have harnessed the power of machine learning to craft a pioneering model that outshines traditional risk assessments, which heavily rely on age alone. Published in *Nature Medicine* on April 16, 2025, their revolutionary model incorporates genetics, lifestyle choices, and medical history—making risk predictions personal and precise.
Why Personalization is Key to Prevention
Dr. Ali Torkamani, a leading researcher in the study, believes that tailored risk assessments could inspire proactive participation in preventative measures. "By first predicting the likelihood of developing CAD, our model empowers healthcare providers to offer customized guidance and interventions," he says.
Unmasking the Silent Threat of CAD
CAD results from a dangerous buildup of plaque in the arteries, leading to reduced blood flow to the heart. Many remain oblivious to this growing threat until they endure a heart attack. It’s crucial to recognize that the seeds of CAD are often sown much earlier in life, highlighting the importance of early intervention.
Data-Driven Precision in Risk Prediction
Torkamani’s team sought to broaden risk factors for CAD beyond mere age, exploring connections with genetics, lifestyles, and medical history. Utilizing a decade’s worth of data from the UK Biobank, they created a machine learning model that identifies CAD risk factors more effectively.
From Thousands to Key Predictors
Starting with around 2,000 potential risk factors, researchers refined their focus to 53 critical indicators, including physical measurements, blood biomarkers, family medical history, mental health, sleep patterns, and specific genetic variations.
A Triumph in Risk Classification
The new model significantly outperformed traditional methods, identifying double the number of CAD events. Impressively, after ten years, about 62.9% of those marked as high risk developed CAD, contrasting starkly with just 0.3% in the low-risk group. According to Chen, this model enhances risk classification for about one in four individuals, pinpointing those truly at risk while alleviating undue anxiety for others.
Spotting Hidden Risks in Low-Risk Groups
Remarkably, this innovative model adeptly identifies individuals—often classified as low-risk due to their age—who actually face high underlying genetic risks for CAD. Torkamani explains, "Even younger patients or women, typically labeled as low risk, can have alarming genetic warnings that require attention."
Genetics: The Predominant Predictor
Through analysis, the strongest risk predictor emerged as genetic predisposition. Conditions such as high blood pressure and cholesterol levels significantly correlate with CAD risk; higher genetic markers mean deeper impacts.
Validation Across Diverse Populations
When validated using the NIH All of Us dataset, which encompasses a more diverse population than the UK Biobank, the model maintained its predictive power across European, African, and Hispanic ancestries.
The Future of CAD Prevention
Looking ahead, the researchers are set to launch a long-term clinical trial assessing whether informing patients about their CAD risks influences their prevention strategies. As Chen puts it, "The key is for patients to understand their personal risks, enabling them to pursue the right treatments and lifestyle adjustments."