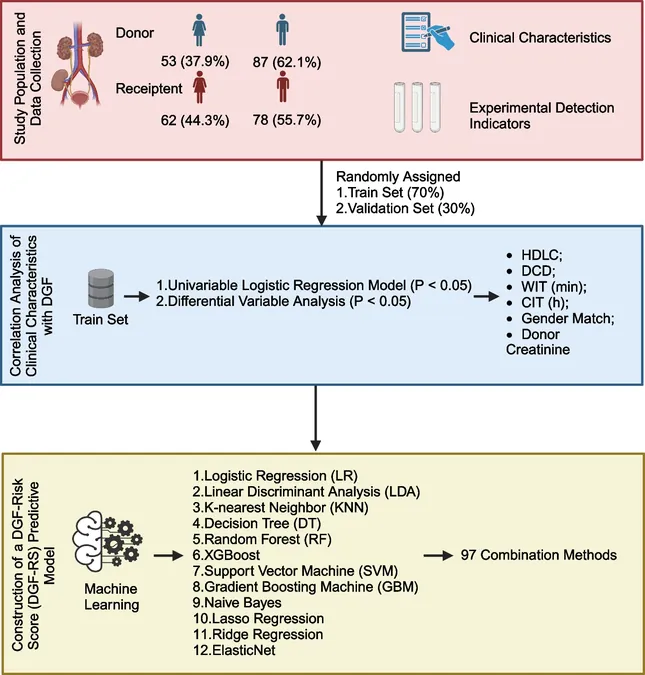
Revolutionary Machine Learning Model Predicts Delayed Graft Function in Pediatric Kidney Transplants from Deceased Donors
2024-09-20
Introduction
Children suffering from end-stage renal disease (ESRD) often find hope in kidney transplantation, which is currently recognized as the superior treatment option. However, the occurrence of Delayed Graft Function (DGF) poses a significant challenge, impacting long-term outcomes for young recipients and potentially compromising the functionality of the graft. Research into DGF in pediatric transplant recipients has been notably limited, underscoring the urgent need for predictive capabilities to guide clinical management and interventions.
This groundbreaking study aims to unveil an integrated machine learning (ML) model that leverages both donor and recipient characteristics to predict the risk of DGF following kidney transplantation from deceased donors. By doing so, the researchers intend to provide essential insights for clinicians, ultimately enhancing pediatric patient care.
Methodology Overview
Conducted as a single-center retrospective cohort study, the research encompasses pediatric patients under 18 who underwent single-donor kidney transplantation between 2016 and 2023. In-depth data collection processes involved demographic, clinical, and laboratory information from both recipients and donors. Utilizing univariate logistic regression analysis, the investigators pinpointed features associated with DGF before constructing a risk score (DGF-RS) based on various machine learning algorithms. The model's performance was rigorously evaluated through receiver operating characteristic curves and decision curve analysis, among other techniques.
Key Findings
Of the 140 pediatric kidney transplant recipients analyzed, 37 (26.4%) developed DGF. Noteworthy predictors identified included high-density lipoprotein cholesterol (HDLC), donor after circulatory death (DCD), warm ischemia time (WIT), cold ischemia time (CIT), donor-recipient gender match, and donor creatinine levels. Among 97 machine learning models assessed, the random forest model emerged as the frontrunner, achieving exceptional area under the curve (AUC) values of 0.983, 1.000, and 0.905 across the entire cohort, training set, and validation set respectively.
Implications for Pediatric Transplantation
The introduction of the DGF-RS model represents a transformative step toward enhancing pediatric kidney transplantation outcomes. By addressing the limited predictive capabilities of traditional indicators, this ML-based approach stands to revolutionize how healthcare professionals identify at-risk patients and implement tailored interventions aimed at mitigating DGF.
Additionally, the study highlighted the interplay between various factors influencing DGF, including the adverse effects of prolonged ischemia, donor age, and emerging biomarkers. The predictive accuracy of the DGF-RS model, significantly exceeding existing methods, indicates its potential utility in clinical decision-making, potentially informing organ allocation, pre-transplant counseling, and post-operative monitoring strategies.
Broader Context and Future Directions
As the machine learning landscape continues to evolve, this study underscores the potential for utilizing advanced computational techniques to analyze complex datasets in medical research. The findings advocate for further investigations into the long-term impacts of DGF and the potential integration of additional biomarkers and pre/post-transplantation factors in predictive models.
Ultimately, this research not only contributes invaluable insights into the prediction of DGF but also paves the way for future developments that could profoundly enhance the quality of life for pediatric kidney transplant recipients. In a world where personalized medicine is becoming increasingly vital, the DGF-RS model holds promise for refining treatment strategies and fostering improved patient outcomes in pediatric nephrology.
With the innovative combination of machine learning and clinical data, this study exemplifies how advanced technology can bridge gaps in healthcare, ensuring that even the youngest patients receive the best possible care in their critical journeys toward recovery.