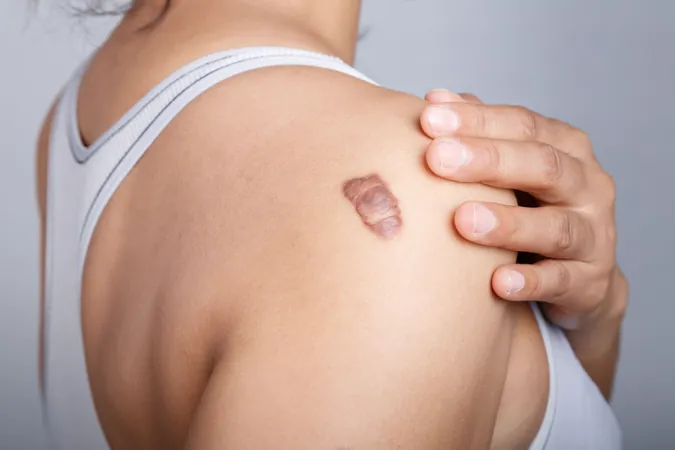
Revolutionary Deep Learning Tool Accurately Distinguishes Keloids from Malignant Skin Lesions Without Dermoscopy!
2025-04-02
Author: Mei
A groundbreaking study has revealed that a deep learning model can effectively differentiate keloids from both benign and malignant skin lesions using only non-dermatoscopic clinical images. This promising research, published in the journal Diagnostics, showcases the immense potential of advanced technology in enhancing medical diagnostics.
The study employed the VGG16 convolutional neural network (CNN), which demonstrated impressive performance metrics: an area under the receiver operating characteristic curve of 0.996, a perfect precision score of 1.0, and an accuracy rate of 0.985. The remarkable results indicate that the VGG16 model could serve as an invaluable tool in medical settings lacking immediate access to dermatology specialists or dermatoscopic imaging capabilities.
Keloids—raised scars that can develop after skin injuries—are often mistakenly identified as malignant lesions due to their similar characteristics: aggressive growth patterns, lack of spontaneous regression, and a high rate of recurrence. While these thickened scars result from excessive collagen production and do not spread like cancerous tumors, misdiagnosis can lead to ineffective treatment strategies, highlighting the critical role of accurate diagnostics.
The study's findings were particularly encouraging as VGG16 outperformed eight other pretrained CNNs in two of three essential classification tasks: accurately categorizing seven types of lesions as either malignant or benign and distinctly identifying keloids as a separate category. Interestingly, the DenseNet121 model excelled in the challenging task of telling keloids apart from their malignant counterparts.
To achieve these results, researchers retrained the CNNs using publicly available datasets that included 2,298 images of common skin cancers and 274 images of keloids. Validation against new test data demonstrated consistent performance, illustrating the model's practicality in environments with limited access to advanced imaging technologies.
However, challenges remain. The VGG16 model misclassified one test image due to poor image quality, underscoring the importance of clear and well-framed images for reliable diagnoses. The researchers noted, “This could be attributed to a lack of similar data from specific anatomical regions used during training. We believe that more diverse image datasets will enhance model performance. Moreover, integrating clinical features of each skin lesion with their corresponding images has been shown to enhance CNN performance, a strategy we are excited to explore.”
The authors also highlighted that the training sets were relatively limited and might not adequately represent all anatomical regions. Additionally, some benign lesion images lacked pathological confirmation, which could affect the overall reliability of the model.
This innovative approach to skin lesion classification has the potential to revolutionize dermatology, making accurate diagnostics more accessible, especially in remote locations. As research continues, the integration of artificial intelligence in healthcare promises to bridge the gap in medical expertise, ultimately leading to better patient outcomes. Stay tuned for more updates on this remarkable development!