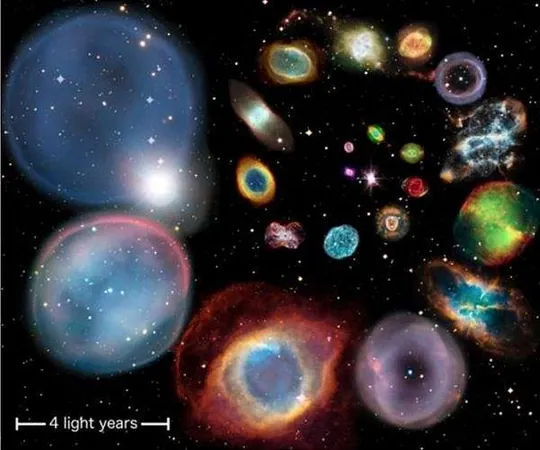
Revolutionary AI Technologies Transform Stellar Classification with Unprecedented Accuracy
2025-03-25
Author: Yu
In an exciting development for astronomers around the globe, artificial intelligence (AI) is taking center stage in the realm of stellar classification, significantly enhancing the precision with which stars are categorized.
A groundbreaking research collaboration recently revealed how advanced deep learning algorithms and large language models have been employed to accurately and efficiently classify stars based on their light curves.
Published on February 26, 2025, in the journal *Intelligent Computing*, the study, titled "Deep Learning and Methods Based on Large Language Models Applied to Stellar Light Curve Classification," showcases these state-of-the-art techniques.
At the heart of the research is the innovative StarWhisper LightCurve series, comprising three AI-powered models specifically designed to process and classify variable stars using light curve data.
These models harness automated deep learning techniques to independently adjust critical training parameters—such as learning rate, batch size, and model complexity—minimizing the need for manual oversight and allowing for streamlined operations.
The training data for these models was sourced from NASA’s Kepler and K2 missions, including information on five predominant categories of variable stars and a smaller selection of rare types, which provides the models with a broader range of reference points for classification.
When evaluated for performance, the AI models exhibited remarkable accuracy in distinguishing between various kinds of variable stars.
Notably, the Conv1D + BiLSTM model, which integrates convolutional neural networks with bidirectional long short-term memory layers, achieved an impressive accuracy rate of 94%.
Even more striking, the Swin Transformer—an advanced model originally designed for natural language processing—achieved a staggering 99% accuracy rate.
This included a highlight where it successfully identified Type II Cepheid stars, a rare pulsating star type representing just 0.02% of the dataset, with an impressive accuracy of 83%.
While the Swin Transformer boasts superior accuracy, it does require extensive preprocessing, including converting light curves into image formats.
In contrast, the StarWhisper LightCurve models approached close to 90% accuracy with minimal human intervention, thus greatly enhancing operational efficiency.
This efficiency is paving the way for scalable and automated analysis methods in astronomical research, which are essential for handling the growing amounts of astronomical data being collected.
The StarWhisper LightCurve suite features three large language models specifically tailored for different types of astronomical data analysis: 1. A text-based model utilizing Gemini 7B, optimized specifically for time-series data classification. 2. A multimodal model based on DeepSeek-VL-7B-Chat, crafted for analyzing image-rendered light curves. 3. An audio-based model, engineered using Qwen-Audio, which translates light curves into sound wave data for classification applications.
These models are part of a broader initiative aimed at enhancing astronomical research through the development of AI technologies that possess advanced reasoning and instruction-following capabilities.
As our understanding of the universe expands, these AI solutions provide astronomers with powerful tools to decode the mysteries of the cosmos with unprecedented accuracy.
Stay tuned for more revolutionary discoveries in astrophysics as researchers continue to explore the potential of AI in celestial studies!