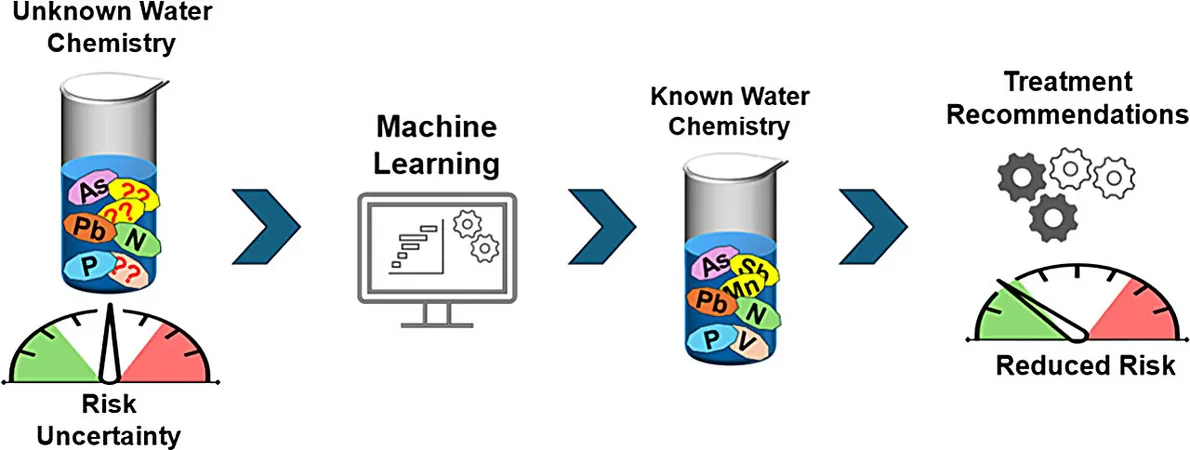
Revolutionary Machine Learning Framework Discovers Groundwater Contamination Hotspots—What You Need to Know!
2024-11-12
Author: Li
Groundbreaking Machine Learning Development
In a groundbreaking development, a team of interdisciplinary researchers has harnessed the power of machine learning to create a cutting-edge framework designed to predict the presence of inorganic pollutants in groundwater supplies. This innovative tool leverages limited water quality samples, allowing regulatory bodies and public health officials to focus their testing efforts on the most at-risk aquifers.
Wider Implications of the Research
While the initial research concentrated on groundwater quality in Arizona and North Carolina, the potential applications of this technology could fill significant voids in water quality data across various regions in the United States and beyond. The study is detailed in the open-access paper titled "Multiple Data Imputation Methods Advance Risk Analysis and Treatability of Co-occurring Inorganic Chemicals in Groundwater," published in the prestigious journal Environmental Science & Technology.
Importance of Groundwater Quality
Groundwater serves as a crucial source of drinking water for millions. Unfortunately, it often harbors contaminants that pose serious health risks. Alarmingly, many regions are plagued by incomplete groundwater quality datasets, which hampers effective monitoring and response.
Expert Insights on Monitoring Challenges
“Monitoring water quality can be a daunting, time-consuming, and costly process,” explains Yaroslava Yingling, a co-corresponding author and Kobe Steel Distinguished Professor of Materials Science and Engineering at North Carolina State University. “Given these constraints, identifying which groundwater supplies warrant priority testing becomes vital in optimizing our limited monitoring resources.”
Predicting Pollutants in Groundwater
The researchers focus on the likelihood of naturally occurring pollutants—such as arsenic and lead—co-occurring due to various geological and environmental factors. This pivotal study poses a significant question: with limited data, is it possible to predict the presence and levels of other harmful pollutants?
“Our aim was not just to identify harmful elements but also to forecast the presence of beneficial elements like phosphorus, which can have contrasting implications for agriculture and the environment,” states Alexey Gulyuk, a co-first author and teaching professor of materials science and engineering at NC State.
The Dataset Behind the Model
To tackle this issue, the team utilized an extensive dataset encompassing over 140 years of water quality monitoring data specifically for North Carolina and Arizona. This wealth of information includes more than 20 million data points across 50 distinct water quality parameters, serving as the backbone for training their machine learning model.
Functionality of the Machine Learning Model
“This model allows us to make educated guesses about which pollutants might be present, even when only a few water quality parameters are known,” explains Akhlak Ul Mahmood, another co-first author and former Ph.D. student at NC State. "So, if we have sparse data, our framework can still predict the presence of inorganic pollutants and their probable concentrations."
Revelations on Groundwater Safety Standards
Strikingly, the study highlights that the machine learning model indicates groundwater pollutants may be exceeding safe drinking standards in far more locations than previously recognized. While field data suggested that 75%-80% of sampled locations fell within acceptable limits, this new analysis predicts that only 15%-55% of those sites are genuinely risk-free.
Impacts on Water Safety Assessments
“This discovery reveals numerous groundwater sites that warrant immediate attention for further testing,” notes Minhazul Islam, co-first author and a Ph.D. student at Arizona State University. “By pinpointing these potential 'hot spots,' state agencies can effectively allocate their resources to areas of highest concern, ensuring targeted testing and effective remediation strategies.”
Future Plans for Model Enhancement
The researchers are excited about the model's potential efficacy but acknowledge their real-world testing will determine its accuracy.
As they look to the future, the team aims to enhance their model by incorporating diverse datasets across different U.S. regions, integrating new environmental data layers to recognize emerging contaminants, and conducting practical tests to ensure robust groundwater safety measures globally.
Broader Applications for Sustainable Management
“We see immense possibilities with this approach,” asserts Paul Westerhoff, co-corresponding author and Regents' Professor at ASU. “By consistently refining its accuracy and application, we are establishing a proactive framework for global water safety initiatives.”
Monitoring Phosphorus Levels
In an additional layer of complexity, Jacob Jones, director of the Science and Technologies for Phosphorus Sustainability (STEPS) Center at NC State, emphasizes that this model could also serve as a vital tool for monitoring phosphorus levels in groundwater. He highlights that managing this essential nutrient could significantly impact various ecosystems and agricultural practices, promoting a more sustainable future.
Conclusion: A New Era for Groundwater Monitoring
In summary, the introduction of this innovative machine learning framework heralds a significant leap forward in groundwater health monitoring and management. This unprecedented technology may very well lead to transformative water safety measures, paving the way for cleaner and safer drinking water for all. Don’t miss out on the developments—stay informed about this critical issue to ensure the water you drink is both safe and sustainable!