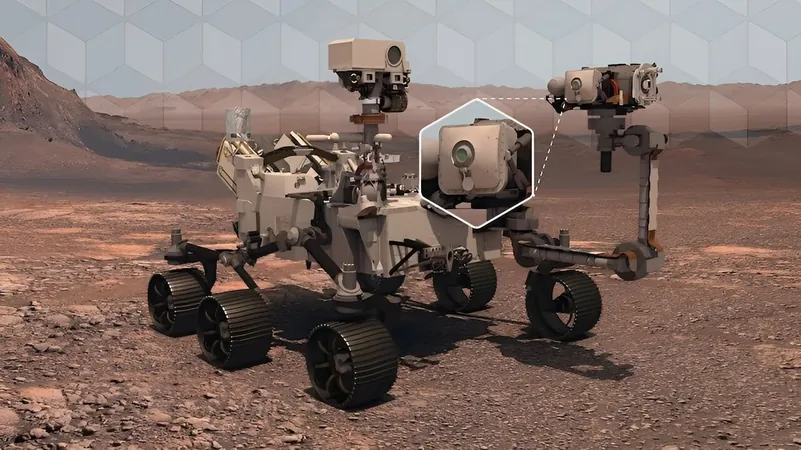
Revolutionary Algorithm from Mars Rover Enhances Earthly Weather Predictions
2024-09-20
Introduction
A groundbreaking algorithm developed and tested on NASA's Perseverance Rover could significantly improve how scientists forecast extreme weather events like hurricanes and wildfires—phenomena that threaten millions of lives across the globe. This innovative technology, known as Nested Fusion, has the potential to reshape our understanding and management of critical environmental issues on Earth.
The Genius Behind Nested Fusion
The genius behind this development is Austin P. Wright, a Ph.D. student at Georgia Tech, whose research focuses on machine learning. His recent publication introduced Nested Fusion, providing an essential tool for scientists to analyze vast datasets, particularly in their quest for signs of ancient life on Mars. This algorithm not only supports NASA's Mars 2020 mission but also offers versatility for various scientific disciplines dealing with complex data.
Presentation and Recognition
Wright unveiled Nested Fusion at the 2024 International Conference on Knowledge Discovery and Data Mining (KDD 2024), where it impressively secured the runner-up spot for the best paper award. The findings are published in the esteemed journal, *Proceedings of the 30th ACM SIGKDD Conference on Knowledge Discovery and Data Mining*.
Benefits Across Research Sectors
Wright emphasizes the auxiliary benefits of Nested Fusion across diverse research sectors: "This method is instrumental not only for NASA scientists but for researchers in fields like chemistry, biology, and geology. It facilitates a clearer visualization of intricate datasets that can overwhelm initial analyses."
Functionality of Nested Fusion
The Nested Fusion algorithm is designed to merge datasets of varying resolutions, culminating in a singular, high-resolution output that streamlines data interpretation. This feature empowers Mars researchers to analyze multi-source datasets more effectively, propelling faster investigations into Mars' surface to uncover traces of historical life.
Applications in Climate and Environmental Science
Additionally, Wright is actively exploring applications of Nested Fusion in climate science, biology, and environmental studies. Its adaptable nature allows the amalgamation of overlapping data types—ranging from satellite images to climate statistics—allowing scientists to draw critical insights about shifting ecosystems and climate trends.
Efficiency and Impact on Research
Promptly addressing the often convoluted process of cross-correlational analysis, Nested Fusion not only expedites data processing but also enhances early-stage pattern recognition. Before this algorithm's introduction, formulating even an initial mineral composition prediction could take days. However, with Nested Fusion, a single scientist can accomplish this task in just hours.
Role in PIXLISE
Wright serves as the data science and machine learning lead for PIXLISE, a software toolkit employed by NASA's Jet Propulsion Laboratory (JPL) to analyze Mars data collected by the Perseverance Rover. This rover utilizes the Planetary Instrument for X-ray Lithochemistry (PIXL), which consists of the X-ray Fluorescence (XRF) Spectrometer and the Multi-Context Camera (MCC) to investigate Martian mineral composition.
Challenges and Solutions
When conducting analyses, PIXL produces co-aligned datasets from these two instruments. While XRF gathers fine-scale elemental data, MCC provides essential visual details of the sampled areas. The dissimilar resolutions of these datasets historically complicated the process of mapping combined data layers—yet Wright and his collaborators have expertly harnessed Nested Fusion to eliminate these issues.
Advancing Scientific Progress
In addition to advancing scientific progress, Nested Fusion significantly enhances efficiency for NASA researchers, allowing for quicker assessments while freeing experts to focus on broader research questions.
Collaboration and Real-World Applications
"Working closely with collaborators has taught me the importance of anchoring my machine learning and data science work in practical applications," Wright explains. "Understanding and addressing their specific challenges paves the way to innovative solutions in data science."
Conclusion
In conclusion, Nested Fusion has emerged as a seminal development in both planetary sciences and earth sciences, spotlighting how advancements in technology can lead to impactful changes in our understanding of complex data landscapes. At the conference, many esteemed research papers were presented, making Wright's recognition even more remarkable. His mentors, Scott Davidoff of NASA JPL and Polo Chau from Georgia Tech, played pivotal roles in this achievement, lending their expertise to the project.
As Wright celebrates this honor, he notes, "This recognition reaffirms the value of applying our work to real-world problems, encouraging us to continue our pursuit of knowledge at the intersection of technology and science."