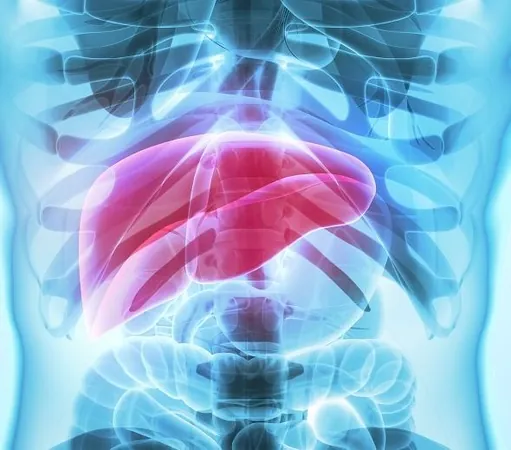
Revolutionary Machine Learning Model Set to Transform HCC Risk Prediction in Chronic HBV Patients!
2024-12-21
Author: Mei
Introduction
A groundbreaking machine learning model is making waves in the medical community by offering a superior method for predicting the risk of hepatocellular carcinoma (HCC) in patients with chronic hepatitis B virus (HBV) following five years of robust antiviral therapy. Dubbed the “Machine learning Algorithm for Prediction of Liver cancer after 5 years of antiviral therapy” (MAPL-5), this innovative tool combines logistic regression and random forest methodologies to analyze 36 detailed clinical variables, aiming to enhance the accuracy of traditional prediction models for HCC risk.
Background
According to the World Health Organization, in 2022, approximately 254 million people worldwide were living with chronic HBV, resulting in an alarming 1.1 million deaths, primarily due to cirrhosis and HCC. While the use of potent nucleos(t)ide analogs, like entecavir (ETV) or tenofovir (TFV), has effectively reduced complications and mortality rates among patients, concerns persist over the long-term risk of HCC as these patients enjoy extended lifespans through successful treatment.
Research Findings
Dr. Han Chu Lee and his research team from the Asan Liver Center at the University of Ulsan College of Medicine, South Korea, emphasized that as the life expectancy of chronic hepatitis B patients increases, so does the incidence of HCC. They highlighted the need for reliable prediction models for patients who remain free of HCC after five years of antiviral therapy, noting that while initial risks may be low, the absolute risk could incrementally rise due to enhanced longevity.
To validate the MAPL-5 model, researchers conducted a multicenter retrospective cohort study, leveraging data from two hospitals for patients who commenced ETV or TFV therapy between January 2009 and December 2015. The study specifically excluded patients diagnosed with HCC or other malignancies in the first five years, those with decompensated liver cirrhosis at the start of therapy, or individuals lacking regular monitoring for HCC development.
The study included 6,470 patients, with a significant discrepancy in age and gender distribution between the derivation and validation cohorts. Throughout an impressive median follow-up duration of 8.6 years, 279 (4.7%) of patients developed HCC in the derivation group versus 25 (4.5%) in the external validation group.
Machine Learning Techniques
Among the various machine learning techniques assessed, AdaBoost and logistic regression achieved the highest balanced accuracy when combined with other algorithms, ultimately refining the final model that reached a balanced accuracy of 0.754 and an area under the curve (AUC) of 0.811. This ensemble model notably outperformed traditional methods, showcasing its potential to revolutionize HCC risk predictions.
Validation and Future Implications
The MAPL-5 model demonstrated strong external validation results, achieving a balanced accuracy of 0.771 and an AUC of 0.862 in an independent cohort. If further global validation and larger patient cohort analyses support its efficacy, this model could redefine clinical practices by aiding healthcare professionals in making informed decisions regarding HCC surveillance and patient education.
Limitations
However, researchers also recognized the limitations of this model, including potential biases relating to ethnicity and HBV genotypes, pinpointing the essential need for more extensive studies to define risk cut-offs accurately.
Conclusion
“The MAPL-5 model can assist practitioners in clinical decision-making, educate patients about their real risks, and guide evidence-based policies for HCC monitoring on a public health level,” concluded the investigators, signaling a promising future in the fight against liver cancer for individuals with chronic HBV.
Stay tuned for updates on this exciting development in the realm of liver health and disease prevention!