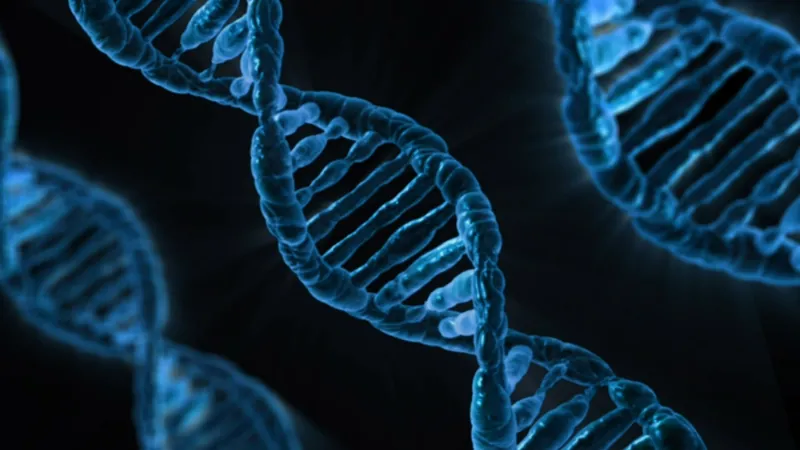
Revolutionary Gene Study Method May Lead to Tailored Treatments for Genetic Disorders!
2024-11-07
Author: Wei Ling
Introduction
In an exciting breakthrough for genomics, researchers from MIT have developed a groundbreaking strategy to understand the intricate web of interactions among the approximately 20,000 genes in the human genome. This innovative causal theory aims to unravel the complex cause-and-effect relationships between genes, paving the way for targeted treatments that could transform how we approach many diseases.
Understanding Gene Interactions
By investigating changes in gene expression, scientists can gain invaluable insights into cellular functions, which is critical for understanding the onset and progression of various health conditions. However, the challenge lies in the fact that genes interact in highly complex ways, often forming modules that influence each other’s regulation. Identifying which gene groups to target for effective treatment has long been a daunting task.
MIT's Innovative Approach
The MIT team has made significant strides in this arena by creating theoretical foundations that allow for the efficient grouping of genes based on their relationships. Their research, now available on the arXiv preprint server, utilizes only observational data—meaning that it could eliminate the need for costly and sometimes impractical interventional experiments.
Key Insights from Researchers
Graduate student Jiaqi Zhang, one of the co-lead authors of the study, emphasizes the importance of understanding the mechanisms underlying various cell states. "If you figure out the right way to aggregate the observed data, the information you learn about the system should be more interpretable and useful," Zhang stated confidently.
These findings were further supported by fellow co-lead author Ryan Welch, and senior author Caroline Uhler, who heads the Eric and Wendy Schmidt Center at the Broad Institute of MIT and Harvard. Uhler remarked on the team's novel causal theory, which lays the groundwork for future investigations into genetic data, including how to pinpoint gene modules and their regulatory interactions.
Significance of the Research
What makes this research particularly significant is its ability to derive meaningful insights from observational data alone. Traditionally, causal disentanglement has required interventional studies—which can be both costly and controversial. With this new method, MIT researchers are taking giant leaps forward in genetic research.
Causal Disentanglement Explained
The innovative approach involves a process called causal disentanglement, where genes are combined into groups to better understand their collective influence on biological processes like cell differentiation and development. By leveraging advanced machine-learning algorithms, the team can identify causal modules and reconstruct an accurate representation of underlying mechanisms—shedding light on how sets of genes interact without needing experimental manipulation.
Developing a Novel Causal Theory
"We had to develop a novel causal theory to understand what could and could not be learned from observational data," Uhler explained. "Armed with this knowledge, we can apply our findings to genetic data to uncover vital gene interactions often obscured in traditional studies."
Algorithmic Efficiency
In a significant achievement, the researchers devised an efficient algorithm to manage the complexity of these interactions, determining which gene groups are interconnected and how they regulate one another. Their simulation results confirm the algorithm's effectiveness, demonstrating its potential to transform our understanding of genetic interactions.
Future Applications
Looking ahead, the researchers intend to apply their approach to real-world genetics research, with the hope of identifying specific gene programs linked to diseases. This could lead scientists to more targeted and effective drug therapies aimed at firing on the right genetic pathways for treatment.
Conclusion
In a medical landscape that increasingly emphasizes personalized medicine, this methodology could be the key to unlocking new avenues for combating genetic disorders and ultimately improving patient outcomes. Keep an eye on this innovative research as it unfolds—it may very well shape the future of medical treatments!